Why Do AI Chatbots Hallucinate? Exploring the Science
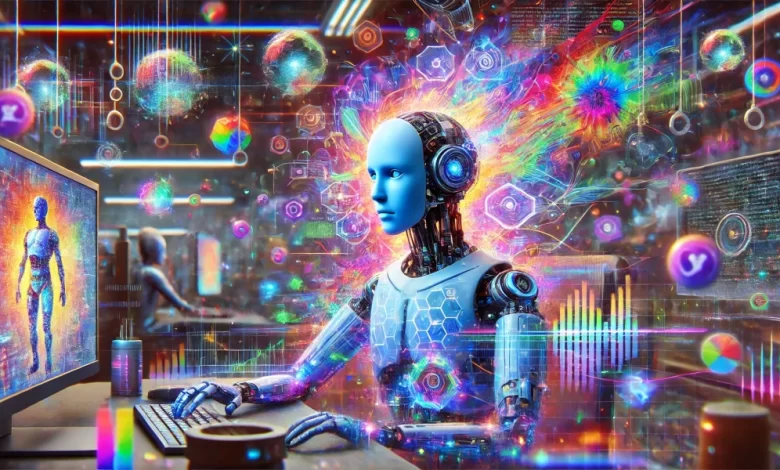
Artificial intelligence (AI) chatbots have become an integral part of our lives today, helping with everything from managing schedules to providing customer support. However, as these chatbots become more sophisticated, the worrying problem known as hallucination has emerged. In AI, hallucination refers to instances in which a chatbot generates inaccurate, misleading, or completely fabricated information.
Imagine asking your virtual assistant about the weather, and it gives you outdated or completely wrong information about a storm that never happened. While this may be interesting, such hallucinations can lead to serious consequences in critical areas such as healthcare or legal advice. Therefore, understanding why AI chatbots hallucinate is essential to increase their reliability and security.
The basics of AI chatbots
AI chatbots are powered by advanced algorithms that allow them to understand and generate human language. There are two main types of AI chatbots: rules-based and generative models.
Rules-based chatbots follow predefined rules or scripts. They can perform simple tasks such as reserving a table at a restaurant or answering frequently asked customer service questions. These bots operate within a limited range and rely on specific triggers or keywords to provide accurate answers. However, their rigidity limits their ability to handle more complex or unexpected questions.
Generative models, on the other hand, use machine learning and Natural Language Processing (NLP) to generate answers. These models are trained on large amounts of data, learning patterns and structures in human language. Popular examples include OpenAI’s GPT series and Google’s BERT. These models can create more flexible and contextually relevant responses, making them more versatile and adaptable than rules-based chatbots. However, this flexibility also makes them more susceptible to hallucinations, as they rely on probabilistic methods to generate responses.
What is AI Hallucination?
AI hallucination occurs when a chatbot generates content that is not based in reality. This could be as simple as a factual error, such as misrepresenting the date of a historical event, or something more complex, such as making up an entire story or a medical recommendation. While human hallucinations are sensory experiences without external stimuli, often caused by psychological or neurological factors, AI hallucinations arise from the model’s misinterpretation or overgeneralization of the training data. For example, if an AI has read a lot of texts about dinosaurs, it can incorrectly generate a new, fictional dinosaur species that never existed.
The concept of AI hallucination has been around since the early days of machine learning. Initial models, which were relatively simple, often made seriously questionable errors, such as suggesting that “Paris is the capital of Italy.” As AI technology advanced, the hallucinations became more subtle but potentially more dangerous.
Initially, these AI errors were seen as mere anomalies or curiosities. However, as AI’s role in critical decision-making processes has grown, addressing these issues has become increasingly urgent. The integration of AI into sensitive areas such as healthcare, legal advice and customer service increases the risks associated with hallucinations. This makes it essential to understand and mitigate these events to ensure the reliability and security of AI systems.
Causes of AI hallucination
To understand why AI chatbots hallucinate, several interconnected factors need to be examined:
Data quality issues
The quality of the training data is critical. AI models learn from the data they receive, so if the training data is biased, outdated, or inaccurate, the AI’s results will reflect these shortcomings. For example, if an AI chatbot is trained on medical texts that contain outdated practices, it may recommend outdated or harmful treatments. Furthermore, if the data is not diverse, the AI may not understand the context outside its limited training range, which can lead to erroneous results.
Model architecture and training
The architecture and training process of an AI model also play a crucial role. Overfitting happens when an AI model learns the training data too well, including the noise and errors, causing it to perform poorly on new data. Conversely, underfitting occurs when the model needs to adequately learn the training data, resulting in oversimplified responses. Therefore, maintaining a balance between these extremes is challenging, but essential for reducing hallucinations.
Ambiguities in language
Human language is by nature complex and full of nuances. Words and phrases can have multiple meanings depending on the context. For example the word “Bank‘ could mean a financial institution or the bank of a river. AI models often require more context to disambiguate such terms, leading to misunderstandings and hallucinations.
Algorithmic challenges
Current AI algorithms have limitations, especially in dealing with long-term dependencies and maintaining consistency in their responses. These challenges can cause the AI to make contradictory or unlikely statements even within the same conversation. For example, an AI might claim one fact at the beginning of a conversation and then contradict itself later.
Recent developments and research
Researchers are constantly working to reduce AI hallucinations, and recent studies have shown promising progress in several important areas. An important effort is to improve data quality by compiling more accurate, diverse and up-to-date data sets. This includes developing methods to filter out biased or incorrect data and ensure that the training sets represent different contexts and cultures. By refining the data on which AI models are trained, the likelihood of hallucinations decreases as the AI systems gain a better foundation of accurate information.
Advanced training techniques also play a crucial role in tackling AI hallucinations. Techniques such as cross-validation and more extensive data sets help reduce problems such as overfitting and underfitting. In addition, researchers are exploring ways to incorporate better contextual understanding into AI models. Transformer models, such as BERT, have shown significant improvements in understanding and generating contextually appropriate responses, reducing hallucinations by allowing the AI to understand nuances more effectively.
In addition, algorithmic innovations are being explored to directly address hallucinations. One of those innovations is Exploreable AI (XAI), which aims to make AI decision-making processes more transparent. By understanding how an AI system reaches a certain conclusion, developers can more effectively identify and correct the sources of hallucinations. This transparency helps identify and mitigate the factors that lead to hallucinations, making AI systems more trustworthy and reliable.
These combined efforts in data quality, model training, and algorithmic advancements represent a multifaceted approach to reducing AI hallucinations and improving the overall performance and reliability of AI chatbots.
Real-world examples of AI hallucination
Real-world examples of AI hallucination show how these errors can impact different sectors, sometimes with serious consequences.
In health care, a study from the University of Florida College of Medicine tested ChatGPT on common urology-related medical questions. The results were worrying. The chatbot only provided appropriate answers 60% of the time. It often misinterpreted clinical guidelines, omitted important contextual information, and made incorrect treatment recommendations. For example, it sometimes recommends recognizing treatments without critical symptoms, which could lead to potentially dangerous advice. This shows the importance of ensuring that medical AI systems are accurate and reliable.
There have been significant incidents in customer service where AI chatbots provided incorrect information. A remarkable case concerns Air Canada’s chatbot, who provided inaccurate details about their policy on funeral rates. This misinformation led to a traveler missing out on a refund, causing significant disruption. The court ruled against Air Canada and emphasized their responsibility for the information provided by their chatbot. This incident highlights the importance of regularly updating and verifying the accuracy of chatbot databases to avoid similar issues.
The legal industry has experienced significant problems with AI hallucinations. In a lawsuit New York attorney Steven Schwartz used ChatGPT to generate legal references for a brief, which contained six fabricated case citations. This led to serious consequences and highlighted the need for human oversight of AI-generated legal advice to ensure accuracy and reliability.
Ethical and practical implications
The ethical implications of AI hallucinations are profound, as AI-driven disinformation can lead to significant harm, such as medical misdiagnoses and financial losses. Ensuring transparency and accountability in AI development is critical to mitigating these risks.
Misinformation from AI can have real-world consequences, putting lives at risk through incorrect medical advice and resulting in unjust outcomes through flawed legal advice. Regulators such as the European Union have begun to address these issues with proposals such as the AI Act, which aims to establish guidelines for the safe and ethical use of AI.
Transparency in AI operations is essential, and XAI’s field focuses on making AI decision-making processes understandable. This transparency helps identify and correct hallucinations, making AI systems more trustworthy and reliable.
It comes down to
AI chatbots have become essential tools in several areas, but their propensity for hallucinations poses significant challenges. By understanding the causes, ranging from data quality issues to algorithmic limitations, and implementing strategies to mitigate these errors, we can improve the reliability and security of AI systems. Continued advances in data curation, model training, and explainable AI, combined with essential human oversight, will ensure that AI chatbots provide accurate and reliable information, ultimately increasing the trust and usability of these powerful technologies.
Readers should also learn about the best AI hallucination detection solutions.