Western Bias in AI: Why Global Perspectives Are Missing
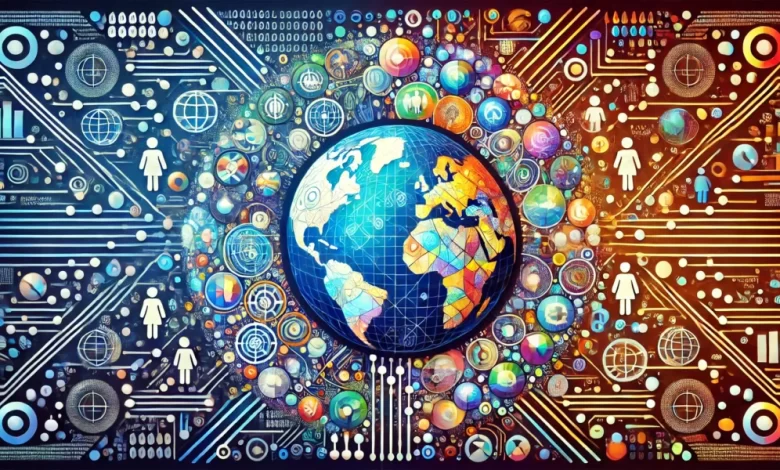
An AI assistant gives an irrelevant or confusing answer to a simple question, revealing an important problem while struggling to understand cultural nuances or language patterns outside of its training. This scenario is typical of billions of people who rely on AI for essential services such as healthcare, education or job support. For many, these resources fall short, often causing their needs to be misrepresented or completely excluded.
AI systems are primarily driven by Western languages, cultures and perspectives, creating a limited and incomplete representation of the world. These systems, built on biased data sets and algorithms, do not reflect the diversity of the world’s population. The impact goes beyond technical limitations, reinforcing social inequality and deepening divisions. Addressing this imbalance is essential to realizing and harnessing AI’s potential to serve all of humanity, not just a privileged few.
Understanding the roots of AI bias
AI bias is not simply an error or oversight. It comes from the way AI systems are designed and developed. Historically, AI research and innovation have been concentrated in Western countries. This concentration has resulted in the dominance of English as the primary language for academic publications, data sets, and technological frameworks. As a result, the fundamental design of AI systems often does not take into account the diversity of global cultures and languages, leaving large regions underrepresented.
Biases in AI can generally be divided into algorithmic biases and data-driven biases. Algorithmic bias occurs when the logic and rules within an AI model favor specific outcomes or populations. For example, hiring algorithms trained on historical employment data may unintentionally favor specific demographic groups, reinforcing systemic discrimination.
Data-driven bias, on the other hand, stems from using data sets that reflect existing social inequalities. For example, facial recognition technology often performs better on people with lighter skin tones because the training datasets consist mainly of images from Western regions.
A 2023 report from the AI Now Institute highlighted the concentration of AI development and power in Western countries, especially the United States and Europe, where large technology companies dominate the field. In the same way the Stanford University’s 2023 AI Index Report highlights the significant contributions of these regions to global AI research and development, which reflect a clear Western dominance in data sets and innovation.
This structural imbalance requires that AI systems urgently adopt a more inclusive approach that represents the diverse perspectives and realities of the world’s population.
The global impact of cultural and geographical differences in AI
The dominance of Western-centric data sets has created significant cultural and geographic biases in AI systems, limiting their effectiveness for diverse populations. For example, virtual assistants can easily recognize idiomatic expressions or references common in Western societies, but often fail to respond accurately to users from other cultural backgrounds. A question about a local tradition may receive a vague or incorrect answer, reflecting the system’s lack of cultural awareness.
These biases extend beyond cultural misrepresentations and are exacerbated by geographic differences. Most AI training data comes from urban, well-connected regions in North America and Europe and does not sufficiently include rural areas and developing countries. This has serious consequences in vital sectors.
Agricultural AI tools designed to predict crop yields or detect pests often fail in regions such as Sub-Saharan Africa or Southeast Asia because these systems are not adapted to the unique environmental conditions and agricultural practices of these areas. Similarly, healthcare AI systems, which are typically trained on data from Western hospitals, struggle to make accurate diagnoses for populations in other parts of the world. Research has shown that dermatology AI models trained primarily on lighter skin tones perform significantly worse when tested on different skin types. For example, a 2021 study found that AI models for skin disease detection experienced a 29-40% drop in accuracy when applied to datasets containing darker skin tones. These issues transcend technical limitations and reflect the urgent need for more inclusive data to save lives and improve global health outcomes.
The social consequences of this bias are far-reaching. AI systems designed to empower individuals often create barriers instead. Education platforms powered by AI tend to prioritize Western curricula, leaving students in other regions without access to relevant or localized resources. Language tools often fail to capture the complexity of local dialects and cultural expressions, rendering them ineffective for large parts of the world’s population.
Bias in AI can reinforce harmful assumptions and increase systemic inequities. For example, facial recognition technology has been criticized for higher error rates among ethnic minorities, leading to serious real-world consequences. In 2020, Robert Williams, a black manwas wrongly arrested in Detroit due to a flawed facial recognition match, highlighting the societal impact of such technological biases.
Economically, neglecting global diversity in AI development could limit innovation and reduce market opportunities. Companies that don’t take diverse perspectives into account risk alienating large segments of potential users. A 2023 McKinsey report estimates that generative AI could contribute between $2.6 trillion and $4.4 trillion annually to the global economy. However, realizing this potential depends on creating inclusive AI systems that target diverse populations around the world.
By addressing biases and expanding representation in AI development, companies can explore new markets, drive innovation, and ensure that the benefits of AI are equitably distributed across all regions. This highlights the economic imperative to build AI systems that effectively reflect and serve the world’s population.
Language as a barrier to inclusivity
Languages are closely linked to culture, identity and community, but AI systems often do not reflect this diversity. Most AI tools, including virtual assistants and chatbots, perform well in a few commonly spoken languages and overlook the less represented. This imbalance means that indigenous languages, regional dialects and minority languages are rarely supported, further marginalizing the communities that speak these languages.
While tools like Google Translate have transformed communication, they still struggle with many languages, especially those with complex grammar or limited digital presence. This exclusion means that millions of AI-powered tools remain inaccessible or ineffective, widening the digital divide. A UNESCO report 2023 revealed that more than 40% of the world’s languages are at risk of disappearing, and their absence in AI systems amplifies this loss.
AI systems reinforce Western dominance in technology by prioritizing only a small part of the world’s linguistic diversity. Addressing this gap is essential to ensure that AI becomes truly inclusive and serves communities around the world, regardless of the language they speak.
Tackling Western biases in AI
Resolving Western biases in AI will require significant change in the way AI systems are designed and trained. The first step is to create more diverse data sets. AI needs multilingual, multicultural, and regionally representative data to serve people around the world. Projects like Masakhanethat supports African languages, and AI4Bharatthat focuses on Indian languages are great examples of how inclusive AI development can succeed.
Technology can also help solve the problem. Federated learning enables data collection and training from underrepresented regions without compromising privacy. Explainable AI tools make detecting and correcting biases easier in real time. However, technology alone is not enough. Governments, private organizations and researchers must work together to fill the gaps.
Laws and policies also play an important role. Governments must enforce regulations that require diverse data for AI training. They must hold companies accountable for distorted outcomes. At the same time, advocacy groups can raise awareness and push for change. These actions ensure that AI systems represent the diversity of the world and serve everyone fairly.
Furthermore, collaboration is just as important as technology and regulations. Developers and researchers from underserved regions must be part of the AI creation process. Their insights ensure AI tools are culturally relevant and practical for different communities. Technology companies also have a responsibility to invest in these regions. This means funding local research, hiring diverse teams and creating partnerships that focus on inclusion.
The bottom line
AI has the potential to transform lives, bridge gaps and create opportunities, but only if it works for everyone. When AI systems overlook the rich diversity of cultures, languages and perspectives worldwide, they fail to deliver on their promise. The issue of Western bias in AI is not just a technical error, but an issue that requires urgent attention. By prioritizing inclusivity in design, data, and development, AI can become a tool that uplifts all communities, not just a privileged few.