Transformers and Beyond: Rethinking AI Architectures for Specialized Tasks
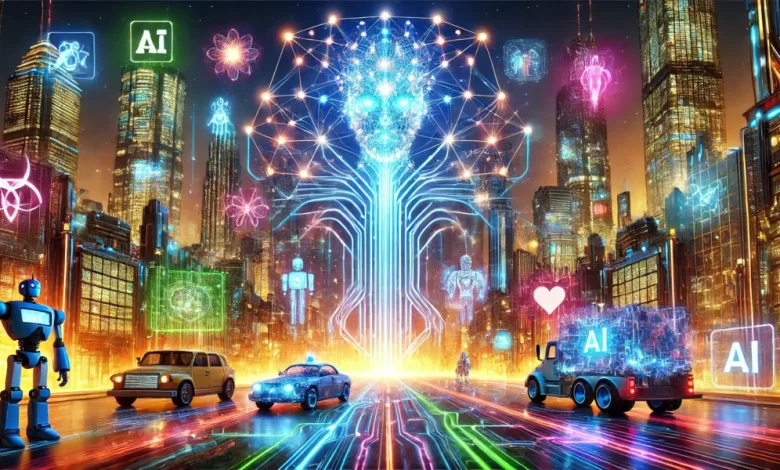
In 2017, an important change of artificial intelligence (AI) reformed. A paper entitled Attention is all you need Introduced transformers. Initially developed to improve the language translation, these models have evolved into a robust framework that excels in sequence modeling, which makes unprecedented efficiency and versatility in various applications possible. Nowadays, transformers are not just a tool for the processing of natural language; They are the reason for a lot of progress in areas that are so diverse as biology, health care, robotics and finances.
What started as a method to improve how machines understand and generate human language, has now become a catalyst for solving complex problems that have existed for decades. The adaptability of transformers is remarkable; their self -confidence With the architecture they can process data and learn in ways that traditional models cannot. This possibility has led to innovations that have completely transformed the AI domain.
Initially, transformers excelled in language tasks such as translation, summary and question answers. Models such as Bert And GPT Nam languages insight into new depths by grabbing the context of words more effectively. For example, chatgpt revolution brought about in conversational AI, transforming customer service and making content.
As these models progressed, they indicated more complex challenges, including multi-turn conversations and understanding less common languages. The development of models such as GPT-4, which integrates both text and image processing, shows the growing possibilities of transformers. This evolution has broadened their application and enabled them to perform specialized tasks and innovations in various industries.
With industries that are increasingly using transformation models, these models are now used for more specific purposes. This trend improves efficiency and deals with issues such as bias and fairness and emphasizes the sustainable use of these technologies. The future of AI with Transformers is about refining their capacities and applying this.
Transformers in various applications outside NLP
The adaptability of transformers has expanded their use much further than the processing of the natural language. Vision transformers (VITs) have a considerably advanced computer vision by using attention mechanisms instead of traditional convention layers. Thanks to this change, VITs can perform better than conventional neural networks (CNNs) in image classification and object detection tasks. They are now being used in areas such as autonomous vehicles, face recognition systems and augmented reality.
Transformers have also found critical applications in health care. They improve diagnostic imaging by improving the detection of diseases in X -rays and MRIs. An important achievement is AlfafoldA transformer-based model developed by DeepMind, which solved the complex problem of predicting protein structures. This breakthrough has accelerated the discovery of medicines and bioinformatics, helping vaccine development and lead to personalized treatments, including cancer therapies.
In robotics, transformers improve decision -making and movement planning. The Tesla AI team uses transformer models in their self-driving systems to analyze complex driving situations in real time. In Finance, transformers help detect fraud and market forecast by processing large datasets quickly. Moreover, they are used in autonomous drones for agriculture and logistics, which shows their effectiveness in dynamic and real -time scenarios. These examples emphasize the role of transformers in promoting specialized tasks in various industries.
Why Transformers excel in specialized tasks
The core strengths of transformers make them suitable for different applications. Scalability enables them to handle massive data sets, making them ideal for tasks that require extensive calculation. Their parallelism, made possible by the self -number mechanism, ensures faster processing than successive models such as recurrent neural networks (RNNs). The ability of Transformers to process data in parallel, has been crucial in time-sensitive applications such as real-time video analysis, whereby the processing speed directly influences the results, such as in surveillance or emergency assistance systems.
Transfer learning further improves their versatility. Models such as GPT-3 or VIT can be refined for domain-specific needs, which considerably reduces the required drugs for training. This adaptability enables developers to reuse existing models for new applications, to save time and computational sources. For example, the Transformers library of Hugging Face offers many pre-trained models that researchers have adapted for niche fields such as summarization of legal document and analysis of the agricultural crops.
The adaptability of their architecture also makes transitions possible between modalities, from text to images, sequences and even genomic data. Genoom sequencing and analysis, driven by transformer architectures, have improved precision in identifying genetic mutations related to hereditary diseases, which underlines their usefulness in health care.
AI architectures reconsider for the future
As Transformers expand their reach, the AI community restores the architectural design to maximize efficiency and specialization. Emerging models such as Line And Large bird Address computational bottlenecks by optimizing the use of memory use. These progress ensure that transformers remain scalable and accessible as their applications grow. Linformer, for example, reduces the quadratic complexity of standard transformers, making it feasible to process longer sequences with a fraction of the costs.
Hybrid approaches also gain popularity and combine transformers with symbolic AI or other architectures. These models excel in tasks that require both deep learning and structured reasoning. For example, hybrid systems are used in legal document analysis, in which transformers extract context, while symbolic systems ensure compliance with regulating frameworks. This combination bridges the unstructured and structured data gap, making more holistic AI solutions possible.
Specialized customized transformers for specific industries are also available. Healthcare -specific models such as Path Can cause a revolution in predictive diagnostics by analyzing Pathology Dears with unprecedented accuracy. Likewise, climate -oriented transformers to improve environmental modeling, predicting weather patterns or simulating climate change scenarios. Open-source frameworks such as cuddling face are crucial in democratizing access to these technologies, so that smaller organizations can use advanced AI without priceless costs.
Challenges and barriers for expanding transformers
While innovations such as OpenAi’s scanty Mechanisms have contributed to reducing the calculation tax, making these models more accessible, the general requirements of the resources are still a barrier for widespread acceptance.
Data dependence is a different obstacle. Transformers require huge, high -quality data sets, which are not always available in specialized domains. Tackling this scarcity often includes synthetic data generation or transfer learning, but these solutions are not always reliable. New approaches, such as data enlargement and federal learning, are on the rise to help, but they come up with challenges. In health care, for example, generating synthetic data sets that are accurately reflected of the real-world diversity while the protection of the patient remains a challenging problem.
Another challenge is the ethical implications of transformers. These models can unintentionally strengthen prejudices in the data on which they are trained. This can lead to unfair and discriminatory results
In sensitive areas such as recruitment or law enforcement.
The integration of transformers with quantum computing could further improve scalability and efficiency. Quantum transformers can make breakthroughs possible in cryptography and drug synthesis, where the calculation requirements are exceptionally high. For example, IBMs Work on combining Kwantum Computing with AI already shows promising when solving optimization problems that were previously deemed unmanageable. As models become more accessible, the adaptability of the cross-domain is likely to become the norm, which stimulates innovation in areas to explore the potential of AI.
The Bottom Line
Transformers have really changed the game to AI and go much further than their original role in language processing. Nowadays they have an influence on health care, robotics and finance, solving problems that once seemed impossible. Their ability to handle complex tasks, process large amounts of data and to work in real time is to open new possibilities in various industries. But with all this progress there are challenges – such as the need for quality data and the risk of bias.
As we continue, we must continue to improve these technologies and at the same time consider their ethical and environment impact. By embracing and combining new approaches with emerging technologies, we can ensure that Transformers help us build a future where AI benefits everyone.