The Rise of Small Reasoning Models: Can Compact AI Match GPT-Level Reasoning?
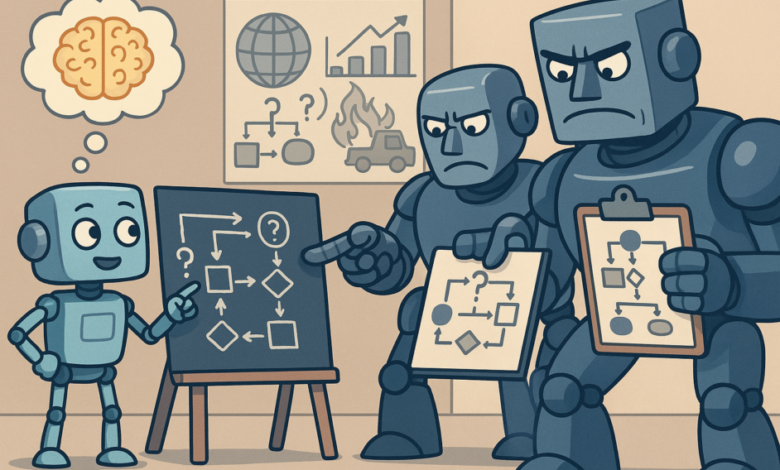
In recent years, the AI field has been fascinated by the success of large language models (LLMS). Initially designed for the processing of natural language, these models have evolved into powerful reasoning aids that are able to tackle complex problems with human -like step -by -step thinking process. Despite their exceptional reasoning possibilities, however, LLMS have considerable disadvantages, including high calculation costs and slow implementation speeds, making them impractical for Real-World use in environments in sources such as mobile devices or Edge Computing. This has led to a growing interest in developing smaller, more efficient models that can offer similar reasoning options and at the same time minimize costs and resources. This article investigates the rise of these small reasoning models, their potential, challenges and implications for the future of AI.
A shift in perspective
For a large part of the recent history of AI, the field has followed the principle of ‘scale laws’, which suggests that model performance improves predictably as data, calculating power and the increase in model size. Although this approach has produced powerful models, it has also resulted in considerable considerations, including high infrastructure costs, impact on the environment and latency issues. Not all applications require the full possibilities of solid models with hundreds of billions of parameters. In many practical cases-as with devices, Skaller models can achieve comparable results if they can reasonably reason.
Understand reasoning in AI
Reasoning in AI refers to the ability of a model to follow logical chains, to understand cause and effect, to divert implications, plan in a process and identify contradictions. For language models, this often means not only the collection of information, but also manipulating and distracting information via a structured, step -by -step approach. This level of reasoning is usually achieved by refining LLMS to perform Multi-Step reasoning before you come to an answer. Although effective, these methods require significant calculation sources and can be slow and expensive to implement, which expresses concern about their accessibility and environment impact.
Insight into small reasoning models
Small reasoning models are intended to replicate the reasoning options of large models, but with greater efficiency in terms of computing power, memory use and latency. These models often use a technique called Knowledge DestillationWhere a smaller model (the “student”) learns from a larger, pre -trained model (the “teacher”). The distillation process includes the training of the smaller model on data generated by the larger, with the aim of transferring reasoning power. The student model is then refined to improve performance. In some cases, reinforcement education with specialized domain -specific remuneration functions is used to further improve the power of the model to perform task -specific reasoning.
The rise and progress of small reasoning models
A remarkable milestone in the development of small reasoning models came with the release of Deepseek-R1. Although he was trained on a relatively modest cluster of older GPUs, Deepseek-R1 achieved performance that is comparable to larger models such as OpenAI’s O1 on benchmarks such as MMLU and GSM-8K. This performance has led to a reconsideration of the traditional scale approach, which assumed that larger models were inherently superior.
The success of Deepseek-R1 can be attributed to the innovative training process, which combined large-scale reinforcement learning without trusting guided refinements in the early phases. This innovation led to the creation of Deepseek-R1-ZeroA model that demonstrated impressive reasoning options compared to large reasoning models. Further improvements, such as the use of cold starting data, have improved the coherence of the model and the implementation of the model, in particular in areas such as mathematics and code.
Moreover, distillation techniques have proven to be crucial in developing smaller, more efficient models of larger ones. Deepseek, for example, has released distilled versions of its models, with sizes ranging from 1.5 billion to 70 billion parameters. With the help of these models, researchers have trained relatively a much smaller model Deepseek-R1-Distill-QWEN-32B He then surpasses OpenAi’s O1-Mini About different benchmarks. These models can now be used with standard hardware, making them a more feasible option for a wide range of applications.
Small models may match the reasoning at GPT level
To assess whether small reasoning models (SRMs) can match the reasoning power of large models (LRMs) such as GPT, it is important to evaluate their performance in standard benchmarks. For example the Deepseek-R1 model scored about 0.844 on the Mmlu testSimilar to larger models such as O1. On the GSM-8K Dataset, which focuses on mathematics at the learning school, the distilled model of Deepseek-R1 reaches Top performance, which surpasses both O1 and O1-Mini.
When coding tasks, such as those on Livecodebench And CodeforcesDeepseek-R1’s distilled models executed Just like O1-Mini and GPT-4O, which demonstrates strong reasoning options in programming. However, larger models still have one edge In tasks that require broader language comprehension whether long context windows have to handle, because smaller models are usually more task -specific.
Despite their strengths, small models can struggle with extensive reasoning tasks or when they are confronted with data outside the distribution. In LLM Chess simulations, for example, Deepseek-R1 made more errors than larger models, indicating limitations in the ability to maintain focus and accuracy for long periods.
Decisions and practical implications
The considerations between model size and performance are crucial in comparing SRMs with GPT level LRMs. Smaller models require fewer memory and computing power, making them ideal for Edge devices, mobile apps or situations where offline conclusion is needed. This efficiency results in lower operational costs, with models such as Deepseek-R1 a maximum of 96% cheaper To turn larger models such as O1.
However, these efficiency profits come with a few compromises. Smaller models are usually refined for specific tasks that can limit their versatility compared to larger models. While Deepseek-R1, for example, excels in mathematics and coding, missing Multimodal possibilities, such as the possibility of interpreting images, that can handle larger models such as GPT-4O.
Despite these limitations, the practical applications of small reasoning models are enormous. In healthcare they can feed diagnostic aids that analyze medical data about standard hospital servers. In education they can be used to develop personalized tutor systems and to give step -by -step feedback to students. In scientific research they can help with data analysis and hypotheset tests in areas such as mathematics and physics. The open-source character of models such as Deepseek-R1 also promotes cooperation and democratizes access to AI, allowing smaller organizations to benefit from advanced technologies.
The Bottom Line
The evolution of language models for smaller reasoning models is an important progress in AI. Although these models may not yet fully match the broad possibilities of large language models, they offer important benefits in efficiency, cost -effectiveness and accessibility. By finding a balance between reasoning power and the efficiency of resources, smaller models will play a crucial role in various applications, making AI more practical and more sustainable for use in practice.