The LLM Car: A Breakthrough in Human-AV Communication

As autonomous vehicles (AVs) inch closer to widespread adoption, a key challenge remains: bridging the communication gap between human passengers and their robot drivers. While AVs have made remarkable progress in navigating complex road environments, they often struggle to interpret the nuanced, natural language commands that come so easily to human drivers.
Enter one innovative study from Purdue University’s Lyles School of Civil and Construction Engineering. Led by Assistant Professor Ziran Wang, a team of engineers has developed an innovative approach to improve AV-human interaction using artificial intelligence. Their solution is to integrate large language models (LLMs) such as ChatGPT into autonomous driving systems.”
The power of natural language in AVs
LLMs represent a leap forward in AI’s ability to understand and generate human-like text. These advanced AI systems are trained on large amounts of textual data, allowing them to grasp context, nuance, and implicit meaning in ways that traditional programmed responses cannot.
In the context of autonomous vehicles, LLMs offer a transformative capability. Unlike conventional AV interfaces that rely on specific voice commands or button input, LLMs can interpret a wide range of natural language instructions. This means passengers can interact with their vehicles in much the same way as they would with a human driver.
The improvement in AV communication capabilities is significant. Imagine telling your car, “I’m late,” and having it automatically calculate the most efficient route, adapting driving style to safely reduce travel time. Or consider the possibility of saying, “I’m a little car sick,” causing the vehicle to adjust its motion profile for a smoother ride. These nuanced interactions, which human drivers understand intuitively, become possible for AVs through the integration of LLMs.
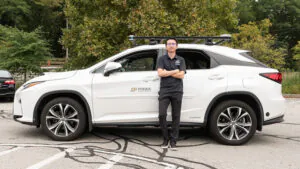
Purdue University assistant professor Ziran Wang stands next to an autonomous test vehicle that he and his students have equipped to interpret passenger commands using ChatGPT or other large language models. (Purdue University photo/John Underwood)
The Purdue Study: Methodology and Findings
To test the potential of LLMs in autonomous vehicles, the Purdue team conducted a series of experiments using a level four autonomous vehicle – just one step away from full autonomy as defined by SAE International.
The researchers began training ChatGPT to respond to a range of commands, from direct instructions such as “Please drive faster” to more indirect requests such as “I’m feeling a little motion sick right now.” They then integrated this trained model with the vehicle’s existing systems, allowing it to take into account factors such as traffic rules, road conditions, weather and sensor data when interpreting commands.
The experimental design was rigorous. Most of the testing was conducted at a proving ground in Columbus, Indiana – a former airport runway that allowed for safe, high-speed testing. Additional parking testing was conducted on the grounds of Purdue’s Ross-Ade Stadium. During the experiments, the LLM-assisted AV responded to both pre-learned and novel commands from passengers.
The results were promising. Participants reported significantly less discomfort compared to typical experiences in level four AVs without LLM support. The vehicle consistently outperformed standard safety and comfort metrics, even when responding to commands for which it had not been explicitly trained.
Perhaps most impressively, the system demonstrated the ability to learn and adapt to the preferences of individual passengers during a ride, demonstrating the potential for truly personalized autonomous transportation.
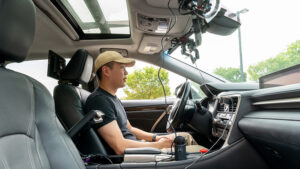
Purdue PhD candidate Can Cui takes a ride in the autonomous test vehicle. A microphone in the console picks up his commands, which interpret large language models in the cloud. The vehicle drives according to instructions generated from the large language models. (Purdue University photo/John Underwood)
Implications for the future of transportation
For users, the benefits are numerous. The ability to interact naturally with an AV shortens the learning curve associated with new technology, making autonomous vehicles more accessible to a wider range of people, including those who may be intimidated by complex interfaces. Additionally, the personalization capabilities demonstrated in the Purdue study suggest a future where AVs can adapt to individual preferences, providing each passenger with a customized experience.
This improved interaction could also increase safety. By better understanding the passenger’s intent and state – for example, by recognizing when someone is in a hurry or not feeling well – AVs can adjust their driving behavior accordingly, potentially reducing the number of accidents due to miscommunication or passenger discomfort .
From an industry perspective, this technology could be a key differentiator in the competitive AV market. Manufacturers that can provide a more intuitive and responsive user experience can gain a significant advantage.
Challenges and future directions
Despite the promising results, several challenges remain before LLM-integrated AVs become a reality on public roads. An important problem is the processing time. The current system takes an average of 1.6 seconds to interpret and respond to a command – acceptable for non-critical scenarios, but potentially problematic in situations requiring quick responses.
Another major concern is the potential for LLMs to ‘hallucinate’ or misinterpret assignments. Although the study includes safeguards to mitigate this risk, comprehensively addressing this issue is critical to real-world implementation.
Looking ahead, Wang’s team is exploring several avenues for further research. They evaluate other LLMs, including Google’s Gemini and Meta’s Llama AI assistants, to compare performance. Preliminary results suggest that ChatGPT currently outperforms others in terms of security and efficiency, although published findings will be available soon.
An intriguing future direction is the potential for vehicle-to-vehicle communications using LLMs. This could enable more advanced traffic management, such as AVs that prioritize intersections.
In addition, the team is embarking on a project to study large vision models – AI systems trained on images rather than text – to help AVs navigate extreme winter weather conditions common in the Midwest. This research, supported by the Center for Connected and Automated Transportation, could further improve the adaptability and safety of autonomous vehicles.
The bottom line
Purdue University’s groundbreaking research into integrating large language models with autonomous vehicles marks a pivotal moment in transportation technology. By enabling more intuitive and responsive human-AV interaction, this innovation addresses a critical challenge in AV adoption. While obstacles such as processing speed and potential misinterpretations remain, the research’s promising results pave the way for a future where communicating with our vehicles could be as natural as talking to a human driver. As this technology evolves, it has the potential to revolutionize not only the way we travel, but also the way we perceive and interact with artificial intelligence in our daily lives.