The AI Price War: How Lower Costs Are Making AI More Accessible
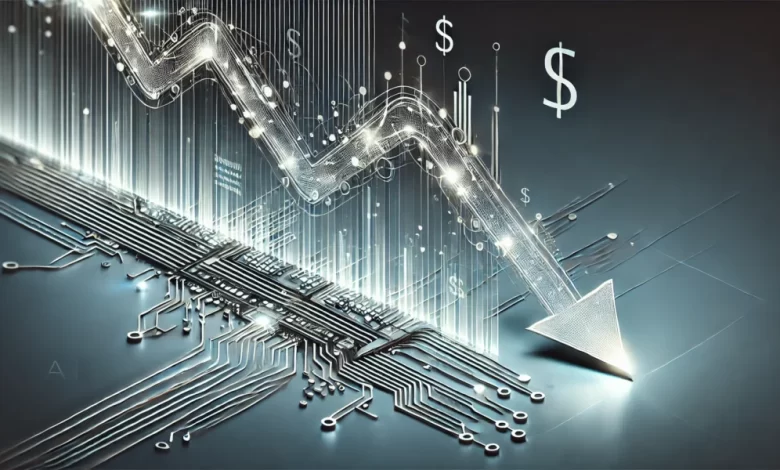
Ten years ago, the development of artificial intelligence (AI) was something that only large companies and well-funded research institutions could afford. The required costs for hardware, software and data storage were very high. But a lot has changed since then. It all started in 2012 with AlexNet, a deep learning model that showed the true potential of neural networks. This was a game changer. Then in 2015, Google released TensorFlow, a powerful tool that made advanced machine learning libraries available to the public. This move was critical in reducing development costs and encouraging innovation.
The momentum continued in 2017 with the introduction of transformer models such as BERT and GPT, which revolutionized natural language processing. These models made AI tasks more efficient and cost-effective. By 2020, OpenAI’s GPT-3 has set new standards for AI capabilities, highlighting the high cost of training such large models. For example, training an advanced AI model like OpenAI’s GPT-3 in 2020 would take approx $4.6 millionputting advanced AI out of reach for most organizations.
By 2023, further developments such as more efficient algorithms and specialized hardware, such as NVIDIA’s A100 GPUshad continued to reduce the costs of AI training and implementation. These steady cost reductions have created an AI price war, making advanced AI technologies more accessible to a wider range of industries.
Key players in the AI price war
The AI price war involves major tech giants and smaller startups, each playing a crucial role in lowering costs and making AI more accessible. Companies like Google, Microsoft and Amazon are leading the way, using their vast resources to innovate and cut costs. Google has made significant strides with technologies such as Tensor Processing Units (TPUs) and the TensorFlow framework, which significantly reduces the cost of AI operations. These tools will allow more people and companies to use advanced AI without incurring huge costs.
Similarly, Microsoft Azure offers AI services that are scalable and affordable, allowing companies of all sizes to integrate AI into their operations. This has leveled the playing field, giving small businesses access to previously exclusive technologies for large companies. The same goes for its AWS offerings, including SageMakerAmazon simplifies the process of building and deploying AI models, allowing companies to start using AI quickly and with minimal hassle.
Startups and smaller companies are playing a vital role in the AI price war. They are introducing innovative and cost-effective AI solutions, challenging the dominance of larger companies and driving the industry forward. Many of these smaller players are using open source tools, which help reduce their development costs and promote greater competition in the market.
The open source community is essential in this context, providing free access to powerful AI tools such as PyTorch and Keras. Additionally, open-source datasets such as ImageNet and Common Crawl are invaluable resources that developers use to build AI models without significant investment.
Large companies, startups and open source contributors are reducing the cost of AI and making the technology more accessible to businesses and individuals around the world. This competitive environment drives down prices and drives innovation, continually pushing the boundaries of what AI can achieve.
Technological progress ensures cost savings
Advances in hardware and software have been critical in reducing AI costs. Specialized processors such as GPUs and TPUs, designed for intensive AI computation, have outperformed traditional CPUs, reducing both development time and costs. Software improvements have also contributed to cost efficiency. Techniques such as model pruning, quantization, and knowledge distillation create smaller, more efficient models that require less power and storage, enabling deployment across devices.
Cloud computing platforms such as AWS, Google Cloud and Microsoft Azure provide scalable, cost-effective AI services on a pay-as-you-go model, reducing the need for significant upfront infrastructure investments. Edge computing further reduces costs by processing data closer to the source, reducing data transfer costs and enabling real-time processing for applications such as autonomous vehicles and industrial automation. These technological advancements expand the reach of AI, making it more affordable and accessible.
Economies of scale and investment trends have also had a significant impact on AI pricing. As AI adoption increases, development and implementation costs decrease as fixed costs are spread over larger units. Venture capital investments in AI startups have also played a key role in reducing costs. These investments enable startups to scale and innovate quickly, bringing cost-effective AI solutions to market. The competitive financing environment encourages startups to cut costs and improve efficiency. This environment supports continued innovation and cost reduction, benefiting businesses and consumers.
Market responses and democratization of AI
As AI costs decrease, consumers and businesses have quickly adopted these technologies. Companies use affordable AI solutions to improve customer service, optimize operations and create new products. AI-powered chatbots and virtual assistants have become commonplace in customer service, providing efficient support. Lower AI costs have also had significant impacts globally, especially in emerging markets, helping companies compete globally and increase economic growth.
No-code and low-code platforms and AutoML tools further democratize AI. These tools simplify the development process, allowing users with minimal programming skills to create AI models and applications, reducing development time and costs. AutoML tools automate complex tasks such as data preprocessing and feature selection, making AI accessible even to non-experts. This will increase the impact of AI across industries and allow companies of all sizes to benefit from AI’s capabilities.
The implications of AI cost reduction for industry
Reducing the cost of AI results in widespread adoption and innovation across industries, transforming companies’ operations. AI improves diagnostics and treatments in healthcare, with tools such as IBM Watson Health And Zebra medical vision providing better access to advanced care.
Likewise, AI personalizes customer experiences and optimizes retail operations, with companies like Amazon and Walmart leading the way. Smaller retailers are also adopting these technologies, increasing competition and fostering innovation. In finance, AI improves fraud detection, risk management and customer service, at banks and companies such as Ant financial using AI to assess creditworthiness and expand access to financial services. These examples show how lower AI costs drive innovation and expand market opportunities across industries.
Challenges and risks associated with lower AI costs
While lower AI costs have enabled broader adoption, they also come with hidden costs and risks. Data privacy and security are major concerns as AI systems often handle sensitive information. Ensuring regulatory compliance and securing these systems can increase project costs. Additionally, AI models require constant updates and monitoring to remain accurate and effective, which can be costly for companies without specialized AI teams.
The desire to cut costs could compromise the quality of AI solutions. High-quality AI development requires large, diverse data sets and significant computing resources. Cutbacks can lead to less accurate models, which affects reliability and user confidence. Furthermore, as AI becomes more accessible, the risk of misuse increases, such as creating deepfakes or automating cyber-attacks. AI can also increase bias if trained on biased data, leading to unfair results. Addressing these challenges requires careful investments in data quality, model maintenance, and strong ethical practices to ensure responsible AI use.
The bottom line
As AI becomes more affordable, its impact across industries becomes clearer. Lower costs make advanced AI tools accessible to companies of all sizes, driving innovation and competition on a global scale. AI-powered solutions are now part of daily operations, improving efficiency and creating new growth opportunities.
However, the rapid adoption of AI also brings challenges that need to be addressed. Lower costs can hide data privacy, security and ongoing maintenance costs. Ensuring compliance and protecting sensitive data increases the overall cost of AI projects. There is also a risk that AI quality will be compromised if cost-cutting measures compromise data quality or computing resources, leading to flawed models.
Stakeholders must work together to balance the benefits of AI with its risks. Investing in high-quality data, robust testing, and continuous improvement will maintain AI integrity and build trust. Promoting transparency and fairness ensures that AI is used ethically, enriching business and improving the human experience.