The AI Feedback Loop: When Machines Amplify Their Own Mistakes by Trusting Each Other’s Lies
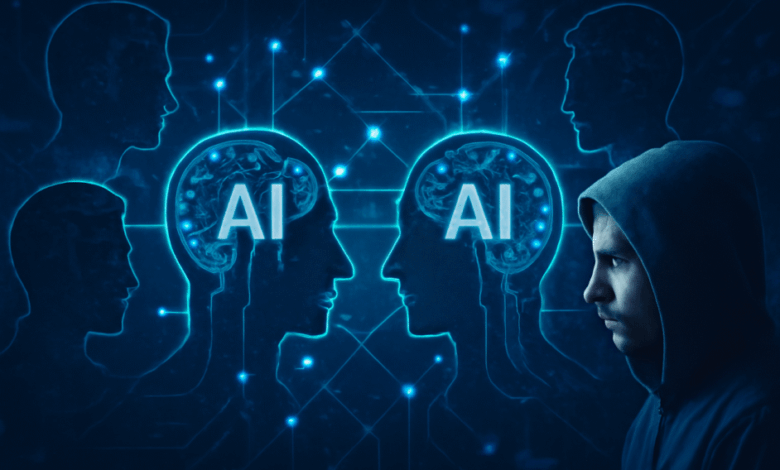
Because companies are increasingly dependent on artificial intelligence (AI) to improve activities and customer experiences, growing care is on the rise. Although AI has proven to be a powerful tool, it also entails a hidden risk: the AI feedback run. This happens when AI systems are trained on data that includes output from other AI models.
Unfortunately, these outputs can sometimes contain errors, which are strengthened every time they are reused, creating a cycle of errors that gets worse over time. The consequences of this feedback job can be serious, which leads to companies, damage to the reputation of a company and even legal complications if they are not correctly managed.
What is an AI feedback loop and how does this influence AI models?
An AI feedback job occurs when the output of one AI system is used as input to train another AI system. This process is common in machine learning, where models are trained on large datasets to make predictions or generate results. However, when the output of one model is traced back into another model, it creates a loop that can improve the system or in some cases can introduce new errors.
For example, if an AI model is trained on data with content generated by another AI, any errors of the first AI, such as misunderstanding a subject or providing incorrect information, can be passed on as part of the training data for the second AI. As this process repeats itself, these errors can get worse, which means that the performance of the system is broken down over time and make it more difficult to identify and repair inaccuracies.
AI models learn from huge amounts of data to identify patterns and make predictions. For example, the recommendation engine of an e-commerce site can suggest products based on the browsing history of a user, which refines the suggestions as the more data processes. However, if the training data is inadequate, especially if it is based on the outputs of other AI models, it can replicate and even strengthen these errors. In industries such as health care, where AI is used for critical decision-making, a biased or inaccurate AI model can lead to serious consequences, such as incorrect diagnoses or incorrect treatment recommendations.
The risks are particularly high in sectors that depend on AI for important decisions such as finance, health care and legislation. In these areas, errors in AI outputs can lead to a significant financial loss, legal disputes or even damage to individuals. As AI models continue to train on their own output, compound errors are likely to be anchored in the system, leading to more serious and more difficult problems.
AI -Hallucinations phenomenon
AI -Hallucinations occur when a machine output generates that seems plausible but is completely untrue. For example, an AI-Chatbot can provide manufactured information with confidence, such as a non-existent company policy or an invented statistics. In contrast to man-generated mistakes, AI-Hallucinations may seem authoritative, making it difficult to recognize, especially when the AI is trained on content generated by other AI systems. These errors can vary from small errors, such as incorrectly quoted statistics, to more serious, such as fully manufactured facts, incorrect medical diagnoses or misleading legal advice.
The causes of AI -Hallucinations can be reduced to various factors. An important problem is when AI systems are trained on data from other AI models. If an AI system generates incorrect or biased information and this export is used as training data for another system, the error is transferred. In the course of time this creates an environment in which the models begin to trust these lies and reproduce as legitimate data.
Moreover, AI systems are highly dependent on the quality of the data on which they are trained. If the training data is inadequate, incomplete or biased, the output of the model will reflect those imperfections. A data set with sexual or racial prejudices can, for example, lead to AI systems that generate biased predictions or recommendations. Another contributing factor is Overfitting, where a model becomes exaggerated on specific patterns in the training data, making it more likely to generate inaccurate or nonsensical outputs when confronted with new data that do not fit in those patterns.
AI-hallucinations can cause important problems in real-world scenarios. AI-driven aids for generating content such as GPT-3 and GPT-4 can, for example, produce articles that contain manufactured quotes, fake sources or incorrect facts. This can be the credibility of organizations that harm these systems. Likewise, AI-driven customer service bots can offer misleading or completely false answers, which can lead to dissatisfaction of customers, damaged trust and potential legal risks for companies.
How to strengthen Feedback Klussen errors and influence the Real-World companies
The danger of AI feedback klussen lies in their ability to strengthen minor errors in important problems. When an AI system makes an incorrect prediction or offers an incorrect output, this error can influence the following models on that data. As this cycle continues, errors are strengthened and enlarged, which leads to increasingly worse performance. Over time, the system becomes more confident in its mistakes, making it more difficult for human supervision to detect and correct them.
Feedback klussen can have serious real consequences in industries such as finance, health care and e-commerce. In financial prediction, AI models that are trained for defective data can, for example, yield inaccurate predictions. When these predictions influence future decisions, errors become intensification, leading to poor economic results and considerable losses.
In e-commerce, AI recommendation engines that depend on biased or incomplete data can ultimately promote the content that strengthens stereotypes or prejudices. This can create ultrasound rooms, polarize the public and throw out customer confidence, ultimately damage the sale and brand reputation.
Similarly, in customer service AI chatbots that have been trained on defective data can offer incorrect or misleading answers, such as incorrect return policy or defective product details. This leads to the dissatisfaction of customers, eroded trust and potential legal issues for companies.
In the health care sector, AI models used for medical diagnoses can reproduce errors if trained on biased or defective data. A wrong diagnosis of one AI model can be passed on to future models, the problem worsening and endangering the health of patients.
Reducing the risks of AI feedback klussen
To reduce the risks of AI feedback klussen, companies can take different steps to ensure that AI systems remain reliable and accurate. Firstly, the use of various high -quality training data is essential. When AI models are trained on a wide range of data, they are less likely to make biased or incorrect predictions that can lead to errors that build up over time.
Another important step is to include human supervision via (HITL) systems (HITL). By having human experts assess AI-generated output before they are used to train further models, companies can ensure that errors are caught early. This is especially important in industries such as health care or finance, where accuracy is crucial.
Regular audits from AI systems help detect errors early, so that they do not spread through feedback klussen and later cause greater problems. Current checks enable companies to identify when something goes wrong and make corrections before the problem is too widespread.
Companies must also consider using AI error detection tools. These tools can help recognize errors in AI outputs before they cause considerable damage. By marking errors early, companies can intervene and prevent the spread of inaccurate information.
Looking ahead, emerging AI trends companies offer new ways to manage feedback klussen. New AI systems are being developed with built-in error control functions, such as self-correction algorithms. Moreover, regulators emphasize a larger AI transparency, which encourages companies to assume practices that make AI systems more understandable and responsible.
By following these best practices and staying informed of new developments, companies can get the most from AI, while minimizing the risks. Focusing on ethical AI practices, good data quality and clear transparency will be essential to use AI safely and effectively in the future.
The Bottom Line
The AI Feedback Loop is a growing challenge that companies have to take on to fully use AI’s potential. Although AI offers enormous value, the ability to strengthen errors has significant risks ranging from incorrect predictions to large business disturbances. As AI systems become integral for decision-making, it is essential to implement guarantees, such as the use of different and high-quality data, the absorption of human supervision and performing regular audits.