Synthetic Data: A Double-Edged Sword for the Future of AI
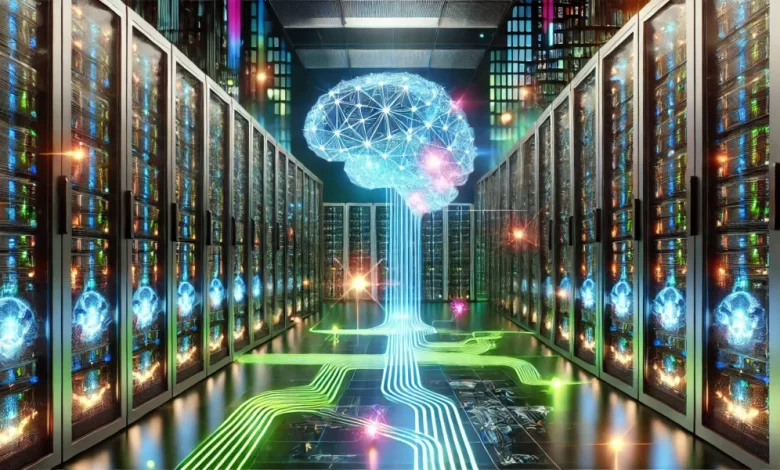
The rapid growth of artificial intelligence (AI) has created a huge demand for data. Traditionally, organizations have relied on real-world data—such as images, text, and audio—to train AI models. This approach has made significant progress in areas such as natural language processing, computer vision and predictive analytics. However, as the availability of real-world data reaches its limitsare synthetic data emerging as a critical resource for AI development. While promising, this approach also introduces new challenges and implications for the future of technology.
The rise of synthetic data
Synthetic data is artificially generated information designed to replicate the characteristics of real-world data. It is created using algorithms and simulations, which allows the production of data designed to serve specific needs. For example, generative adversaries (GANs) can produce photorealistic images, while simulation engines generate scenarios for training autonomous vehicles. According to GartnerSynthetic data is expected to become the primary source for AI training by 2030.
This trend is driven by several factors. First, the growing demands of AI systems are outpacing the speed at which humans can produce new data. As real-world data becomes increasingly scarce, synthetic data provides a scalable solution to meet these demands. Generative AI tools such as Openai’s Chatgpt and Google’s Gemini contribute further by generating large amounts of text and images, increasing performance of synthetic content online. Consequently, it is becoming increasingly difficult to distinguish between original and AI-generated content. With the growing use of online data for training AI models, synthetic data will likely play a crucial role in the future of AI development.
Efficiency is also a key factor. Preparing real-world datasets—from collection to labeling—is possible be good for up to 80% of AI development time. Synthetic data, on the other hand, can be generated faster, more cost-effectively, and customized for specific applications. Companies like Nvidia,, MicrosoftAnd Synthesis AI have adopted this approach, using synthetic data to supplement or even replace real-world data sets in some cases.
The benefits of synthetic data
Synthetic data offers numerous benefits to AI, making it an attractive alternative for companies looking to scale their AI efforts.
One of the most important benefits is the limitation of privacy risks. Legal frameworks such as GDPR and CCPA Place strict requirements on the use of personal data. By using synthetic data that closely resembles real-world data without revealing sensitive information, companies can adhere to these regulations and continue training their AI models.
Another advantage is the ability to create balanced and unbiased data sets. Real-world data often reflects societal biases, leading to AI models that unintentionally perpetuate these biases. Synthetic data allows developers to carefully craft datasets to ensure fairness and inclusivity.
Synthetic data also allows organizations to simulate complex or rare scenarios that can be difficult or dangerous to replicate in the real world. For example, training autonomous drones to navigate dangerous environments can be accomplished safely and efficiently with synthetic data.
In addition, synthetic data can provide flexibility. Developers can generate synthetic datasets to include specific scenarios or variations that may be underrepresented in real-world data. For example, synthetic data can simulate various weather conditions for training autonomous vehicles, allowing the AI to perform reliably in rain, snow, or fog – conditions that may not be extensively captured in real driving datasets.
Moreover, synthetic data is scalable. Generating data algorithmically allows companies to create massive data sets at a fraction of the time and cost required to collect and label real-world data. This scalability is particularly beneficial for startups and smaller organizations that lack the resources to amass large data sets.
The risks and challenges
Despite its advantages, synthetic data is not without limitations and risks. One of the most pressing concerns is the potential for inaccuracies. If synthetic data does not accurately represent real-world patterns, the AI models trained on it can perform poorly in practical applications. This issue, often referred to as model collapse, highlights the importance of maintaining a strong link between synthetic and real-world data.
Another limitation of synthetic data is its inability to capture the full complexity and unpredictability of real-world scenarios. Real-world data sets inherently reflect the nuances of human behavior and environmental variables, which are difficult to replicate via algorithms. AI models trained only on synthetic data may struggle to generalize effectively, leading to suboptimal performance when deployed in dynamic or unpredictable environments.
Moreover, there is also the risk of too much dependence on synthetic data. While it can supplement real-world data, it cannot completely replace it. AI models still require a degree of grounding in real-world observations to maintain reliability and relevance. Over-reliance on synthetic data can lead to models that do not generalize effectively, especially in dynamic or unpredictable environments.
Ethical concerns also play a role. While synthetic data addresses some privacy concerns, it can create a false sense of security. Poorly designed synthetic data sets can unintentionally encode biases or perpetuate inaccuracies, undermining efforts to build fair and equitable AI systems. This is particularly concerning in sensitive areas such as healthcare or criminal justice, where the stakes are high, and unintended consequences can have significant implications.
Finally, generating high-quality synthetic data requires advanced tools, expertise and computational resources. Without careful validation and benchmarking, synthetic datasets may fail to meet industry standards, leading to unreliable AI results. Ensuring that synthetic data aligns with real-world scenarios is critical to its success.
The way forward
Addressing the challenges of synthetic data requires a balanced and strategic approach. Organizations should treat synthetic data as a complement rather than a replacement for real-world data, combining the strengths of both to create robust AI models.
Validation is critical. Synthetic datasets must be carefully evaluated for quality, alignment with real-world scenarios, and potential biases. Testing AI models in real environments ensures their reliability and effectiveness.
Ethical considerations must remain central. Clear guidelines and accountability mechanisms are essential to ensure responsible use of synthetic data. Efforts should also focus on improving the quality and reliability of synthetic data through advances in generative models and validation frameworks.
Collaboration across industries and academia can further improve the responsible use of synthetic data. By sharing best practices, developing standards and promoting transparency, stakeholders can collectively address challenges and maximize the benefits of synthetic data.