Speed Meets Quality: How Adversarial Diffusion Distillation (ADD) is Revolutionizing Image Generation
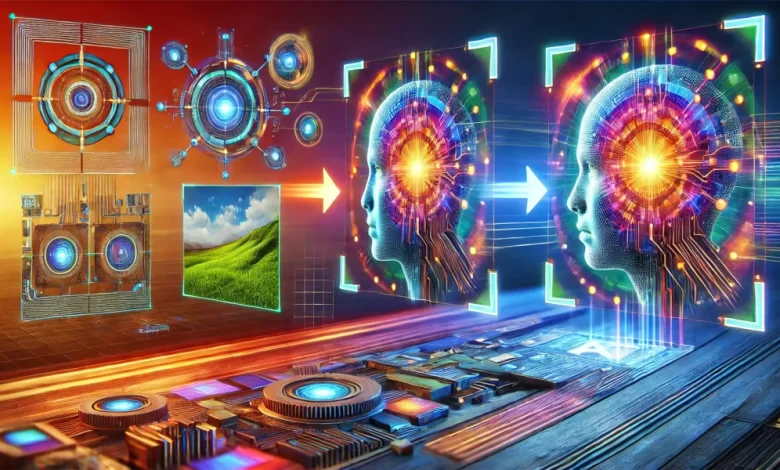
Artificial intelligence (AI) has brought about profound changes in many areas, and one area where its impact is very clear is image generation. This technology has evolved from generating simple, pixelated images to creating highly detailed and realistic images. One of the newest and most exciting developments is… Adversarial Diffusion Distillation (ADD)a technique that combines speed and quality in generating images.
The development of ADD has gone through several important phases. Initially, image generation methods were quite simple and often produced unsatisfactory results. The introduction of Genative Adversarial Networks (GANs) marked a significant improvement, allowing photorealistic images to be created using a dual-network approach. However, GANs require significant computing resources and time, which limits their practical applications.
Diffusion models represented another important advance. They iteratively refine images from random noise, resulting in high-quality output, albeit at a slower pace. The biggest challenge was finding a way to combine the high quality of diffusion models with the speed of GANs. ADD emerged as the solution, integrating the strengths of both methods. By combining the efficiency of GANs with the superior image quality of diffusion models, ADD has succeeded in transforming image generation, creating a balanced approach that improves both speed and quality.
How ADD works
ADD combines elements of both GANs and diffusion models through a three-step process:
Initialization: The process starts with a noise image, such as the initial state in diffusion models.
Diffusion Process: The noise image transforms and gradually becomes more structured and detailed. ADD accelerates this process by distilling the essential steps, reducing the number of iterations required compared to traditional diffusion models.
Contradictory training: During the diffusion process, a discriminator network evaluates the generated images and provides feedback to the generator. This hostile component ensures that the images improve in quality and realism.
Score distillation and enemy loss
In ADD, two key components, score distillation and opponent loss, play a fundamental role in quickly producing high-quality, realistic images. Below you will find details about the components.
Score distillation
Score distillation is about keeping image quality high throughout the generation process. We can see it as transferring knowledge from a super smart teacher model to a more efficient student model. This transfer ensures that the images created by the student model match the quality and detail of the images created by the teacher model.
By doing this, score distillation allows the student model to generate high-quality images in fewer steps, while maintaining excellent detail and fidelity. This step reduction makes the process faster and more efficient, which is essential for real-time applications such as gaming or medical imaging. Furthermore, it ensures consistency and reliability across different scenarios, making it essential for areas such as scientific research and healthcare, where accurate and reliable images are a must.
Contradictory loss
Adversarial loss improves the quality of the generated images by making them look incredibly realistic. This is done by including a discriminator network, a quality control that checks the images and provides feedback to the generator.
This feedback loop forces the generator to produce images so realistic that they can fool the discriminator into thinking they are real. This ongoing challenge ensures that the generator improves its performance, resulting in increasingly better image quality over time. This aspect is especially important in the creative industries, where visual authenticity is crucial.
Even if fewer steps are used in the diffusion process, adversarial loss ensures that the images do not lose their quality. The discriminator’s feedback helps the generator focus on creating high-quality images efficiently, ensuring excellent results even in low-step scenarios.
Benefits of ADD
The combination of diffusion models and adversarial training provides several important benefits:
Speed: ADD reduces the iterations required, speeding up the image generation process without sacrificing quality.
Quality: The adversarial training ensures that the images generated are high quality and highly realistic.
Efficiency: By leveraging the strengths of diffusion models and GANs, ADD optimizes computing resources, making image generation more efficient.
Recent developments and applications
Since its introduction, ADD has revolutionized several fields thanks to its innovative capabilities. Creative industries such as film, advertising and graphic design have quickly adopted ADD to produce high-quality images. For example, SDXL turbo, a recent ADD development, has reduced the number of steps required to create realistic images from 50 to just one. These advances allow film studios to produce complex visual effects faster, reducing production time and costs, while advertising agencies can quickly create eye-catching campaign images.
ADD significantly improves medical imaging and helps in early detection and diagnosis of diseases. Radiologists are improving MRI and CT scans with ADD, leading to clearer images and more accurate diagnoses. This rapid image generation is also critical for medical research, where large datasets of high-quality images are needed for training diagnostic algorithms, such as those used for early tumor detection.
Likewise, scientific research benefits from ADD by speeding up the generation and analysis of complex images with microscopes or satellite sensors. In astronomy, ADD helps create detailed images of celestial bodies, while in environmental sciences it helps monitor climate change through high-resolution satellite images.
Case study: DALL-E 2 from OpenAI
One of the most prominent examples of ADD in action is that of OpenAI DALL-E 2, an advanced image generation model that creates detailed images from textual descriptions. DALL-E 2 uses ADD to produce high-quality images at remarkable speed, demonstrating the technique’s potential to generate creative and visually appealing content.
DALL-E 2 significantly improves image quality and coherence compared to its predecessor due to the integration of ADD. The model’s ability to understand and interpret complex textual input and its rapid image generation capabilities make it a powerful tool for a variety of applications, from art and design to content creation and education.
Comparative analysis
Comparing ADD with other methods in a few steps, such as GANs and Latent consistency models highlights its clear benefits. Traditional GANs, while effective, require significant computing resources and time, while Latent Consistency Models streamline the generation process but often compromise image quality. ADD integrates the strengths of diffusion models and adversarial training, achieving superior performance in one-step synthesis and converging to state-of-the-art diffusion models such as SDXL in just four steps.
One of the most innovative aspects of ADD is its ability to achieve real-time image synthesis in one step. By drastically reducing the number of iterations required to generate images, ADD enables near-instantaneous creation of high-quality images. This innovation is especially valuable in areas that require rapid image generation, such as virtual reality, gaming and real-time content creation.
It comes down to
ADD represents an important step in image generation, combining the speed of GANs with the quality of diffusion models. This innovative approach has revolutionized various fields, from the creative industries and healthcare to scientific research and real-time content creation. ADD enables fast and realistic image synthesis by significantly reducing iteration steps, making it highly efficient and versatile.
Integrating score distillation and enemy loss ensures high-quality results, which proves essential for applications that require precision and realism. Overall, ADD stands out as a transformative technology in the era of AI-driven image generation.