Revolutionary AI Model Predicts Physical Systems Without Predefined Knowledge
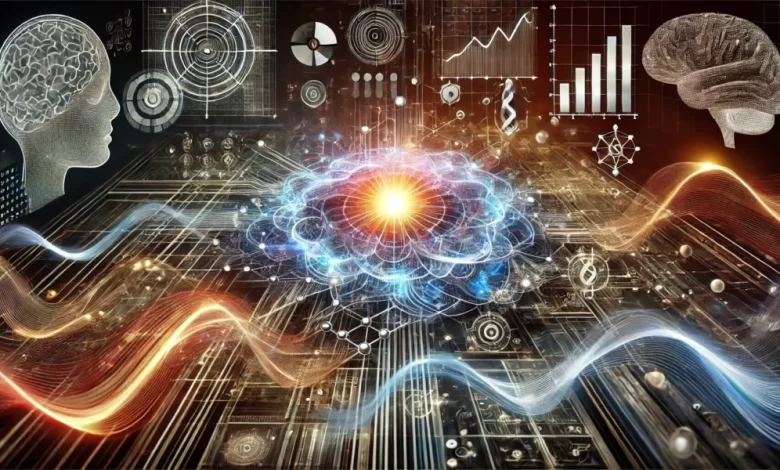
A recent study by researchers from Archetype AI has unveiled a groundbreaking AI model capable of generalizing across various physical signals and phenomena, marking a significant leap forward in the field of artificial intelligence. The paper, titled “A Phenomenological AI Basic Model for Physical Signals,“ proposes a new approach to building a unified AI model that can predict and interpret physical processes from different domains, all without prior knowledge of the underlying physical laws.
A new approach to AI for physical systems
The research aims to develop a basic AI model that can process physical signals from a wide range of systems, including electrical currents, fluid flows and optical sensor data. By hiring a phenomenological approach, the researchers avoided embedding specific physical laws into the model, allowing it to generalize to new physical phenomena it had not encountered before.
Well trained 0.59 billion sensor measurements From various domains, the model has shown exceptional performance in predicting the behavior of physical systems. These systems range from simple mechanical oscillators to complex processes such as the dynamics of the electrical grid, underscoring the versatility of the model.
A phenomenological AI framework
The research approach is based on a phenomenological framework. Unlike traditional AI models that rely on predefined inductive biases (such as conservation laws), the researchers trained their AI solely on observational data from sensors. This allows the model to learn the intrinsic patterns of various physical phenomena without assuming any prior knowledge of the governing physical principles.
By focusing on physical quantities such as temperature, electrical current and torque, the model was able to generalize across different sensor types and systems, opening the door to applications in industries ranging from energy management to advanced scientific research.
The Ω framework: a path to universal physical models
The core of this breakthrough is the Ω frameworka structured methodology developed by the researchers for creating AI models that can infer and predict physical processes. In this framework, all physical processes are represented as sets of observable quantities. The challenge in building a universal model lies in the fact that not all possible physical quantities can be measured or included in training. Nevertheless, the Ω-Framework ensures that the model can infer behavior in new systems based on the data it encounters.
This ability to generalize comes from the way the model handles incomplete or noisy sensor data, which is typical of real-world applications. The AI learns to decode and reconstruct these signals, predicting future behavior with impressive accuracy.
Transformer-based architecture for physical signals
The model’s architecture is based on transformer networks, which are often used in natural language processing but are now being applied to physical signals. These networks transform sensor data into one-dimensional patches, which are then embedded into a unified latent space. This embedding allows the model to capture the complex temporal patterns of physical signals, regardless of the specific sensor type.
Downstream phenomenological decoders then enable the model to reconstruct past behavior or predict future events, making it adaptable to a wide range of physical systems. The lightweight decoders also enable task-specific fine-tuning without having to retrain the entire model.
Validation across various physical systems
The researchers conducted extensive experiments to test the model’s generalization capabilities. In one set of tests, the model was evaluated on a spring-mass harmonic oscillator and a thermoelectric system. Both systems were known for their chaotic or complex behavior, making them ideal candidates for testing the model’s predictive accuracy.
The AI successfully predicted the behavior of these systems with minimal errors, even during chaotic phases. This success highlights its potential for predicting physical systems that exhibit nonlinear dynamics.
Further experiments were performed using real world dataincluded:
- Electricity consumption in different countries.
- Temperature variations in Melbourne, Australia.
- Oil temperature data of electrical transformers.
In each case, the model outperformed traditional, domain-specific models, demonstrating its ability to handle complex, real-world systems.
Zero-Shot Generalization and Versatility
One of the most exciting findings from this research is the model’s zero-shot generalization ability. The AI was able to predict behavior in systems it had never encountered during training, such as thermoelectric behavior and the dynamics of electrical transformers, with a high degree of accuracy.
This capability mirrors the performance of natural language models, such as GPT-4, where a single model trained on a massive data set can outperform models specialized for specific tasks. This breakthrough could have far-reaching consequences for AI’s ability to interpret physical processes.
Implications for industry and research
The possible applications of this AI base model are huge. By switching on sensor-agnostic systemsthe model can be used in domains where collecting large, specialized data sets is difficult. The ability to learn autonomously from observational data could lead to the development of self-learning AI systems that adapt to new environments without human intervention.
Moreover, this model holds great promise for scientific discovery. In areas such as physics, materials science and experimental research, where data is often complex and multi-dimensional, the model could speed up the analysis process and provide insights previously inaccessible with traditional methods.
Future directions
While the model represents significant progress in the field of AI for physical systems, the study also identifies areas for further research. These include refining the model’s handling of sensor-specific noise, examining its performance on non-periodic signals, and addressing corner cases where the predictions were less accurate.
Future work could also focus on developing more robust decoders for specific tasks, such as anomaly detection, classification, or handling edge cases in complex systems.
Conclusion
The introduction of this Phenomenological AI basic model for physical signals marks a new chapter in AI’s ability to understand and predict the physical world. With its ability to generalize across a wide range of phenomena and sensor types, this model could transform industries, scientific research and even everyday technologies. The zero-shot learning ability demonstrated in the study opens the door to AI models that can learn autonomously and adapt to new challenges, without the need for domain-specific retraining.
This groundbreaking research, led by Archetype AIwill likely have lasting impacts on the way AI is applied to physical systems, revolutionizing fields that rely on accurate and scalable predictions.