Recommender Systems Using LLMs and Vector Databases
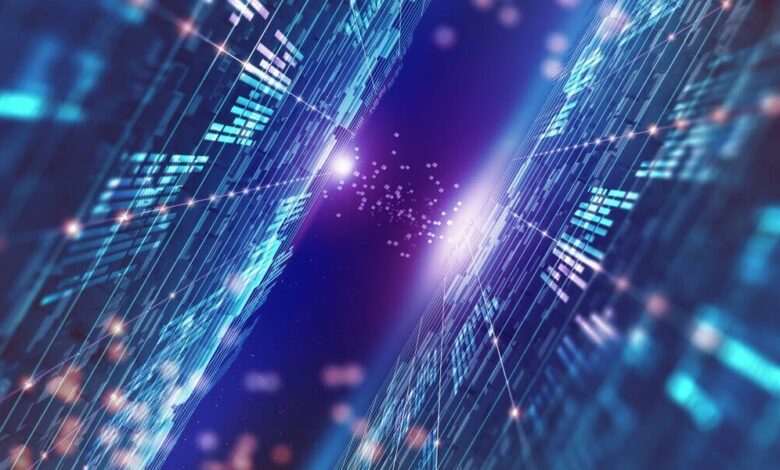
Recommendation systems are everywhere, whether you’re on Instagram, Netflix or Amazon Prime. A common element between the platforms is that they all use recommendation systems to tailor content to your interests.
Traditional recommendation systems are mainly based on three main approaches: collaborative filtering, content-based filtering, and hybrid methods. Collaborative filtering suggests items based on similar user preferences. While content-based filtering recommends items that match a user’s previous interactions. The hybrid method combines the best of both worlds.
These techniques work well, but LLM-based recommender systems excel due to the limitations of traditional systems. In this blog we discuss the limitations of traditional recommendation systems and how advanced systems can help us mitigate them.
An example of a recommendation system (Source)
Limitations of traditional recommendation systems
Despite their simplicity, traditional recommendation systems face significant challenges, such as:
- Cold start problem: It’s difficult to generate accurate recommendations for new users or items due to a lack of interaction data.
- Scalability issues: Challenges in processing large data sets and maintaining real-time responsiveness as user base and item catalogs grow.
- Personalization restrictions Over-adapting existing user preferences in content-based filtering or failing to capture nuanced tastes in collaborative filtering.
- Lack of diversity: These systems can limit users to their established preferences, leading to a lack of new or diverse suggestions.
- Data parity: Insufficient data for certain user-item pairs can hinder the effectiveness of collaborative filtering methods.
- Interpretability challenges: Difficult to explain why specific recommendations are made, especially in complex hybrid models.
How AI-powered systems outperform traditional methods
The emerging recommendation systems, especially those that integrate advanced AI techniques such as GPT-based chatbots and vector databases, are significantly more advanced and effective than traditional methods. Here’s how they’re better:
- Dynamic and conversational interactions: Unlike traditional recommendation systems that rely on static algorithms, GPT-based chatbots can engage users in real-time, dynamic conversations. This allows the system to adapt recommendations on the fly and understand and respond to nuanced user input. The result is a more personalized and engaging user experience.
- Multimodal recommendations: Modern recommendation systems go beyond text-based recommendations by incorporating data from various sources, such as images, videos, and even social media interactions.
- Context awareness: GPT-based systems excel at understanding the context of conversations and adjusting their recommendations accordingly. This means that recommendations are not only based on historical data, but are tailored to the current situation and user needs, increasing relevance.
As we have seen, LLM-based recommender systems provide a powerful way to overcome the limitations of traditional approaches. Using an LLM as a knowledge center and using a vector database for your product catalog will make creating a recommendation system much easier.
For more insights on implementing advanced AI technologies, visit Unite.ai and stay informed of the latest developments in this field.