Monetizing Research for AI Training: The Risks and Best Practices
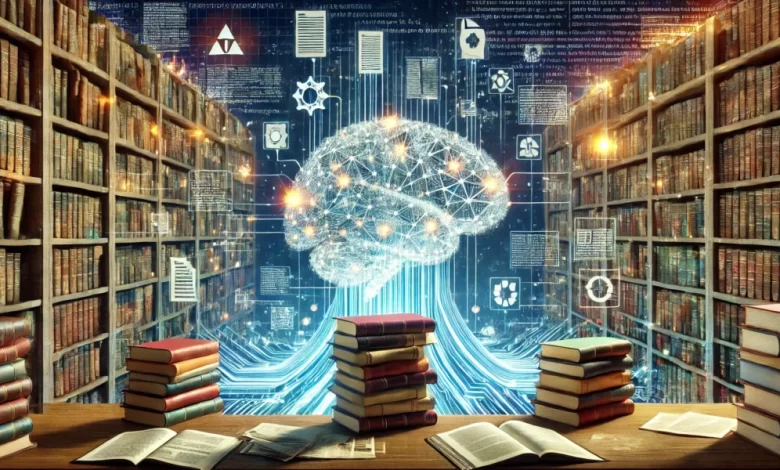
As the demand for generative AI grows, so does the appetite for high-quality data to train these systems. Scientific publishers have begun to monetize their research content to provide training data for large language models (LLMs). While this development creates a new revenue stream for publishers and enables generative AI for scientific discovery, it raises critical questions about the integrity and reliability of the research used. This raises a crucial question: are the datasets being sold reliably, and what implications does this practice have for the scientific community and generative AI models?
The rise of cash research deals
Major academic publishers, including Wiley, Taylor & Francis and others, have done so reported significant revenue from licensing their content to technology companies developing generative AI models. Wiley, for example, revealed more than $40 million in revenue from such deals this year alone. These agreements allow AI companies to access diverse and extensive scientific data sets, presumably improving the quality of their AI tools.
Publishers’ pitch is simple: licensing allows for better AI models, benefits society, and rewards authors with royalties. This business model benefits both technology companies and publishers. However, the increasing trend to monetize scientific knowledge comes with risks, especially when questionable research infiltrates these AI training datasets.
The shadow of fake research
The scientific community is no stranger to issues of fraudulent research. Studies suggest that many published findings are flawed, biased or simply unreliable. A 2020 survey found that nearly half of researchers reported problems such as selective data reporting or poorly designed field studies. More than by 2023 10,000 papers were withdrawn due to falsified or unreliable results, a number that continues to rise annually. Experts believe that this figure represents the tip of an iceberg, as there are numerous dubious studies circulating in scientific databases.
The crisis was mainly caused by “paper mills‘shadow organizations that produce fabricated studies, often in response to academic pressure in regions such as China, India and Eastern Europe. That is estimated about 2% of magazine submissions worldwide come from paper mills. These fake papers may look like legitimate research, but are packed with fictional data and unsubstantiated conclusions. It is alarming that such articles slip through peer review and end up in respected journals, jeopardizing the reliability of scientific insights. During the COVID-19 pandemic, for example flawed studies about ivermectin falsely suggested its efficacy as a treatment, sowing confusion and delaying effective public health responses. This example highlights the potential harm of spreading unreliable research, where flawed results can have a significant impact.
Implications for AI training and trust
The implications are significant if LLMs train with databases that contain fraudulent or low-quality research. AI models use patterns and relationships within their training data to generate output. If the input data is corrupted, the output may perpetuate or even amplify inaccuracies. This risk is especially high in fields like medicine, where incorrect AI-generated insights can have life-threatening consequences.
Furthermore, the issue threatens public trust in academia and AI. As publishers continue to make agreements, they must address concerns about the quality of the data sold. Failure to do so could damage the reputation of the scientific community and undermine the potential societal benefits of AI.
Ensuring reliable data for AI
Reducing the risks of flawed research distorting AI training will require a collaborative effort from publishers, AI companies, developers, researchers and the broader community. Publishers need to improve their peer-review process to catch unreliable studies before they end up in training datasets. Offering better rewards for reviewers and setting higher standards can help. An open assessment process is crucial. It ensures more transparency and accountability, which increases confidence in the research.
AI companies need to be more careful who they work with when sourcing research for AI training. Choosing publishers and journals with a strong reputation for high-quality, well-reviewed research is critical. In this context, it’s worth looking closely at a publisher’s track record, such as how often they retract articles or how open they are about their review process. Being selective improves the reliability of the data and builds trust within the AI and research communities.
AI developers must take responsibility for the data they use. This means working with experts, carefully checking research and comparing results from multiple studies. AI tools themselves can also be designed to identify suspicious data and reduce the risks of questionable research spreading further.
Transparency is also an essential factor. Publishers and AI companies should openly share details about how research is used and where royalties go. Tools like the Generative AI license agreement tracker are promising, but need wider acceptance. Researchers should also have a say in how their work is used. Opt-in policylike that of Cambridge University Pressprovide authors with control over their contributions. This builds trust, ensures fairness and ensures that authors actively participate in this process.
Furthermore, open access to high-quality research should be encouraged inclusivity and fairness in the field of AI development. Governments, nonprofits, and industry players can fund open-access initiatives, reducing dependence on commercial publishers for critical training datasets. Furthermore, the AI industry needs clear rules for ethical data sourcing. By focusing on reliable, well-reviewed research, we can build better AI tools, protect scientific integrity, and maintain public trust in science and technology.
The bottom line
Monetizing research for AI training presents both opportunities and challenges. While licensing academic content allows for the development of more powerful AI models, it also raises concerns about the integrity and reliability of the data used. Flawed research, including that of “paper mills,” can corrupt AI training datasets, leading to inaccuracies that could undermine public trust and the potential benefits of AI. To ensure AI models are based on reliable data, publishers, AI companies and developers must work together to improve peer review processes, increase transparency and prioritize high-quality, well-vetted research. By doing this, we can secure the future of AI and uphold the integrity of the scientific community.