Modern Data Engineering Services in 2025: Key Trends Fueling Enterprise-Wide Digital Transformation
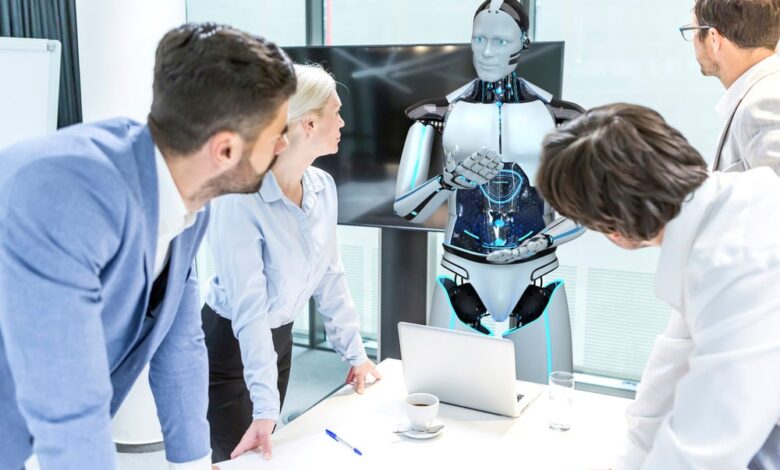
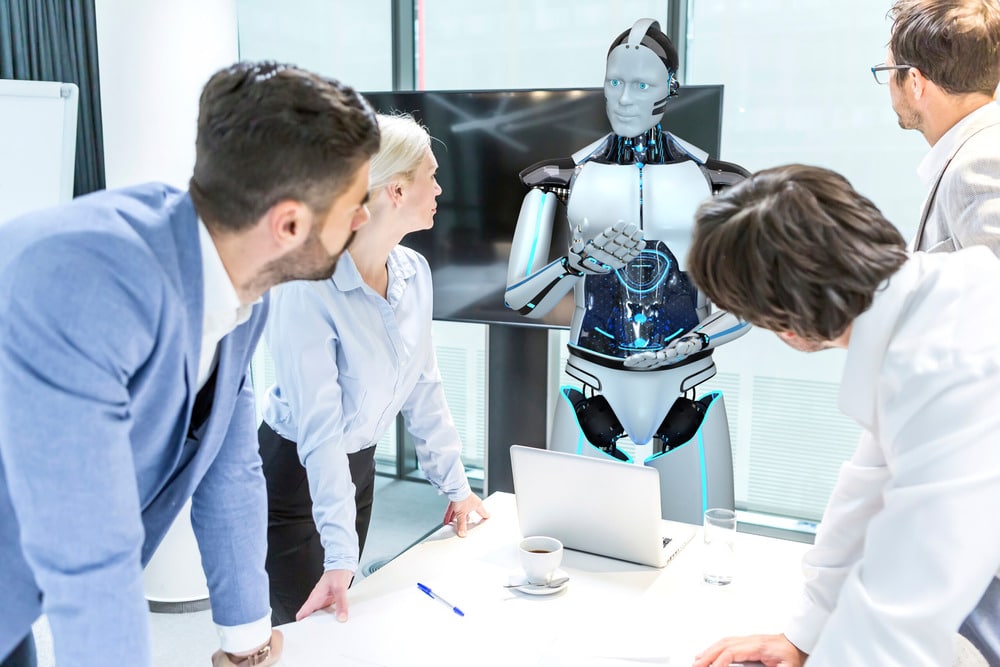
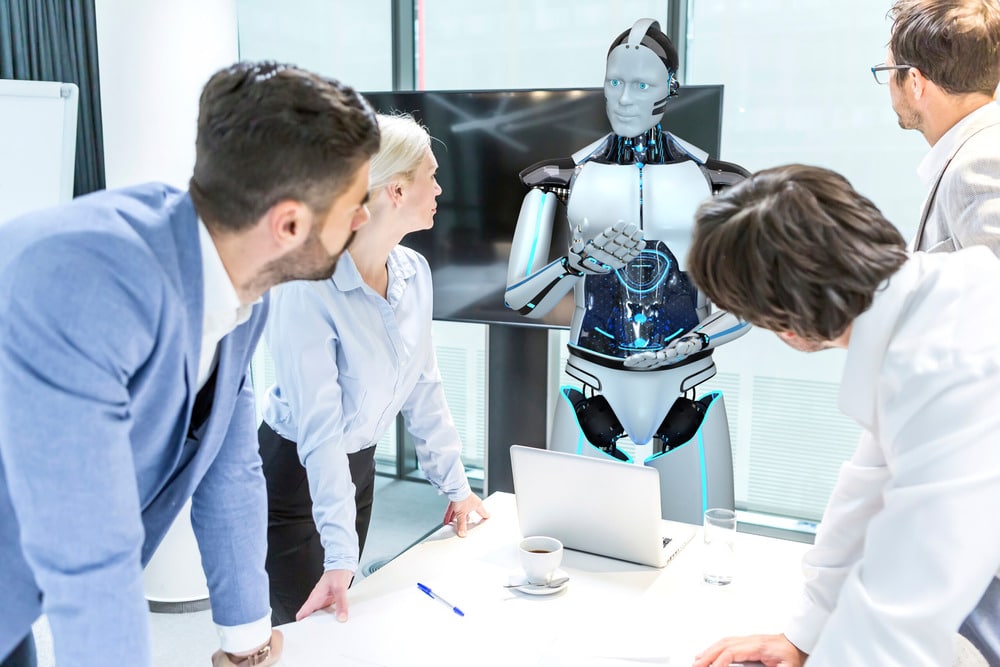
Introduction: The data -driven imperative
In 2025, the digital economy works at an unprecedented speed and complexity and data has become the most valuable currency. Organizations are under pressure to extract useful insights from solid, fast -growing data volumes. The success of digital transformation strategies now depends on the possibility of developing data flows that are scalable, agile and real -time. Modern Data Engineering is no longer only about ETL processes – It is the backbone of Enterprise Intelligence and Innovation.
According to IDC, worldwide data creation is expected to reach 175 seat babytes in 2025, an increase of 64.2 seat babytes in 2020. This explosive growth requires modernized, automated and cloud-centric data technology practices with which organizations can convert data into speed and precision.
The shift to modern data engineering
Traditional Data Engineering focused on structured data movement using rigid batch ETL processes. However, this approach no longer meets the needs of dynamic companies. In 2025, organizations adopt flexible, event-driven data architectures.
Modern Data Engineering now emphasizes:
- Real -time data intake and processing With the help of tools such as Apache Kafka and Apache.
- Modular and scalable architectures Such as the data lakehouse and data mesh.
- Infrastructure as a code (IAC) To automate data pipeline facility.
- Sereless Data Engineering To make cost efficiency and scalability possible.
The transition is about more than tools; It reflects a mentality shift – from moving data to engaging business value via intelligent pipelines.
Top Data Engineering Trends is 2025
Trend #1: Cloud-Native and Hybrid DataLatforms
Companies implement multi-cloud and hybrid data ecosystems to meet compliance, latency and cost objectives. Cloud-Native Dataplatforms such as Snowflake, Bigquery and Azure Synaps make elastic scalability possible, while hybrid setups are in balance with the premium protection with cloud-agility.
A Gartner survey shows that 85% of companies will have a cloud-first strategy by 2025 and data engineering services are central to this shift.
Trend #2: AI/ml-driven automation in pipelines
Manual data transformation and integration are no longer sustainable. Dataing engineers now use machine learning for schedule -detection, anomalo detection and pipeline optimization. AI-driven perceptibility helps to maintain data hubniveness and origin without manual intervention.
Trend #3: Rise of Dataops and Orchestration Tools
Dataops evolves as the devops of the data world. It provides CI/CD in data pipelines with tools such as Apache Airflow, Dagster and Prefect. Data version, rollback and automated tests are now standard in modern pipelines.
Trend #4: Focus on data quality and perceptibility
Inexcuretic data undermine analysis and decision -making. In 2025, companies will increase automated data quality controls, monitoring tools and real -time anomalo detection to guarantee trusted insights.
Trend #5: Democratization via Self-Service Platforms
Data democratization enables non-technical users to explore and visualize data. Self-service analysis tools such as Looker and Power BI are supported by governed semantic layers, giving business teams access to accurate data without trusting it.
Enterprise transformation via Data Engineering
The role of data technology in digital transformation goes much further than IT operations. It stimulates fundamental changes in how companies work and compete.
- Faster decision -making: Real-time dashboards and analyzes enable leadership to act on live insights, reducing the latency in business reactions.
- Personalized customer experiences: Unified Data Pipelines integrate customer behavior, purchasing history and preferences to coordinate services in real time.
- Operational efficiency: Predictive analyzes powered by clean, real -time data can predict the question, optimize supply chains and detect fraud before it influences turnover.
Safety, Compliance and Governance on scale
As data volumes grow, the security and compliance requirements do. Modern Data Engineering includes:
- Roll -based access control (RBAC)
- End-to-end data coding
- Audit paths and origin
- Integration with privacy frameworks such as GDPR and CCPA
In 2025, organizations will close governance in pipelines from the ground, so that each data point is compliant and traceable.
Challenges for data -engineering teams
Despite technological progress, organizations face various challenges:
- Talent deficit: The demand for skilled data senior exceeds the supply. In 2024, LinkedIn mentioned “Data Engineer” in the top 10 of the most common technical roles.
- Complexity of tools: Managing a vast ecosystem of tools and platforms can lead to integration headache and operational overhead.
- Legacy System Integration: Many companies still rely on Legacy systems. Bridging these with modern platforms requires careful planning and hybrid architectures.
Future Outlook: What is the next step for Data Engineering?
Data Engineering is on the way to greater abstraction and autonomy. By 2027 it is expected that more than 60% of data engineering tasks will be automated using generative AI and intelligent orchestration.
Emerging trends include:
- Edge Data Engineering For real -time processing closer to data sources
- Builders with LOW code/NO code Datapijpline builders For faster experiments
- Compound data platforms that adapt to changing business needs without again architecture
The future is one of which data -engineers spend less time on repairing pipelines and more time to make strategic insights possible.
Conclusion: a strategic pillar for digital success
Modern Data Engineering Services Are no longer a back-office function-they are a strategic enabler of enterprise agility, resilience and growth. In 2025, organizations that invest in intelligent, automated and secure data engineering practices will lead the following wave of digital innovation.
The progress of the trip not only requires tools, but a cultural shift to data such as product, pipelines such as code and insights as a competitive advantage. Those who adapt will thrive in the data -driven economy of the future.
Frequently asked questions (frequently asked questions)
1. What is modern data engineering and how does it differ from traditional ETL?
Modern data engineering emphasizes real-time, scalable and modular data processing with the help of tools such as Kafka, airflow and cloud-native platforms, while traditional ETL depends on batch processing and rigid workflows.
2. Why is Data Engineering crucial for digital transformation in 2025?
Data Engineering provides the basis for fast, reliable insights, driving decision -making, personalization, automation and operational efficiency in all business functions.
3. What are the biggest challenges for Data Engineering Teams today?
Important challenges include a shortage of competent talent, the complexity of managing various tool sets, guaranteeing data quality on scale and integrating legacy systems with modern architectures.
4. How does AI workflows improve for engineering?
AI makes intelligent automation possible in pipeline management, anomalia detection, schedule -insertion and perceptibility, reducing manual efforts and improving data reliability.
5. Which trends will define the future of Data Engineering after 2025?
Emerging trends include Edge-based data processing, the rise of low-code data tools, composite architectures and the integration of generative AI for pipeline orchestation and self-healing systems.