Less Is More: Why Retrieving Fewer Documents Can Improve AI Answers
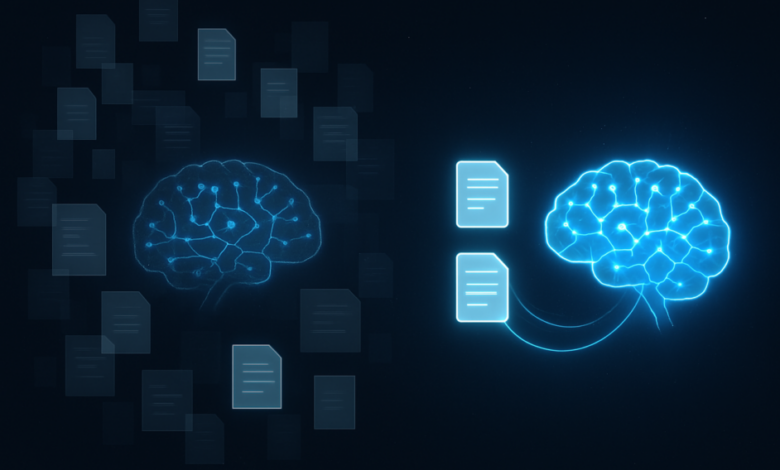
A striking generation (RAG) is an approach for building AI systems that combines a language model with an external source of knowledge. In simple terms, the AI first searches for relevant documents (such as articles or web pages) related to the question of a user and then uses these documents to generate a more accurate answer. This method is celebrated for helping large language models (LLMS) and reducing hallucinations by grounding their answers in real data.
Intuitive you could think that the more documents an AI picks up, the better informed his answer will be. Recent research, however, suggests a surprising turn: when it comes to feeding information to an AI, sometimes is less.
Fewer documents, better answers
A New study Researchers from the Hebrew University of Jerusalem investigated how the number From documents given to a raging system has an impact on his performance. It is crucial that they kept the total amount of text constant – which means that if fewer documents were provided, those documents were somewhat expanded to fill the same length as many documents would do. In this way, all performance differences can be attributed to the amount of documents instead of just having a shorter entry.
The researchers used a question-answering dataset (Musique) with Trivia questions, each originally accompanied by 20 Wikipedia Para cafes (of which only a few contain the answer, where the rest are distractors). By cutting the number of documents from 20 to only the 2-4 really relevant – and filling them with a little extra context to maintain a consistent length – they created scenarios where the AI had fewer pieces of material to consider, but still about the same total words to read.
The results were striking. In most cases, the AI models answered more accurately when they received fewer documents instead of the full set. The performance improved considerably – in some cases up to 10% in accuracy (F1 score) when the system only used the handful of supporting documents instead of a large collection. This counter-intuitive boost was observed in various open-source language models, including variants of Meta’s Llama and others, indicating that the phenomenon is not bound by a single AI model.
One model (QWen-2) was a remarkable exception that handled several documents without a decrease in the score, but almost all tested models performed better with fewer documents in general. In other words, adding more reference material outside the most important relevant pieces actually harm their performance more often than it helped.
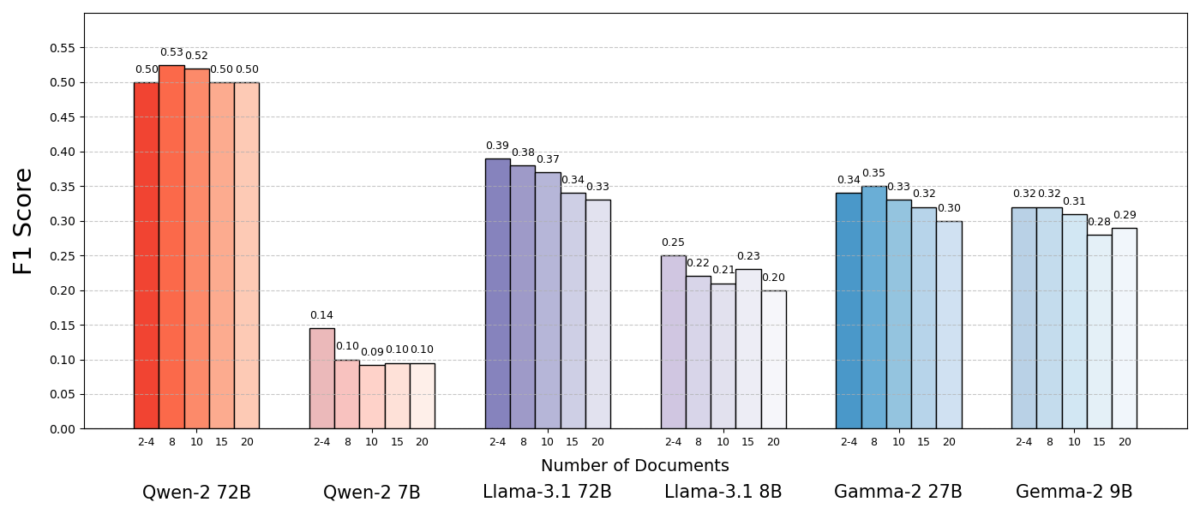
Source: Levy et al.
Why is this such a surprise? Usually rag systems are designed in the assumption that the collection of a wider amount of information can only help the AI - if the answer is not in the first few documents, it can be in the tenth or twentieth.
This study turns that script and shows that without distinction can post on extra documents. Even when the total text length was kept constant, the mere presence of many different documents (each with their own context and peculiarities) made the question answer task more challenging for the AI. It seems that every extra document introduced more noise than signal after a certain point, confusing the model and the ability to extract the correct answer.
Why less in day can be
This “less is more” result is logical as soon as we consider how AI language models process information. When an AI only gets the most relevant documents, the context it sees is focused and free of distractions, just like a student who has received exactly the right pages to study.
In the study, models performed considerably better if they only received the supporting documents, with irrelevant material removed. The remaining context was not only shorter but also cleaner – it contained facts that immediately pointed to the answer and nothing else. With fewer documents to juggle, the model could pay full attention to the relevant information, making it less likely to get on a sideline or confused.
On the other hand, when many documents were picked up, the AI had to search a mix of relevant and irrelevant content. Often these extra documents were “similar but not related” – they can share a subject or keywords with the query, but do not really contain the answer. Such content can mislead the model. The AI can waste effort trying to connect dots in documents that do not actually lead to a correct answer, or even worse, it can incorrectly merge information from multiple sources. This increases the risk of hallucinations – cases where the AI generates an answer that sounds plausible but is not in a single source.
In essence, feeding too many documents to the model can dilute the useful information and introduce conflicting details, making it more difficult for the AI to decide what is true.
Interestingly, the researchers discovered that if the extra documents were clearly irrelevant (for example, random non -related text), the models were better in ignoring them. The real problems come from distracting data that look relevant: when all the texts collected are about similar topics, the AI assumes that it should use them all, and it may have difficulty telling which details are actually important. This is in line with the observation of the study Random distractors caused less confusion than realistic distractors In the input. The AI can filter flagrant nonsense, but subtle off-topic information is a slick fall-hey sneaks under the guise of relevance and derails the answer. By reducing the number of documents to just the really necessary, we avoid these falls in the first place.
There is also a practical advantage: the collection and processing of fewer documents lowers the computational overhead for a rag system. Every document that is attracted must be analyzed (embedded, read and observed by the model), which uses time and computer sources. Eliminating superfluous documents makes the system more efficient – it can find answers faster and at lower costs. In scenarios where accuracy improved by concentrating on fewer sources, we get a win-win: better answers and a slimmer, more efficient process.
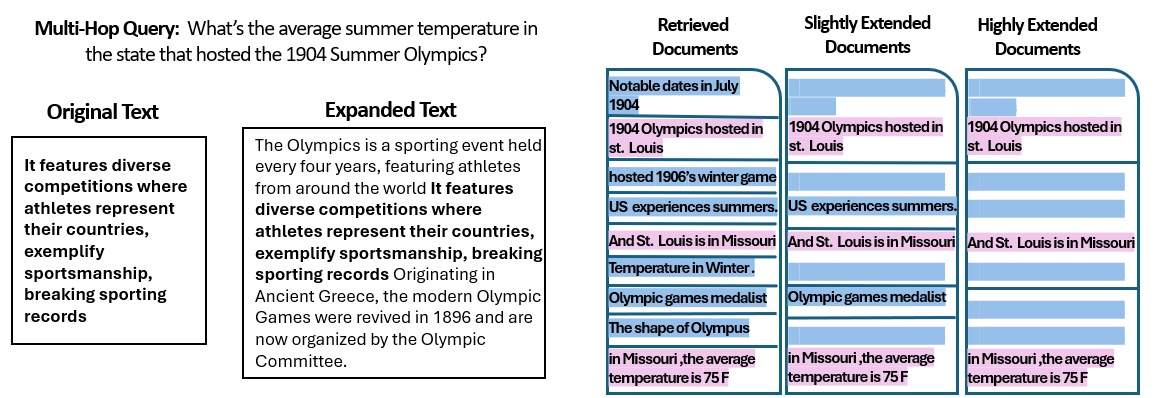
Source: Levy et al.
Rethinking RAG: Future Directions
This new evidence that quality often beats quantity in collecting has important implications for the future of AI systems that depend on external knowledge. It suggests that designers of raging systems must give priority to smart filtering and ranking documents above pure volume. Instead of picking up 100 possible passages and hoping that the answer was buried there somewhere, it can be wiser to only get the top some very relevant.
The authors of the study emphasize the need for collecting methods to “find a balance between relevance and diversity” in the information they provide to a model. In other words, we want to provide sufficient coverage of the subject to answer the question, but not so much that the nuclear facts are drowned in a sea of external text.
In the future, researchers will probably investigate techniques that help AI models to process multiple documents more gracefully. An approach is to develop better Retriever systems or recovery plates that can identify which documents really add value and which only introduce conflicts. Another perspective is to improve the language models themselves: if a model (such as QWen-2) managed to cope with many documents without losing the accuracy, investigating how it was trained or structured could offer other models to make other models. Perhaps future large language models mechanisms will include to recognize when two sources say the same (or contradict each other) and focus accordingly. The aim would be to enable models to use a rich variety of sources without falling prey to confusion – effectively getting the best out of two worlds (width of information and clarity of focus).
It is also worth noting that as AI systems get larger context windows (the possibility to read more text at the same time), simply more data in the prompt dumping is not a silver bullet. Larger context does not automatically mean a better understanding. This study shows that, even if an AI can read 50 pages at the same time, which gives 50 pages of information from mixed quality, may not result in a good result. The model still benefits from composite, relevant content to work with, instead of any dump. Intelligent collection can become even more important in the era of gigantic context windows – to ensure that the extra capacity is used for valuable knowledge instead of noise.
The findings of “More documents, the same length” (The appropriate title Paper) courageous a re-examination of our assumptions in AI research. Sometimes feeding an AI is all the data that we have not as effective as we think. By concentrating on the most relevant pieces of information, we not only improve the accuracy of answers generated by AI, but also make the systems more efficient and easier to trust. It is a counter -intuitive lesson, but one with exciting consequences: future RAG systems can be both smarter and slimmer by carefully choosing less, better documents to pick up.