How Amazon is Redefining the AI Hardware Market with its Trainium Chips and Ultraservers
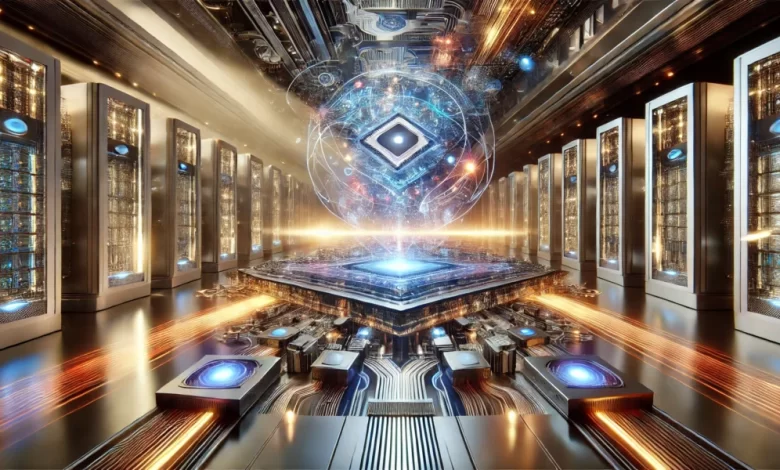
Artificial intelligence (AI) is one of the most exciting technological developments of our time. It’s changing the way industries work, from improving healthcare with more innovative diagnostic tools to personalizing shopping experiences in e-commerce. But what is often overlooked in the AI debates is the hardware behind these innovations. Powerful, efficient, and scalable hardware is essential to supporting the massive computing needs of AI.
Amazonknown for cloud services via AWS and its dominance in e-commerce, is making significant strides in the AI hardware market. With are custom designed Trainium chips and advanced Ultra serversAmazon does more than just provide the cloud infrastructure for AI. Instead, it creates the very hardware that fuels its rapid growth. Innovations like Trainium and Ultraservers are setting a new standard for AI performance, efficiency and scalability and are changing the way companies approach AI technology.
The evolution of AI hardware
The rapid growth of AI is closely linked to the evolution of hardware. Early on, AI researchers relied on general-purpose processors like CPUs for basic machine learning tasks. However, these processors, designed for general purpose computing, were not suited to the demanding demands of AI. As AI models became more complex, CPUs struggled to keep up. AI tasks require massive processing power, parallel computations, and high data throughput, which were significant challenges that CPUs could not handle effectively.
The first breakthrough came with Graphics Processing Units (GPUs), originally designed for video game graphics. Because they could perform many calculations simultaneously, GPUs proved ideal for training AI models. This parallel architecture made GPUs suitable hardware for deep learning and accelerated AI development.
However, GPUs also began to show limitations as AI models became larger and more complex. They were not explicitly designed for AI tasks and often lacked the energy efficiency required for large-scale AI models. This led to the development of specialized AI chips built explicitly for machine learning workloads. Companies like Google introduced Tensor Processing Units (TPUs)as Amazon developed Inference for inference tasks and Trainium for training AI models.
Trainium represents a significant advancement in AI hardware. It is purpose-built to meet the intensive demands of training large-scale AI models. In addition to Trainium, Amazon introduced Ultraservers, high-performance servers optimized for running AI workloads. Trainium and Ultraservers are reshaping AI hardware and providing a solid foundation for the next generation of AI applications.
Trainium chips from Amazon
Amazon’s Trainium chips are custom-designed processors built to handle the compute-intensive task of training large-scale AI models. AI training involves processing large amounts of data through a model and adjusting the parameters based on the results. This requires enormous computing power, often spread over hundreds or thousands of machines. Trainium chips are designed to meet this need, providing exceptional performance and efficiency for AI training workloads.
The power of the first generation of AWS Trainium chips Amazon EC2 Trn1 instances, with up to 50% lower training costs than other EC2 instances. Designed for AI workloads, these chips deliver high performance while reducing operational costs. Amazon’s Trainium2, its second-generation chip, goes one step further, offering up to four times the performance of its predecessor. Trn2 instances, optimized for generative AI, deliver up to 30-40% better price-performance than current generation GPU-based EC2 instances, such as the P5e and P5en.
Trainium’s architecture allows it to deliver substantial performance improvements for demanding AI tasks, such as training large language models (LLMs) and multimodal AI applications. For example, Trn2 UltraServers, which combine multiple Trn2 instances, can achieve up to 83.2 petaflops of FP8 compute power, 6 TB of HBM3 memory, and 185 terabytes per second of memory bandwidth. These performance levels are ideal for core AI models that require more memory and bandwidth than traditional server instances can provide.
In addition to raw performance, energy efficiency is a key advantage of Trainium chips. Trn2 instances are designed to be three times more energy efficient than Trn1 instances, which were already 25% more energy efficient than comparable GPU-powered EC2 instances. This improvement in energy efficiency is significant for companies focusing on sustainability as they scale their AI operations. Trainium chips significantly reduce energy consumption per training operation, helping companies reduce costs and environmental impact.
Integration of Trainium chips with AWS services such as Amazon SageMaker And AWS neuron provides an effective experience for building, training, and deploying AI models. This end-to-end solution allows companies to focus on AI innovation rather than infrastructure management, making it easier to accelerate model development.
Trainium is already used in all sectors. Companies like it Databricks, Ricoh and MoneyForward use Trn1 and Trn2 instances to build robust AI applications. These instances help organizations reduce their total cost of ownership (TCO) and accelerate model training time, making AI more accessible and efficient at scale.
Ultra servers from Amazon
Amazon’s Ultraservers provide the infrastructure needed to run and scale AI models, complementing the computing power of Trainium chips. Designed for both training and inference phases of AI workflows, Ultraservers provides a powerful, flexible solution for businesses that need speed and scalability.
The Ultraserver infrastructure is built to meet the growing demands of AI applications. Its focus on low latency, high bandwidth and scalability makes it ideal for complex AI tasks. Ultra servers can process multiple AI models simultaneously and ensure that the workload is distributed efficiently across the servers. This makes them perfect for companies that need to deploy AI models at scale, whether for real-time applications or batch processing.
An important advantage of Ultraservers is their scalability. AI models require massive computing resources, and Ultraservers can quickly scale up or down resources based on demand. This flexibility helps companies manage costs effectively, while still having the ability to train and deploy AI models. According to Amazon, Ultraservers significantly improve processing speeds for AI workloads, providing better performance compared to previous server models.
Ultraservers integrates effectively with Amazon’s AWS platform, allowing companies to take advantage of AWS’s global network of data centers. This gives them the flexibility to deploy AI models across multiple regions with minimal latency, which is especially useful for organizations with global operations or those handling sensitive data that requires local processing.
Ultraservers have real-world applications in several industries. In healthcare, they could support AI models that process complex medical data, helping with diagnostics and personalized treatment plans. In autonomous driving, Ultraservers can play a crucial role in scaling machine learning models to process the massive amounts of real-time data generated by self-driving vehicles. Their high performance and scalability make them ideal for any industry that requires fast, large-scale data processing.
Market impact and future trends
Amazon’s move into the AI hardware market with Trainium chips and Ultraservers is an important development. By creating custom AI hardware, Amazon is emerging as a leader in AI infrastructure. The strategy aims to provide companies with an integrated solution for building, training and deploying AI models. This approach offers scalability and efficiency, giving Amazon an edge over competitors like Nvidia and Google.
One of Amazon’s key strengths is its ability to integrate Trainium and Ultraservers with the AWS ecosystem. This integration allows companies to use AWS cloud infrastructure for AI operations without the need for complex hardware management. The combination of Trainium’s performance and AWS’s scalability helps companies train and deploy AI models faster and more cost-effectively.
Amazon’s entry into the AI hardware market is changing the discipline again. With purpose-built solutions like Trainium and Ultraservers, Amazon is becoming a strong competitor to Nvidia, which has long dominated the AI GPU market. Trainium is specifically designed to meet the growing needs of AI model training, providing cost-effective solutions for businesses.
AI hardware is expected to grow as AI models become more complex. Specialized chips such as Trainium will play an increasingly important role. Future hardware developments will likely focus on improving performance, energy efficiency and affordability. Emerging technologies such as quantum computing could also shape the next generation of AI tools, enabling even more robust applications. The future looks promising for Amazon. The focus on Trainium and Ultraservers drives innovation in AI hardware and helps companies maximize the potential of AI technology.
The bottom line
Amazon is redefining the AI hardware market with its Trainium chips and Ultraservers, setting new standards in performance, scalability and efficiency. These innovations go beyond traditional hardware solutions and provide companies with the tools needed to tackle the challenges of modern AI workloads.
By integrating Trainium and Ultraservers with the AWS ecosystem, Amazon provides a comprehensive solution for building, training and deploying AI models, making it easier for organizations to innovate.
The impact of these developments extends across sectors, from healthcare to autonomous driving and beyond. With the energy efficiency of Trainium and the scalability of Ultraservers, companies can reduce costs, improve sustainability and deal with increasingly complex AI models.