How AI Solves the ‘Cocktail Party Problem’ and Its Impact on Future Audio Technologies
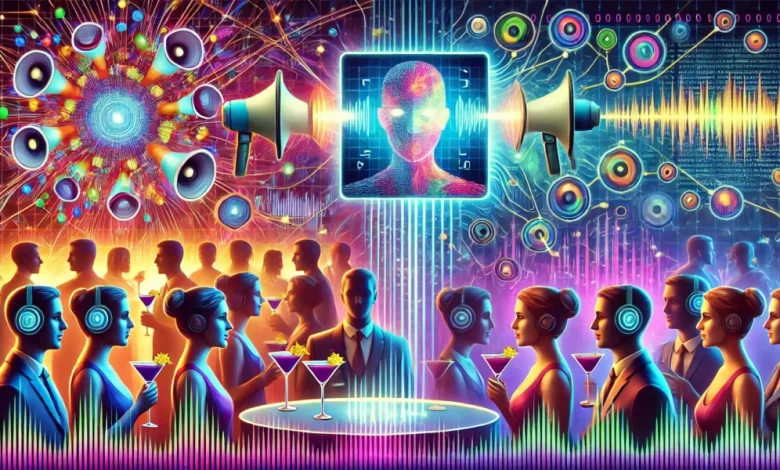
Imagine being at a busy event, surrounded by voices and background noise, and yet you manage to focus on the conversation with the person right in front of you. This ability to isolate a specific sound amidst the noisy background is known as the Cocktail party problema term first coined by British scientist Colin Cherry in 1958 to describe this remarkable ability of the human brain. AI experts have been striving to imitate this human ability with machines for decades, but it remains a daunting task. However, recent developments in artificial intelligence are groundbreaking and provide effective solutions to the problem. This sets the stage for a transformative shift in audio technology. In this article, we explore how AI is making progress in tackling the Cocktail Party problem and the potential it holds for future audio technologies. Before we delve into how AI usually solves this problem, let’s first understand how humans solve the problem.
How people decode the cocktail party problem
Humans have a unique auditory system that helps us navigate noisy environments. Our brains process sounds binaurally, meaning we use input from both ears to detect small differences in timing and volume, allowing us to detect the location of sounds. This ability allows us to orient ourselves to the voice we want to hear, even as other sounds compete for attention.
In addition to hearing, our cognitive skills enhance this process even more. Selective attention helps us filter out irrelevant sounds, allowing us to focus on important information. Meanwhile, context, memory and visual cues, such as lip reading, help separate speech from background noise. This complex sensory and cognitive processing system is incredibly efficient, but replicating it in machine intelligence remains difficult.
Why it remains a challenge for AI?
From virtual assistants that recognize our commands in a busy cafe to hearing aids that help users focus on a single conversation, AI researchers have continuously worked to replicate the human brain’s ability to solve the Cocktail Party Problem. This search has led to the development of techniques such as blind source separation (BSS) And Independent Component Analysis (ICA)designed to identify and isolate different sound sources for individual processing. While these methods have shown promise in controlled environments – where sound sources are predictable and do not overlap significantly in frequency – they have difficulty differentiating overlapping voices or isolating a single sound source in real time, especially in dynamic and unpredictable environments. This is mainly due to the lack of the sensory and contextual depth that humans naturally utilize. Without additional cues such as visual cues or familiarity with specific tones, AI faces challenges in managing the complex, chaotic mix of sounds encountered in everyday environments.
How WaveSciences used AI to solve the problem
In 2019, WaveSciencesa US-based company founded by electrical engineer Keith McElveen in 2009, made a breakthrough in tackling the cocktail party problem. Their solution, Spatial Release from Masking (SRM), uses AI and the physics of sound propagation to isolate a speaker’s voice from background noise. While the human auditory system processes sound from different directions, SRM uses multiple microphones to capture sound waves as they travel through space.
One of the crucial challenges in this process is that sound waves are constantly bouncing around and mixing in the environment, making it difficult to mathematically isolate specific voices. However, using AI, WaveSciences has developed a method to identify the origin of any sound and filter out background noise and ambient voices based on their spatial location. This adaptability allows SRM to deal with changes in real time, such as a moving speaker or the introduction of new sounds, making it significantly more effective than previous methods that struggled with the unpredictable nature of real-world audio settings. These advancements not only improve the ability to focus on conversations in noisy environments, but also pave the way for future innovations in audio technology.
Advances in AI techniques
Recent advances in artificial intelligence, especially in deep neural networkshas significantly improved the ability of machines to solve cocktail party problems. Deep learning algorithms, trained on large datasets of mixed audio signals, excel at identifying and separating different sound sources, even in overlapping voice scenarios. Projects like BioCPPNet have successfully demonstrated the effectiveness of these methods by isolating animal vocalizations, indicating their applicability in various biological contexts beyond human speech. Researchers have shown that deep learning techniques can adapt voice separation learned in musical environments to new situations, improving the robustness of the model in different environments.
Neural bundle formation further enhances these capabilities by using multiple microphones to focus on sounds from specific directions while minimizing background noise. This technique is refined by dynamically adjusting focus based on the audio environment. Moreover, AI models use time-frequency masking to distinguish audio sources based on their unique spectral and temporal characteristics. Advanced diary writing speaker systems isolate voices and track individual speakers, facilitating organized conversations. AI can more accurately isolate and enhance specific voices by recording visual cues such as lip movements in addition to audio data.
Real-world applications of the cocktail party problem
These developments have opened new avenues for the advancement of audio technologies. Some real world applications include the following:
- Forensic analysis: According to one BBC reportSpeech Recognition and Manipulation (SRM) technology is used in courtrooms to analyze audio evidence, especially in cases where background noise complicates the identification of speakers and their dialogue. Often recordings become useless as evidence in such scenarios. However, SRM has proven invaluable in forensic contexts, successfully decoding critical audio for presentation in court.
- Noise Canceling Headphones: Researchers have developed a prototype AI system called Hearing target speech for noise canceling headphones that allow users to select a specific person’s voice to remain audible and cancel out other sounds. The system uses cocktail party problem-based techniques to run efficiently on headsets with limited computing power. It’s currently a proof-of-concept, but the makers are in talks with headphone brands to potentially integrate the technology.
- Hearing aids: Modern hearing aids often struggle in noisy environments because they fail to isolate specific voices from background noise. While these devices can amplify sound, they lack the sophisticated filtering mechanisms that allow human ears to focus on a single conversation amid competing sounds. This limitation is especially challenging in busy or dynamic environments, where overlapping voices and fluctuating sound levels are prevalent. Solutions to the cocktail party problem can improve hearing aids by isolating desired voices and minimizing ambient noise.
- Telecommunications: In telecommunications, AI can improve call quality by filtering out background noise and emphasizing the speaker’s voice. This leads to clearer and more reliable communications, especially in noisy environments such as busy streets or busy offices.
- Voice assistants: AI-powered voice assistants, such as Amazon’s Alexa and Apple’s Siri, can become more effective in noisy environments and solve cocktail party problems more efficiently. These improvements allow devices to accurately understand and respond to user commands, even during background noise.
- Record and edit audio: AI-powered technologies can help audio engineers in post-production by isolating individual sound sources in recorded material. This capability allows for cleaner tracks and more efficient editing.
The bottom line
The Cocktail Party Problem, a key challenge in audio processing, has made remarkable progress thanks to AI technologies. Innovations such as Spatial Release from Masking (SRM) and deep learning algorithms are redefining the way machines isolate and separate sounds in noisy environments. These breakthroughs improve everyday experiences, including clearer conversations in busy environments and improved functionality for hearing aids and voice assistants. Yet they also have transformative potential for applications in forensic analysis, telecommunications and audio production. As AI continues to evolve, its ability to mimic human auditory capabilities will lead to even more significant improvements in audio technologies, ultimately reshaping the way we interact with sound in our daily lives.