Google’s New AI “Co-Scientist” Aims to Accelerate Scientific Discovery
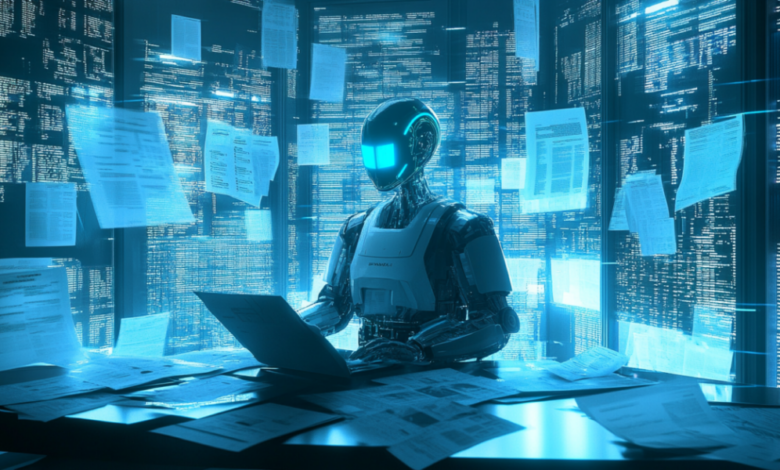
Imagine a research partner who has read every scientific article that you have, without brainstorming the clock on new experiments. Google actually tries to convert this vision with a new AI system that is designed to act as a ‘co-scientist’.
This AI-driven assistant can search huge libraries of research, propose new hypotheses and even experimenting plans out of it all in collaboration with human researchers. The latest tool from Google, tested at Stanford University and Imperial College London, uses advanced reasoning to help scientists synthesize mountains of literature and to generate new ideas. The aim is to speed up scientific breakthroughs by giving information overload and to suggest insights that a person could miss.
This “AI co-scientist“As Google calls it, it is not a physical robot in a laboratory, but an advanced software system. It is built on the latest AI models from Google (especially the Gemini 2.0 model) And reflects the way scientists think – from brainstorming to criticism of ideas. Instead of just summarizing known facts or searching for articles, the system is intended to discover the original knowledge and to propose really new hypotheses based on existing evidence. In other words, it not only finds answers to questions – it helps to find new questions to ask.
Google and his AI unit Deepen have given priority to science applications for AI, after demonstrating successes such as AlfafoldThat AI used to solve the 50-year-old puzzle of protein folds. With the AI-CO scientist, they hope to accelerate “the clock speed” of discoveries in fields from biomedicin to physics.
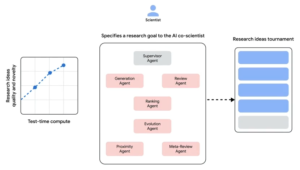
AI Co-Scientist (Google)
How an AI co-scientist works
Under the hood, Google’s AI scientist actually consists of several specialized AI programs together as a team of SuperFast research assistants, each with a specific role. This AI agents Work together in a pipeline that mimics the scientific method: people generate ideas, others criticize and refine them, and the best ideas are forwarded to the human scientist.
According to the Google research team, here is how the process unfolds:
- Generation officer – Mine relevant research and synthesizes existing findings to propose new ways or hypotheses.
- Reflection agent – acts as a peer reviewer, checking the accuracy, quality and novelty of the proposed hypotheses and removing poor ideas.
- Order – performs a “tournament” of ideas, so that the hypotheses actually compete in simulated debates, and then ranks them based on which the most promising corpses.
- Proximity – Groups of comparable hypotheses together and eliminates duplicates, so that the researcher does not assess repetitive ideas.
- Evolution -takes the best -arranged hypotheses and further refines them, using analogies or simplification of concepts for clarity to improve the proposals.
- Meta-review agent – Finally the best ideas put together in a coherent research proposal or overview for the human scientist to assess.
It is crucial that the human scientist stays in the loop in every phase. The co-scientist of AI does not work separately or makes definitive decisions in itself. Researchers start feeding in a research goal or demand in the natural language – for example a goal to find new strategies to treat a certain disease – together with any relevant limitations or first ideas they have. The AI system then goes through the above cycle to produce suggestions. The scientist can give feedback or adjust parameters, and the AI will repeat again.
Google built the system to ‘be specially built for cooperation’, which means that scientists can insert their own seed ideas or criticisms during the AI process. The AI can even search for external tools such as web search and use other specialized models to check facts or collect data while it works, so that the hypotheses are based on up-to-date information.
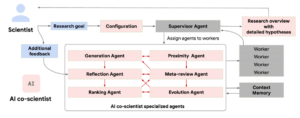
AI Co-science agents (Google)
A faster path to breakthroughs
By outsourcing a part of the drudge work of research – exhausting literature reviews and first brainstorming – to a non -repellent machine, scientists hope to speed up the discovery dramatically. The co-scientist of the AI can read much more articles than any person, and it never comes out of new combinations of ideas to try.
“It has the potential to speed up scientists’ efforts to take on major challenges in science and medicine,” wrote in the newspaper. Early results are encouraging. In one study aimed at liver fibrosis (scars of the liver), Google reported that every approach that suggested the AI-CO scientist showed promising capacity to inhibit drivers of the disease. In fact, the recommendations of the AI in that experiment were not recordings in the dark – they were in line with which experts considered plausible interventions.
Moreover, the system showed a capacity to improve over time with people investigated by people. According to Google, the AI continued to refine and optimize solutions that experts had initially proposed, which indicates that it can learn and add incremental value outside of human expertise with every iteration.
Another remarkable test was the thorny problem of antibiotic resistance. Researchers did the AI on the assignment to explain how a certain genetic element bacteria helps to spread their drug -resistant properties. Without the knowledge of the AI, a separate scientific team (in a not yet published study) had already discovered the mechanism. The AI only received simple background information and a few relevant articles and left to its own devices. Within two days it came to the same hypothesis that the human scientists had.
“This finding was experimentally validated in the independent research study, which was unknown to the co-scientist while generating hypotheses,” the authors noted. In other words, the AI succeeded in rediscovering an important insight in itself, which shows that it can connect dots in a way that can raise human intuition – at least in cases where there are sufficient data.
The implications of such speed and cross-disciplinary reach are enormous. Breakthroughs often happen when insights from different fields collide, but no person can be an expert in everything. An AI who has absorbed knowledge in genetics, chemistry, medicine and more, could imagine ideas that human specialists can overlook. The DeepMind unit of Google has already proven how transforming AI can be in science with Alpafold, who predicted the 3D structures of proteins and was praised as a big leap forward for biology. That performance, which accelerates the discovery of drugs and vaccine development, even earned the DeepMind team part of the highest distinctions of science (including recognition linked to the Nobel Prize).
The new AI-CO scientist wants to bring comparable jumps to the daily brainstorm of research. Although the first applications have been in biomedicin, the system can in principle be applied to any scientific domain of physics to environmental sciences-to the method for generating and checking hypotheses discipline agent. Researchers can use it to hunt new materials, explore climate solutions or discover new mathematical statements. In any case, the promise is the same: a faster path from demand to insight, which may compress years of trial-and-arror in a much shorter time frame.