From Lab to Market: Why Cutting-Edge AI Models Are Not Reaching Businesses
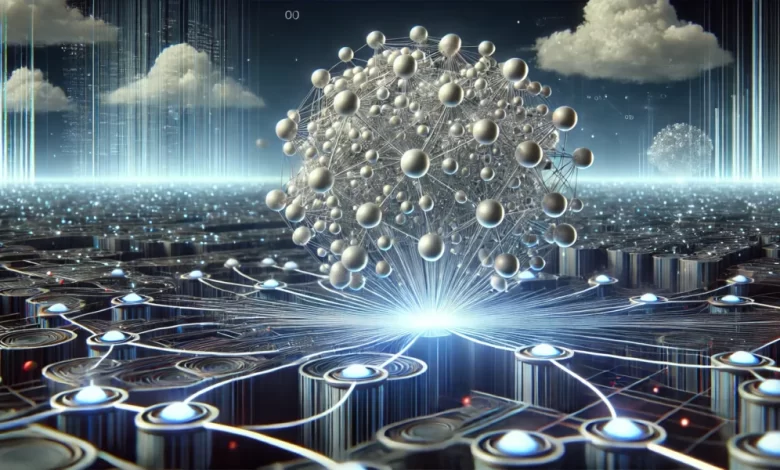
Artificial intelligence (AI) is no longer just a science fiction concept. It is now a technology that has transformed human life and has the potential to reform many industries. AI can change many disciplines, from chatbots that help customer service to advanced systems that accurately diagnose diseases. But even with these important achievements, many companies find the use of AI hard in their daily activities.
While researchers and technology companies AI are making progress, many companies are struggling to keep up. Challenges such as the complexity of integrating AI, the shortage of skilled employees and high costs even make it difficult for the most advanced technologies to be effectively assumed. This gap between making AI and using is not just a missed opportunity; It is a major challenge for companies that try to stay competitive in today’s digital world.
Insight into the reasons behind this gap, identifying the barriers that prevent companies from fully using AI, and finding practical solutions are essential steps to make AI a powerful tool for growth and efficiency in various industries.
Insight into AI’s rapid growth and non -realized potential
AI has reached remarkable technological milestones in the past decade. For example, OpenAi’s GPT models have demonstrated the transforming power of generative AI in areas such as making content, customer service and education. These systems have enabled machines to communicate almost as effectively as people, so that new possibilities are brought into the way companies deal with their audience. At the same time, the progress in Computer Vision has brought innovations into autonomous vehicles, medical imaging and security, so that machines with precision can process and respond to visual data.
AI is no longer limited to niche applications or experimental projects. From the beginning of 2025, global investments in AI are expected to reach an impressive $ 150 billionCompletion of a widespread belief in his ability to bring innovation to different industries. For example, AI-driven chatbots and virtual assistants transform customer service by treating questions efficiently, reducing the burden for human agents and improving the overall user experience. AI is crucial in saving lives by making early disease detection, personalized treatment plans possible and even helping with robot operations. Retailers use AI to optimize supply chains, predict customer preferences and create personalized store experiences that keep customers involved.
Despite this promising progress, such success stories remain the exception than the norm. While large companies like it Amazon have successfully used AI to optimize logistics and Netflix Recommendations Recommendations via advanced algorithms, many companies still have trouble going beyond pilot projects. Challenges such as limited scalability, fragmented data systems and a lack of clarity about the implementation of AI effectively prevent many organizations from realizing its full potential.
A recent study shows that 98.4% Organizations intend to increase their investment in AI and data -driven strategies in 2025. However, around 76.1% of most companies are still in the test or experimental phase of AI technologies. This gap emphasizes the challenges of companies in translating AI’s groundbreaking opportunities into practical, real-world applications.
As companies work on creating a culture driven by AI, they focus more on overcoming challenges such as resistance to change and shortages of competent talent. Although many organizations see positive results of their AI efforts, such as better customer acquisition, improved retention and increased productivity, the greater challenge is to find out how AI can be scaled up effectively and overcome the obstacles. This emphasizes that only investing in AI is not sufficient. Companies must also build strong leadership, good governance and supporting culture to ensure that their AI investments deliver value.
Obstacles that prevent AI adoption
The adoption of AI comes with its own challenges, so that companies often prevent companies from realizing the full potential. These obstacles are challenging, but require targeted efforts and strategic planning to overcome.
One of the biggest obstacles is the lack of trained professionals. Implementing AI successfully requires expertise in data science, machine learning and software development. In 2023, more than 40% of companies identified the talent shortage as an important barrier. Smaller organizations in particular struggle due to limited resources to hire or invest experts in training their teams. To bridge this gap, companies must give priority to updating their employees and promoting partnerships with academic institutions.
Costs are another major challenge. The required investment for AI for AI acceptance, including the acquisition of technology, building infrastructure and training of employees – can be enormous. Many companies hesitate to take the steps without precise projections from ROI. For example, an e-commerce platform can see the potential of an AI-driven recommendation system to stimulate sales, but find the initial costs unaffordable. Pilot projects and phased implementation strategies can provide tangible evidence of the benefits of AI and help reduce the perceived financial risks.
Managing data comes with his own series of challenges. AI models perform well with high-quality, well-organized data. Yet many companies are struggling with problems such as incomplete data, systems that do not communicate well with each other, and strict privacy laws such as GDPR and CCPA. Poor data management can lead to unreliable AI results, which reduces trust in these systems. For example, a healthcare provider can make it difficult to combine radiology data with the history of the patient due to incompatible systems, making AI-driven diagnostics less effective. That is why investing in strong data infrastructure ensures that AI performs reliably.
Moreover, the complexity of the use of AI in real-world institutions is considerable obstacles. Many AI solutions excel in controlled environments, but struggle with scalability and reliability in dynamic, real-world scenarios. For example, predictive maintenance AI can perform well in simulations, but stands for challenges in integrating with existing production systems. Ensuring robust tests and the development of scalable architectures are crucial for bridging this gap.
Resistance to change is another challenge that often disrupts AI acceptance. Employees can fear for job displacement and leadership can hesitate to revise established processes. Moreover, the lack of coordination between AI initiatives and general business objectives often leads to overwhelming results. Implementing an AI chatbot without integrating it into a broader customer service strategy can lead to inefficiencies instead of improvements. To succeed, companies need clear communication about the role of AI, coordination with goals and a culture that embraces innovation.
Ethical and regulatory barriers also slow down the AI acceptance. Concern about data privacy, bias in AI models and accountability for automated decisions create hesitation, in particular in industries such as finance and healthcare. Companies must develop rules while building trust through transparency and responsible AI practices.
Technical barriers for adoption
Hungoing AI models often require significant calculation sources, including specialized hardware and scalable cloud solutions. These technical requirements can be priceless for smaller companies. While cloud -based platforms such as Microsoft Azure And Google AI Provide scalable options, their costs remain a challenge for many organizations.
In addition, controversial malfunctions such as Amazon’s biased recruitment tooldeleted after preferred male candidates to female applicants, and Microsoft’s Tay Chatbotwho quickly started posting offensive content, have eroded faith in AI technologies. IBM Watson for oncology Also confronted with criticism when it was revealed that it did unsafe treatment recommendations because of a trained on a limited dataset. These incidents have emphasized the risks related to AI deployment and have contributed to a growing skepticism among companies.
Finally, the willingness of the market to adopt advanced AI solutions can be a limiting factor. Infrastructure, consciousness and trust in AI are not uniformly divided over industries, making the adoption in some sectors slower. To tackle this, companies must conduct education campaigns and collaborate with stakeholders to demonstrate the tangible value of AI.
Bridging the gap: strategies for successful AI integration
The integration of AI into companies requires a well -considered approach that tunes technology to organizational strategy and culture. The following guidelines outline important strategies for successful AI integration:
- Define a clear strategy: Successful AI acceptance starts with identifying specific challenges that AI can tackle, to set measurable goals and to develop a phased route map for implementation. Starting Small with pilot projects helps to test the feasibility and prove the value of AI before scaling up.
- Start with pilot projects: The implementation of AI on a small scale enables companies to evaluate its potential in a controlled environment. These initial projects offer valuable insights, build up the trust of stakeholders and refine approaches for a broader application.
- Promote innovation: Encouragement of experiments through initiatives such as hackathons, innovation labs or academic collaborations promotes creativity and trust in the possibilities of AI. Building an innovative culture ensures that employees are enabled to explore new solutions and embrace AI as a tool for growth.
- Invest in the development of staff: Bridging the skill gap is essential for effective AI integration. Providing extensive training programs is employees the technical and management skills needed to work together with AI systems. Upskilling teams ensure readyness and improve cooperation between people and technology.
AI can transform the industry, but achieving this requires a proactive and strategic approach. By following these guidelines, organizations can effectively bridge the gap between innovation and practical implementation, so that the full potential of AI is unlocked.
The Bottom Line
AI has the potential to define industries, to resolve complex challenges and improve lives in in -depth ways. However, its value is realized when organizations carefully integrate and close it with their goals. Success with AI requires more than just technological expertise. It depends on promoting innovation, authorizing employees with the right skills and building trust in their possibilities.
Although challenges such as high costs, data fragmentation and resistance to change may seem overwhelming, they are opportunities for growth and progress. By tackling these barriers with strategic action and a dedication to innovation, companies can turn AI into a powerful tool for transformation.