DeepSeek-Prover-V2: Bridging the Gap Between Informal and Formal Mathematical Reasoning
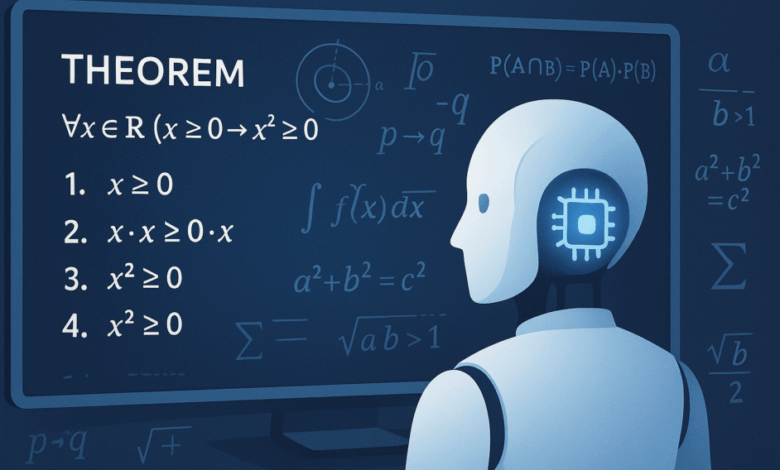
Although Deepseek-R1 has considerably advanced the possibilities of AI in informal reasoning, formal mathematical reasoning has remained a challenging task for AI. This is mainly because producing verifiable mathematical evidence requires both deep conceptual understanding and the ability to construct precise, step -by -step logical arguments. However, significant progress is recently made in this direction, since researchers have introduced at Deepsek-AI Deepseek-Prover-V2An open-source AI model that is able to transform mathematical intuition into rigorous, verifiable evidence. This article will delve into the details of Deepseek-Prover-V2 and consider his potential impact on future scientific discovery.
The challenge of formal mathematical reasoning
Mathematicians often solve problems with the help of intuition, heuristics and high -level reasoning. With this approach they can skip steps that seem obvious or depend on approaches that are sufficient for their needs. Formal statement, however, proves a different approach. It requires complete precision, explicitly stated with each step and logically justified without any ambiguity.
Recent developments in large language models (LLMS) have shown that they can tackle complex mathematical problems at competition level with the help of natural language reasoning. Despite these progress, however, LLMS still has difficulty converting intuitive reasoning into formal evidence that machines can verify. It is mainly because informal reasoning often includes shortcuts and omitted steps that formal systems cannot verify.
Deepseek-Prover-V2 is tackling this problem by combining the strengths of informal and formal reasoning. It breaks complex problems in smaller, manageable parts, while still retaining the precision required by formal verification. This approach makes it easier to bridge the gap between human intuition and machine-oriented evidence.
A new approach to theorem proves
Deepseek Prover-V2 uses a unique pipeline for data processing that entails both informal and formal reasoning. The pipeline starts with Deepseek-V3, a general LLM, which analyzes mathematical problems in the natural language, dissects them in smaller steps and translates those steps into formal language that machines can understand.
Instead of trying to solve the whole problem at the same time, the system breaks it up in a series of “subgoals” – intermediate lemmas that serve as stepping stones in the direction of the final proof. This approach replicates how human mathematicians tackle difficult problems by working by manageable chunks instead of resolving everything in one go.
What makes this approach particularly innovative is how the training data synthesizes. When all subgoals of a complex problem are successfully solved, the system combines these solutions in complete formal evidence. This evidence is then linked to the original reasoning of Deepseek-V3’s original chain of Debit to create high-quality “cold-start” training data for model training.
Learning reinforcement for mathematical reasoning
After the initial training on synthetic data, Deepseek-Prover-V2 uses reinforcement learning to further improve their possibilities. The model receives feedback on whether its solutions are correct or not, and it uses this feedback to learn which approaches work best.
One of the challenges here is that the structure of the generated evidence is not always in line with lemma decoration suggested by the Debit thought out. To resolve this, the researchers recorded a consistency show in the training phases to reduce structural incorrect alignment and to enforce the inclusion of all dissolved lemmas in definitive evidence. This coordination approach has proved particularly effective for complex statements that require multi-step reasoning.
Performance and Real-World options
The performance of Deepseek-Prover-V2 on established benchmarks shows its exceptional possibilities. The model achieves impressive results on the Minif2f test Benchmark and successfully solves 49 out of 658 problems Putnam bench – A collection of problems from the prestigious William Lowell Putnam Mathematical Competition.
Perhaps more impressive, when evaluated on 15 selected problems of recent American Invitational Mathematics Examination (AIME) Competitions, the model has successfully solved 6 problems. It is also interesting to note that, compared to Deepseekprover-V2, Deepseek-V3 8 of these problems resolved with the help of the majority of votes. This suggests that the gap between formal and informal mathematical reasoning quickly narrows in LLMS. However, the performance of the model about combination problems still require improvement, which emphasizes an area where future research could concentrate.
Vroerbench: a new benchmark for AI in mathematics
Deepseek researchers also introduced a new benchmark data set for evaluating the mathematical problem-solving capacity of LLMS. This benchmark, called Speechconsists of 325 formalized mathematical problems, including 15 problems of recent aime competitions, in addition to problems from textbooks and educational tutorials. These problems include fields such as number theory, algebra, calculus, real analysis and more. The introduction of aime problems is special of vital because it assesses the model on problems that not only require knowledge reminder, but also creative problem solving.
Open-source access and future implications
Deepseek-Prover-V2 offers an exciting chance with its open-source availability. Hosted platforms Just like cuddling face, the model is accessible to a wide range of users, including researchers, educators and developers. With both a more lightweight 7 billion parameter version and a powerful parameter version of 671 billion, deep researchers ensure that users with different areas of calculation can still benefit from it. This open access stimulates experiments and enables developers to make advanced AI tools for mathematical problem solving. As a result, this model has the potential to encourage innovation in mathematical research, to enable researchers to tackle complex problems and to reveal new insights into the field.
Implications for AI and mathematical research
The development of Deepseek-Prover-V2 has important implications, not only for mathematical research, but also for AI. The ability of the model to generate formal evidence can help mathematicians solve difficult racks, automate verification processes and suggesting new suspicions. In addition, the techniques used to make DeepSeek-Prover-V2 can influence the development of future AI models in other areas that depend on rigorous logical reasoning, such as software and hardware engineering.
The researchers want to scale up the model to tackle even more challenging problems, such as those at the International Mathematical Olympiad (IMO) level. This could further promote the skills of AI for proving mathematical propositions. As models such as Deepseek-Prover-V2 continue to evolve, they can redefine the future of both mathematics and AI, which means that progress in areas ranging from theoretical research to practical applications in technology.
The Bottom Line
Deepseek-Prover-V2 is an important development in AI-driven mathematical reasoning. It combines informal intuition with formal logic to break down complex problems and to generate verifiable evidence. The impressive performance on benchmarks show are potential to support mathematicians, automate evidence and even stimulate new discoveries in the field. As an open-source model, it is widely accessible and offers exciting opportunities for innovation and new applications in both AI and mathematics.