DeepMind’s Mind Evolution: Empowering Large Language Models for Real-World Problem Solving
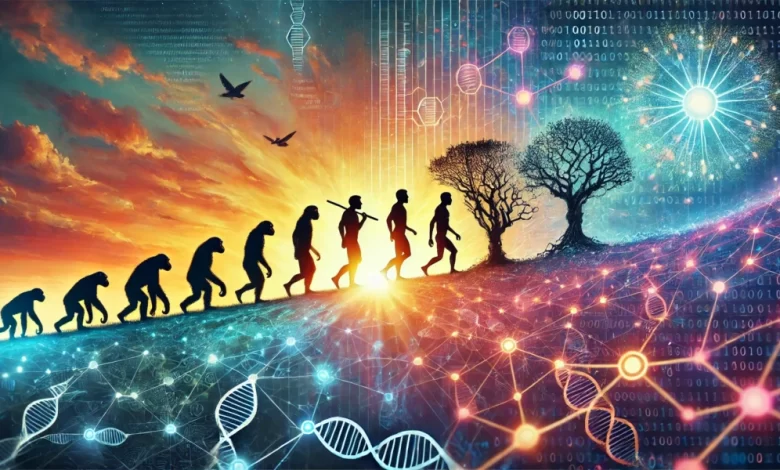
In recent years, artificial intelligence (AI) has emerged as a practical tool for stimulating innovation in industry. At the forefront of this progress there are large language models (LLMS) that are known to understand and generate their ability to understand and generate human language. Although LLMS performs well with tasks such as conversation-AI and making content, they often struggle with complex challenges in practice that require structured reasoning and planning.
For example, if you ask LLMS to plan a multi-urban business trip where flight schemes, meeting times, budget restrictions and adequate rest are coordinated, they can give suggestions for individual aspects. However, they are often confronted with challenges in integrating these aspects to effectively balance competitive priorities. This limitation is becoming even clearer because LLMS is increasingly being used to build AI agents who are able to resolve real-world problems autonomously.
Google DeepMind has recently developed a solution to tackle this problem. Inspired by natural selection, this approach, known as Mind EvolutionRefines problem -solving strategies through iterative adjustment. By leading LLMS in real time, they can effectively tackle complex real-world tasks and adapt to dynamic scenarios. In this article we will investigate how this innovative method works, the potential applications and what it means for the future of AI-driven problem solution.
Why LLMS is struggling with complex reasoning and planning
LLMs are trained to predict the following word in a sentence by analyzing patterns in large text data sets, such as books, articles and online content. This allows them to generate answers that seem logical and contextually suitable. However, this training is based on recognizing patterns instead of understanding the meaning. As a result, LLMS can produce text that seems logical, but struggles with tasks that require a deeper reasoning or structured planning.
The nuclear reduction lies in the way LLMS information processes. They focus on likely or patterns instead of logic, which means that they can process isolated tasks – such as suggesting flight options or hotel recommendations – but fail when these tasks have to be integrated into a coherent plan. This also makes it difficult for them to keep the context over time. Complex tasks often require when maintaining previous decisions and adjusting the new information is created. However, LLMS tends to lose the focus in extensive interactions, which leads to fragmented or inconsistent outputs.
How softening works
DeepMind’s sofa values address these shortcomings by accepting principles from natural evolution. Instead of producing a single response to a complex demand, this approach generates multiple potential solutions, it refines iteratively and selects the best results through a structured evaluation process. For example, consider brainstorming teams for a project. Some ideas are great, others less. The team evaluates all the ideas, keeps the best and throws the rest away. They then improve the best ideas, introduce new variations and repeat the process until they come to the best solution. Mind Evolution applies this principle to LLMS.
Here is a breakdown of how it works:
- Generation: The process starts with the LLM that creates multiple answers to a certain problem. In a travel plan, for example, the model can draw up different routes based on budget, time and user preferences.
- Evaluation: Each solution is assessed against a fitness function, a measure of how well it meets the requirements of the tasks. Low -quality reactions are thrown away, while the most promising candidates continue to the next phase.
- Refinement: A unique innovation of Mind evolution is the dialogue between two personas within the LLM: the author and the critic. The author proposes solutions, while the critic identifies errors and offers feedback. This structured dialogue reflects how people refine ideas through criticism and overhaul. For example, if the author proposes a travel plan with a restaurant visit that exceeds the budget, the critic points this out. The author then regains the plan to tackle the worries of the critic. This process enables LLMs to perform deep analysis that it could not perform before using other prompt techniques.
- Iterative optimization: The refined solutions undergo further evaluation and recombination to produce refined solutions.
By repeating this cycle, the mind -evolution iteratively improves the quality of solutions, so that LLM’s complex challenges can take on more effectively.
Mind evolution in action
DeepMind tested this approach benchmarks pretend Traveler And Nature plan. With the help of this approach, Google’s Gemini reached a success rate of 95.2% on travel planner, which is an excellent improvement compared to a basic line of 5.6%. With the more advanced Gemini Pro, the success rates rose to almost 99.9%. This transforming performance shows the effectiveness of spirit population in tackling practical challenges.
Interestingly, the effectiveness of the model grows with task complexity. While, for example, methods for one pass struggled with multi-day routes involving several cities, the mind-evolution consistently performed better than high success rates, even as the number of limitations increased.
Challenges and future directions
Despite its success, mind evolution is not without limitations. The approach requires important calculation sources because of the iterative evaluation and refinement processes. For example, solving a travel planner -task with mind -evolution used three million tokens and 167 API calls -substantially more than conventional methods. However, the approach remains more efficient than brute-force strategies such as exhausting search.
Moreover, designing effective fitness functions can be a challenging task for certain tasks. Future research can focus on optimizing the calculation efficiency and expanding the applicability of technology on a broader range of problems, such as creative writing or complex decision -making.
Another interesting area for exploration is the integration of domain -specific evaluators. With medical diagnosis, the inclusion of expert knowledge in the fitness function can, for example, further improve the accuracy and reliability of the model.
Applications beyond planning
Although mind revolution is mainly evaluated on planning tasks, it can be applied to various domains, including creative writing, scientific discovery and even generating codes. For example, researchers have introduced a benchmark called Stegpoet, who challenges the model to cod hidden messages in poems. Although this task remains difficult, the mind evolution exceeds traditional methods by reaching the success rate of up to 79.2%.
The ability to adjust and evolve solutions in natural language opens new opportunities for tackling problems that are difficult to formalize, such as improving workflows or generating innovative product designs. By using the power of evolutionary algorithms, Mind Evolution offers a flexible and scalable framework for improving the problem -solving possibilities of LLMS.
The Bottom Line
DeepMind’s Mind evolution introduces a practical and effective way to overcome important limitations in LLMS. By using iterative refinement inspired by natural selection, it improves the ability of these models to process complex, multi-step tasks that require structured reasoning and planning. The approach has already shown great success in challenging scenarios such as travel planning and shows promise about different domains, including creative writing, scientific research and the generation of codes. Although challenges such as high calculation costs and the need for well-designed fitness functions continue to exist, the approach offers a scalable framework for improving the AI options. Mind Evolution is the scene for more powerful AI systems that can reason and plan to resolve realistic challenges.