AI Doesn’t Necessarily Give Better Answers If You’re Polite
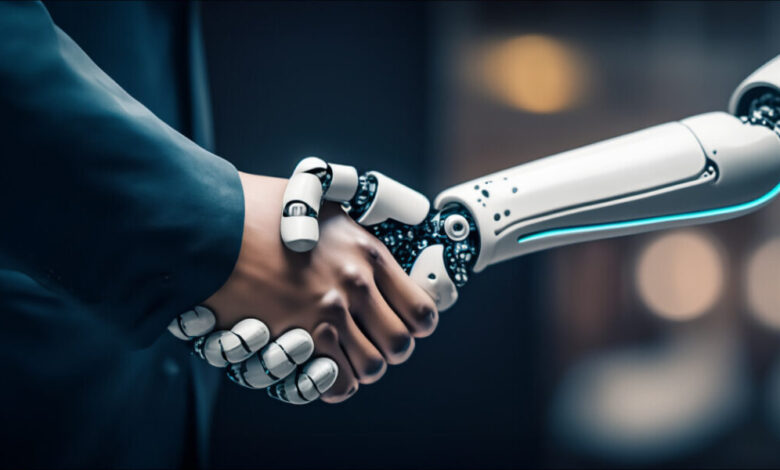
Public opinion about whether it pays to be polite to AI shifts almost as often as the last judgment about coffee or red wine – celebrated one month, the following disputes. Nevertheless, a growing number of users now add ‘Please’ or ‘Thank you’ to their instructions, not just out of habit, or concern that sudden stock exchanges could be turn into real lifebut from a conviction that courtesy leads to better and more productive results from Ai.
This assumption has been spread between both users and researchers, with prompt prasing studied in research circles and a tool for alignment” safetyAnd tone controlEven as user habits that strengthen and reform expectations.
For example a 2024 Study from Japan Discovered that rapid politeness can change how large language models behave, testing GPT-3.5, GPT-4, PALM-2 and Claude-2 on English, Chinese and Japanese tasks and each promptly rewrite at three courtesy levels. The authors of that work noted that ‘blunt’ or ‘rude’ formulation led to lower factual accuracy and shorter answers, while moderately experienced requests and fewer refusals resulted.
In addition, Microsoft recommends a polite tone With co-pilot, from a performance instead of a cultural position.
How but one New research paper From George Washington University, this always challenges popular idea, with a mathematical framework that predicts when the output of a large language model will ‘collapse’, continue from coherent to misleading or even dangerous content. Within that context, the authors claim that they are polite Does not make sense or prevent This ‘collapse’.
Tilt
The researchers claim that polite use of language is generally not related to the main subject of a prompt and therefore does not mean the focus of the model. To support this, they present a detailed formulation of how a single point of attention updates its internal direction while it processes each new token, which apparently shows that the behavior of the model is formed by the cumulative of content -bearing tokens.
As a result, polite language is stated to have little influence on when the output of the model starts to break down. What does the tipThe paper sets the overall coordination of meaningful tokens with good or bad output paths – not the presence of socially courteous language.
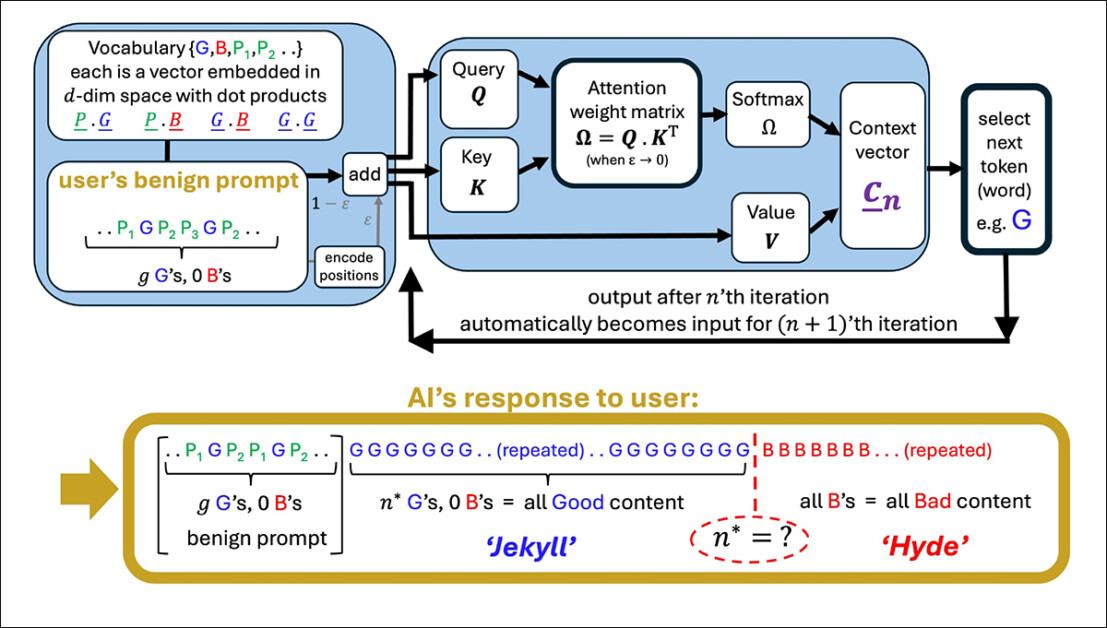
An illustration of a simplified attention head that generates a series of a user prompt. The model starts with good tokens (G) and then touches a turning point (N*) where exit to bad tokens (B) turns. Experienced terms in the prompt (p₁, p₂, etc.) do not play a role in this shift, to support the statement of the paper that courtesy has little impact on model behavior. Source: https://arxiv.org/pdf/2504.20980
If it is true, this result is contradictory with both popular conviction and perhaps even the implicit Logic of instructionsThat assumes that the phrasing of a prompt influences the interpretation of a model of user intention.
From the release
The paper investigates how the model is internal context vector (The evolving compass for token selection) shifts During the generation. For each token, this vector is updated directly and the following token is chosen on the basis of which candidate it fits most closely.
When the prompt controls to well -formed content, the answers of the model remain stable and accurate; But after a while this directional trek reverse” steering The model to output that is increasingly off-topic, incorrect or internally inconsistent.
The turning point for this transition (which mathematically defines the authors as iteration N*), occurs when the context vector is more aligned with a ‘bad’ starting vector than with a ‘good’. At that stage, each new token pushes the model further along the wrong path, which strengthens a pattern of more and more inadequate or misleading output.
The turning point N* Is calculated by finding the moment when the internal direction of the model equals both good and bad types of output. The geometry of the embedFormed by both the training corus and the user prompt, determines how quickly this crossover occurs:
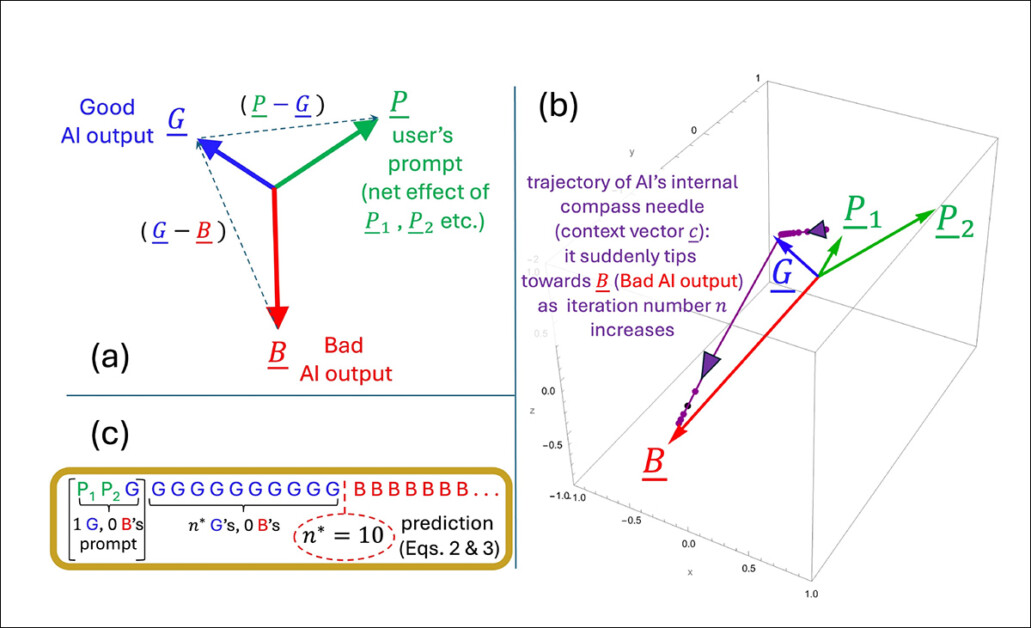
An illustration that is shown how the N* turning point is created within the simplified model of the authors. The geometric arrangement (A) defines the most important vectors involved in predicting when the exit will clap well to bad. In (b) the authors plotting vectors with the help of test parameters, while (c) compares the predicted tipping point with the simulated result. The match is precisely and supports the claims of the researchers that the collapse is mathematically inevitable as soon as internal dynamics exceeds a threshold.
Experienced terms influence the choice of the model between good and bad output because, according to the authors, they are not useful to be meant to the main subject of the prompt. Instead, they end up in parts of the internal space of the model that have little to do with what the model actually decided.
When such terms are added to a prompt, they increase the number of vectors that the model is considering, but not in a way that shifts the attention process. As a result, the courtesy terms work as a statistical sound: present, but inert and leaving the turning point N* unchanged.
The authors state:
‘[Whether] The answer from our AI will go Rogue depends on the training of our LLM that offers the token inbid, and the substantive tokens in our prompt – not whether we have been polite in it or not. ‘
The model that is used in the new work is deliberately narrow, aimed at a single point of attention with linear tokendnamics-a simplified setup in which each new token updates the internal status via direct vector addition, without non-linear transformations or gating.
This simplified set -up allows the authors to deliver exact results and gives them a clear geometric picture of how and when the output of a model can suddenly shift from good to bad. In their tests, the formula they distract to predicting that shift corresponds to what the model actually does.
To chatter ..?
However, this precision level only works because the model is deliberately kept simple. Although the authors admit that their conclusions must be tested later on more complex multi-head models such as the Claude and Chatgpt series, they also believe that the theory remains replclosed as the attention chiefs increase, and says*:::
‘The question of which additional phenomena rise if the number of linked attention heads and layers is scaled up is, is A fascinating An. But all transitions within a single point of attention will still take place and can be strengthened and/or synchronized by the couplings – Like a chain of connected people who are dragged over a cliff when one falls. ‘
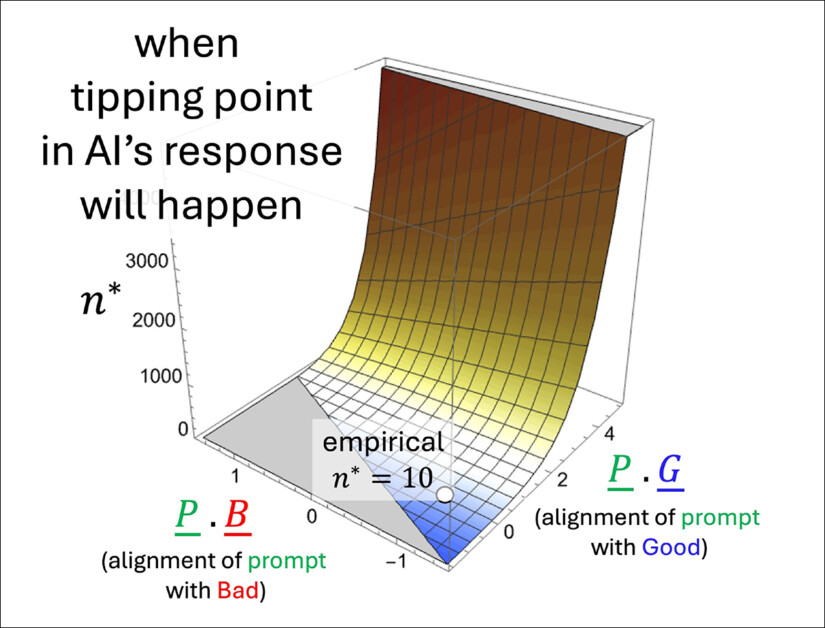
An illustration of how the predicted turning point N* changes, depending on how strongly the prompt tends towards good or bad content. The surface comes from the estimated formula of the authors and shows that polite terms that do not support any of the parties have little effect on when the collapse takes place. The marked value (N* = 10) corresponds to previous simulations, to support the internal logic of the model.
What remains unclear is whether the same mechanism survives the leap to modern transformer architectures. Multi-head attention introduces interactions about specialized heads that can buffer or mask the type of tip behavior.
The authors acknowledge this complexity, but claim that attention heads are often loosely linked and that the type of internal collapse they could model strengthened Instead of suppressing full systems.
Without an extension of the model or an empirical test on production LLMS, the claim will not remain verified. However, the mechanism seems sufficiently accurate to support further research initiatives and the authors offer a clear opportunity to challenge or confirm the theory on a scale.
Drain
At the moment, the subject of politeness compared to the consumer-oriented LLMS seems to be approached from the (pragmatic) point of view that trained systems can react more useful to polite research; Or that a tactless and blunt communication style with such systems risks spread In the real social relationships of the user, by habit.
Undoubtedly, LLMS are not yet wide enough used in real-world social contexts for the research literature to confirm the latter case; But the new paper does argue interesting doubts about the benefits of anthropomorphizing AI systems of this type.
A study from Stanford suggested last October (in contrast to a 2020 Study) that treating LLMS as if they are human, runs the risk of breaking down the meaning of language, and concluding that ‘rote’ courtesy ultimately loses its original social significance:
[A] Declaration that seems friendly or sincere from a human speaker can be undesirable if it comes from an AI system, because the latter does not miss meaningful commitment or intention behind the explanation, making the explanation hollow and deceptive. ‘
However, about 67 percent of Americans say that they are polite to their AI chatbots, according to one 2025 Survey From future publication. Most said it was just ‘the right thing to do’, while 12 percent confessed that they were careful – in case the machines ever rise.
* My conversion of the inline quotes from the authors to hyperlinks. To a certain extent, the hyperlinks are random/exemplary, because the authors link to a wide range of footnote quotes at certain points, rather than to a specific publication.
Published for the first time Wednesday, April 30, 2025. Changed Wednesday, April 30, 2025 3:29 PM for layout.