Agent Laboratory: A Virtual Research Team by AMD and Johns Hopkins
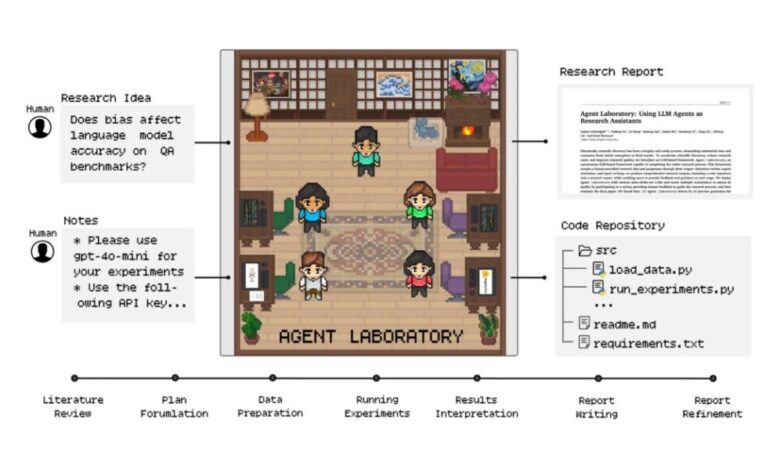
While everyone is talking about AI agents and automation, AMD and Johns Hopkins University have been working to improve the way humans and AI collaborate in research. Their new open source framework, Agent laboratoryis a completely new look at how scientific research can be accelerated through teamwork between humans and AI.
After reviewing numerous AI research frameworks, Agent Laboratory stands out for its practical approach. Rather than trying to replace human researchers (like many existing solutions), it focuses on increasing their capabilities by tackling the time-consuming aspects of research while keeping humans in the driver’s seat.
The core innovation here is simple but powerful: Rather than pursuing fully autonomous research (which often leads to questionable results), Agent Laboratory creates a virtual laboratory where multiple specialized AI agents work together, each handling different aspects of the research process while remaining anchored to human guidance.
Tear down the virtual laboratory
Think of Agent Laboratory as a well-orchestrated research team, but with AI agents playing specialized roles. Just like a real research laboratory, each agent has specific responsibilities and expertise:
- A PhD agent is involved in literature research and research planning
- Postdocs help refine experimental approaches
- ML Engineer agents take care of the technical implementation
- Professor agents evaluate and score research results
What makes this system particularly interesting is the workflow. Unlike traditional AI tools that work in isolation, Agent Laboratory creates a collaborative environment where these agents interact and build on each other’s work.
The process follows a natural research progression:
- Literature overview: The PhD student searches academic articles using the arXiv APIcollecting and organizing relevant research
- Plan formulation: PhD and postdoc agents work together to develop detailed research plans
- Execution: ML Engineer agents write and test code
- Analysis & Documentation: The team works together to interpret the results and generate comprehensive reports
But here’s where it gets really practical: The framework is computationally flexible, meaning researchers can allocate resources based on their access to computing power and budget constraints. This makes it a tool designed for real research environments.
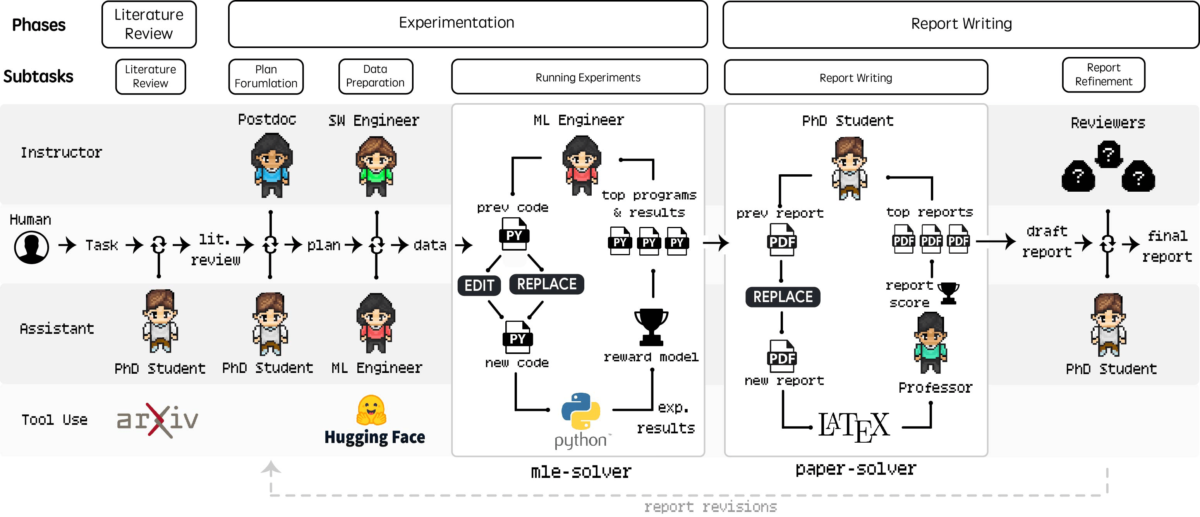
Schmidgall et al.
The human factor: where AI meets expertise
While Agent Laboratory offers impressive automation capabilities, the real magic happens in what they call “co-pilot mode.” In this setup, researchers can provide feedback at every stage of the process, creating a true collaboration between human expertise and AI support.
The co-pilot feedback data reveals some compelling insights. In autonomous mode, Agent Laboratory generated articles scored an average of 3.8/10 in human evaluations. But when researchers were in co-pilot mode, those scores rose to 4.38/10. What’s particularly interesting is where these improvements manifested themselves: the articles scored significantly higher in clarity (+0.23) and presentation (+0.33).
But here’s the reality check: even with human intervention, these papers still scored about 1.45 points below the accepted average NeurIPS paper (which is at 5.85). This is not a failure, but it is a crucial lesson in how AI and human expertise should complement each other.
Another fascinating thing emerged from the evaluation: AI reviewers consistently rated articles about 2.3 points higher than human reviewers. This gap highlights why human oversight remains crucial in research evaluation.
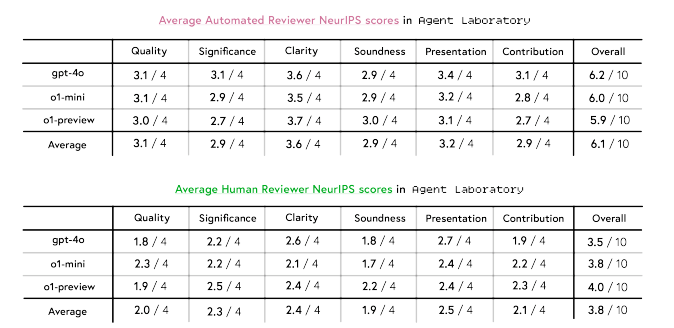
Schmidgall et al.
Breaking down the numbers
What really matters in a research environment? The cost and performance. Agent Laboratory’s approach to model comparison reveals some surprising efficiency gains in this regard.
GPT-4o emerged as the speed champion, completing the entire workflow in just 1,165.4 seconds – that’s 3.2x faster than o1-mini and 5.3x faster than o1-preview. But more importantly, it only costs $2.33 per paper. Compared to previous autonomous research methods that cost around $15, we are looking at an 84% cost reduction.
Looking at model performance:
- o1-preview scored highest on usability and clarity
- o1-mini achieved the best experimental quality scores
- GPT-4o lagged behind in metrics, but led in cost efficiency
The real world implications here are significant.
Researchers can now choose their approach based on their specific needs:
- Need rapid prototyping? GPT-4o offers speed and cost efficiency
- Prioritize experimental quality? o1-mini may be the best choice
- Looking for the most polished output? o1 preview is promising
This flexibility means research teams can tailor the framework to their resources and requirements, rather than being stuck with a one-size-fits-all solution.
A new chapter in research
After exploring the capabilities and results of Agent Laboratory, I am convinced that we are facing a significant change in the way research will be conducted. But it’s not the story of replacement that often dominates the headlines – it’s something much more nuanced and powerful.
While the Agent Laboratory papers themselves do not yet meet the highest conference standards, they create a new paradigm for research acceleration. Think of it as a team of AI research assistants who never sleep, each specializing in different aspects of the scientific process.
The implications for researchers are profound:
- The time spent on literature research and basic coding can be spent on creative ideas
- Research ideas that might have been shelved due to limited resources become viable
- The ability to quickly prototype and test hypotheses could lead to faster breakthroughs
Current limitations, such as the gap between AI and human assessment scores, offer opportunities. Each iteration of these systems brings us closer to a more advanced research collaboration between humans and AI.
Looking ahead, I see three important developments that could reshape scientific discoveries:
- More sophisticated human-AI collaboration patterns will emerge as researchers learn to deploy these tools effectively
- The cost and time savings could democratize research, allowing smaller labs and institutions to pursue more ambitious projects
- The capabilities for rapid prototyping could lead to more experimental approaches to research
The key to maximizing this potential? Understand that Agent Laboratory and similar frameworks are tools for reinforcement, not automation. The future of research isn’t about choosing between human expertise and AI capabilities – it’s about finding innovative ways to combine them.