From o1 to o3: How OpenAI is Redefining Complex Reasoning in AI
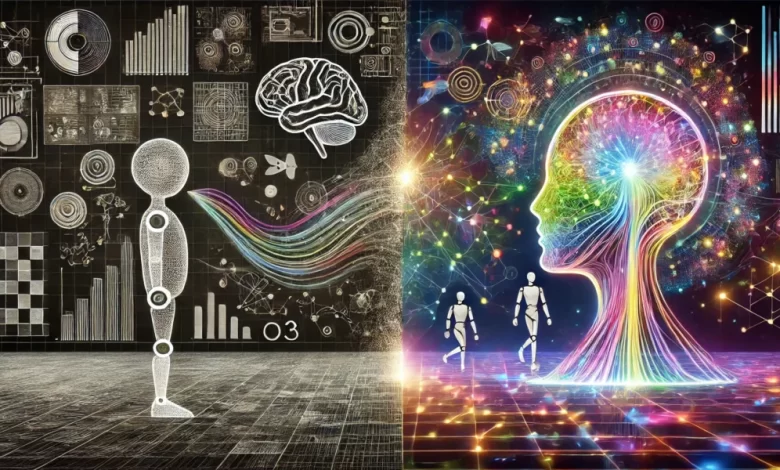
Generative AI has redefined what we think AI can do. What started as a tool for simple, repetitive tasks now solves some of the most challenging problems we face. OpenAI has played a major role in this shift and is leading the way with its ChatGPT system. Early versions of ChatGPT showed how AI could have human-like conversations. This capability offers a glimpse into what was possible with generative AI. Over time, this system has gone beyond simple interactions to tackle challenges that require reasoning, critical thinking and problem solving. This article explores how OpenAI transformed ChatGPT from a conversational tool to a system that can reason and solve problems.
o1: The first leap to real reasoning
OpenAI’s first step towards reasoning came with the release of o1 in September 2024. Before o1, GPT models were good at understanding and generating text, but they struggled with tasks that required structured reasoning. o1 changed that. It is designed to focus on logical tasks, breaking down complex problems into smaller, manageable steps.
o1 achieved this by using a technique called reasoning chains. This method helped the model tackle complex problems, such as math, science and programming, by dividing them into easy-to-solve parts. This approach made o1 much more accurate than previous versions such as GPT-4o. For example, when tested on advanced math problems, o1 solved 83% of the questions, while GPT-4o solved only 13%.
o1’s success did not only come from reasoning chains. OpenAI also improved the way the model was trained. They used custom datasets focused on math and science and applied large-scale reinforcement learning. This helped o1 perform tasks that required several steps to solve. The extra computational time spent on reasoning proved to be a key factor in achieving accuracy that previous models could not match.
o3: Taking reasoning to the next level
Building on the success of o1, OpenAI has now done just that launched o3. Released during the “12 days OpenAI” event, this model takes AI thinking to the next level with more innovative tools and new capabilities.
One of the most important improvements in o3 is its adaptability. It can now check its answers against specific criteria to ensure they are accurate. This capability makes o3 more reliable, especially for complex tasks where precision is crucial. Think of it as a built-in quality control that reduces the chance of errors. The disadvantage is that it takes a little longer to arrive at answers. It can take a few extra seconds or even minutes to solve a problem, compared to models that don’t use reasoning.
Like o1, o3 is trained to ‘think’ before answering. This training enables o3 to perform chain-of-thought reasoning using reinforcement learning. OpenAI calls this approach a ‘private thought chain’. It allows o3 to break down problems and think about them step by step. When o3 gets a prompt, it doesn’t rush with an answer. It takes time to consider related ideas and explain their reasoning. After this, it summarizes the best response it can think of.
Another useful feature of o3 is the ability to adjust how much time it spends reasoning. If the task is simple, o3 can act quickly. However, it can use more computing resources to improve accuracy for more complicated challenges. This flexibility is essential because it allows users to monitor the model’s performance based on the task.
In early tests, o3 showed great potential. On the ARC-AGI benchmarkthat tests AI on new and unfamiliar tasks, o3 scored 87.5%. This performance is a strong result, but also indicates where the model can improve. While it performed excellently on tasks like coding and advanced math, it occasionally struggled with simpler problems.
Has o3 achieved artificial general intelligence (AGI)?
Although o3 significantly improves AI reasoning skills by scoring highly on the ARC Challenge, a benchmark designed to test reasoning and adaptability, it still falls short of human-level intelligence. The organizers of the ARC Challenge have done just that clarified that while o3’s performance reached an important milestone, it is only a step in the direction AGI and not the final result. While o3 can adapt to new tasks in impressive ways, it still struggles with simple tasks that are easy for humans. This shows the gap between current AI and human thinking. Humans can apply knowledge in different situations, while AI still struggles with that level of generalization. So while O3 is a remarkable development, it doesn’t yet have the universal problem-solving capabilities needed for AGI. AGI remains a goal for the future.
The way forward
o3’s advancement is a big moment for AI. It can now solve more complex problems, from coding to advanced reasoning tasks. AI is getting closer to the idea of AGI and the potential is enormous. But with this progress comes responsibility. We need to think carefully about how we move forward. There is a balance between encouraging AI to do more and ensuring it is secure and scalable.
o3 still faces challenges. One of the biggest challenges for o3 is the need for a lot of computing power. Running models like o3 takes significant resources, making this technology difficult to scale and limiting its widespread use. Making these models more efficient is critical to ensuring they reach their full potential. Safety is another primary concern. The more capable AI becomes, the greater the risk of unintended consequences or misuse. OpenAI has already implemented some security measures, such as “purposeful coordination”, which help the model’s decision-making in following ethical principles. However, as AI continues to develop, these measures will need to evolve.
Other companies, such as Google and Deep Searchare also working on AI models that can handle similar reasoning tasks. They face similar challenges: high costs, scalability and security.
The future of AI is promising, but hurdles still exist. Technology is at a crossroads and how we handle things like efficiency, security and accessibility will determine where it goes. It’s an exciting time, but it requires careful thought to ensure AI can reach its full potential.
The bottom line
OpenAI’s move from o1 to o3 shows how far AI has come in reasoning and problem solving. These models have evolved from performing simple tasks to tackling more complex tasks, such as advanced math and coding. o3 stands out for its adaptability, but is still not at the level of Artificial General Intelligence (AGI). Although it can handle a lot, it still struggles with some basic tasks and requires a lot of computing power.
The future of AI looks bright, but it also brings challenges. Efficiency, scalability and security deserve attention. AI has made impressive progress, but there is still more work to be done. OpenAI’s progress with o3 is a significant step forward, but AGI is still on the horizon. How we tackle these challenges will shape the future of AI.