The Hidden Role of Data Annotation in Everyday AI Tools
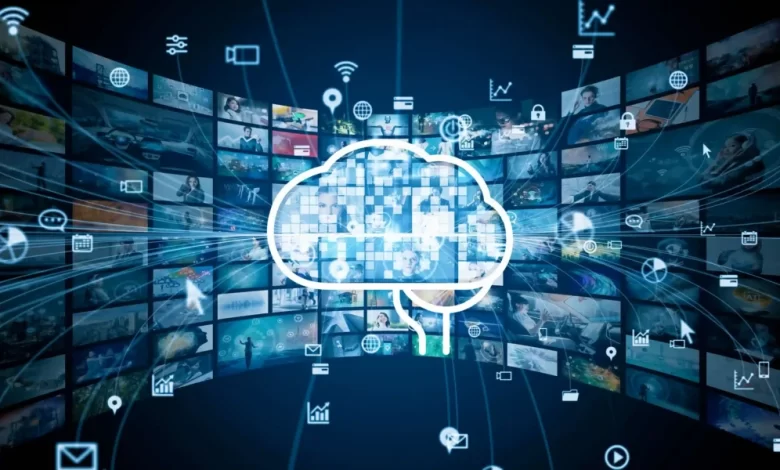
A recent research of 6,000 consumers revealed something intriguing: While only about 33% of people think they use AI, a remarkable 77% actually use AI-powered services or devices in their daily lives.
This gap highlights how many people may not realize how much artificial intelligence is impacting their routines. Despite AI’s impressive capabilities, the underlying processes that make these tools effective often go unnoticed.
Every interaction with AI involves complex algorithms that analyze data to make decisions. These algorithms rely on simple actions, such as checking travel times or receiving personalized content suggestions.
- But how do these algorithms learn to understand our needs and preferences?
- How do they make accurate predictions and provide relevant information?
The answer lies in a crucial process known as data annotation.
What is data annotation?
“Data annotation involves labeling data so that machines can learn from it. This process involves tagging images, text, audio or video with relevant information. For example, if you annotate an image, you can identify objects such as cars, trees or people.”
Consider teaching a child to recognize a cat. You showed them pictures and said, ‘This is a cat.Data annotation works the same way. People carefully label data points such as images and audio with tags that describe their characteristics.
- An image of a cat could be labeled “cat,” “animal,“And”feline”,.
- A video of a cat can be tagged with labels like ‘cat,” “animal,” “feline,” “walking,” “run,” etc.
Simply put, data annotation enriches the machine learning (ML) process by adding context to the content so that models can understand and use this data for predictions.
The evolving role of data annotation
Annotating data has gained enormous importance in recent years. Initially, data scientists worked primarily with structured data, which required minimal annotation. However, the rise of machine learning systems has dramatically changed this domain.
Today, unstructured data dominates the digital space. Examples include:
- Emails
- Social media posts
- Images
- Audio files
- Sensor data
Machine learning algorithms face significant challenges in making sense of this vast information without proper annotation. They can easily become overwhelmed and unable to differentiate between different data points.
This implies that high-quality labeled data directly impacts AI performance. When machines are trained with accurate labels, they understand the tasks better. This leads to better decision-making capabilities and more reliable results.
Annotation improves AI accuracy: examples show how
“Data is the food for artificial intelligence. If an AI eats junk food, it won’t perform as well.” – Matthew Emerick.
This concept is clearly visible in everyday technology.
Take navigation apps like Google Maps as an example. If the training data contains errors or inconsistencies, users may follow incorrect routes or encounter unexpected detours. A simple mislabeling of a street can significantly disrupt travel plans.
Also consider online shopping platforms that recommend products based on user behavior. Poorly annotated data can result in irrelevant suggestions, frustrate customers and worsen their overall experience.
Manual versus automated annotation: a collaborative approach
AI systems owe much of their accuracy and efficiency to data annotation, combining manual expertise with automated processes. Advanced tools and advanced technologies can handle basic labeling tasksbut human input is essential to refine details and add contextual understanding.
The Human Touch: why machines can’t do it alone
The collaboration between skilled annotators and advanced technologies bridges gaps where automation falls short. Human annotators provide a level of understanding that machines cannot replicate. They recognize nuances in language, context and imagery that automated systems may miss.
Annotators rigorously review data, correct errors, and ensure that the data meets the quality needed for reliable AI performance. This human touch is especially vital for complex tasks such as sentiment analysis in text or identifying subtle objects in images.
The scale of data annotation
The volume of data annotation required to train AI models is unprecedented.
Developing technologies such as self-driving cars requires millions of annotated images and videos. Each frame must be labeled with precision to represent real-world conditions such as traffic signs, vehicles, pedestrians, and weather changes. These efforts ensure that the algorithms can correctly interpret their environment and make safe decisions.
Real-world examples of AI tools that use annotated data
Various AI tools in daily use rely heavily on annotated data to function effectively. These examples illustrate the importance of data annotation in improving user experience and improving decision making.
Google Maps
Google Maps is a widely recognized AI tool that uses annotated map data. For accurate navigation, it relies on labeled information about roads, traffic patterns, and landmarks. When users search for directions, the system analyzes this annotated data to recommend the best routes based on real-time conditions.
Updates such as road closures or accidents are smoothly integrated, allowing the app to adapt quickly and keep users informed.
YouTube recommendations
YouTube’s recommendation engine relies on tagged data to suggest videos based on your preferences. It annotates videos with details such as genre, content and user engagement. This allows the AI to recognize your viewing behavior and recommend similar content.
Accurate annotations ensure that YouTube’s algorithm suggests videos that are relevant to your interests.
Smart Home Devices
Smart home devices, including voice assistants and security systems, rely on annotated data to function effectively. When a user issues a command such as “turn on the lights”, the device uses tagged voice data to accurately interpret the request.
Annotations help these systems recognize different accents and speech patterns, improving responsiveness. In home security, AI analyzes sensor data to detect unusual activity, using tagged information to decide when to send alerts.
Diagnostics in healthcare
AI tools use annotated medical images to improve diagnostic capabilities in healthcare. Techniques such as tumor detection and organ segmentation rely on accurately labeling medical images.
In addition to imaging, AI is also making progress memory care. Annotated data plays a crucial role in developing tools that aid cognitive health.
Final Thoughts: Why Data Annotation Is More Important Than Ever
It is expected that global data creation will surpass limits 180 zettabytes in 2025the demand for accurate and comprehensive data labeling will only increase. For example, a few years ago, labeling just a few points on a face was enough to create an AI prototype. Nowadays, up to 20 points can appear on the lips.
Understanding the importance of data annotation helps us appreciate the hidden work that powers the AI systems we use every day. As these technologies get smarter, so will labeling methods, making data annotation an essential part of the future of AI.
Visit unite.ai to stay up to date with the latest AI news, innovations, and everything in between.