AI Meets Spreadsheets: How Large Language Models are Getting Better at Data Analysis
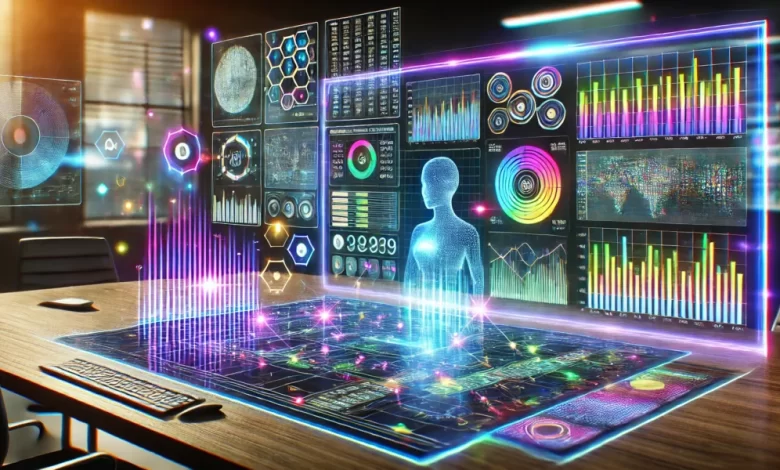
Spreadsheets have been an important tool for organizing data, financial modeling and operational planning in companies across industries. Originally designed for basic calculations and simple data management, their functionality has expanded as the need for data-driven insights grew. Today, enterprises need real-time data analysis, advanced analytics, and even predictive capabilities within the familiar spreadsheet format. As spreadsheet programs become more sophisticated, many non-technical users find it increasingly difficult to navigate and take full advantage of these complex features.
Large Language Models (LLMs), advanced AI models that can understand and generate human language, are changing this domain. Developed by companies like OpenAI, MicrosoftAnd Googling change the way users interact with spreadsheets. By integrating AI directly into platforms like Excel and Google Sheets, LLMs enhance spreadsheets with natural language capabilities that simplify complex tasks. Users can now perform complex data analysis, automate workflows and generate insights by simply typing a request in plain language. This shift allows spreadsheets to serve as intuitive, AI-powered data analysis tools, breaking down technical barriers and democratizing access to meaningful insights at all levels of an organization.
Background information on large language models (LLMs)
To understand how LLMs transform spreadsheets, it is important to know their evolution. LLMs are powerful AI systems trained on vast amounts of data, such as books, websites and specialized content. These models learn to understand language nuances, context and even industry-specific jargon.
In their early days, language models could only perform simple tasks such as classifying text. But modern LLMs, like GPT-4 And Llamais a completely different story. They generate human-like text and can handle complex data processing and analysis, making them incredibly useful for data-intensive tasks such as spreadsheet analysis.
A significant advance came with GPT-3, which improved the way models understood and interacted with language. Each new version has become better at handling complex tasks, faster at processing queries, and better at understanding context. Today, the demand for LLMs in data analytics is so high that the sector is experiencing rapid growth, with these models expected to play a major role in business intelligence.
These advances are reflected in tools such as Microsoft’s Copilot for Excel and Duet AI from Google Sheetswhich add LLM capabilities directly to the spreadsheet software that millions of people already use. These tools allow people to gain valuable insights from data without specialized technical skills, which is especially useful for small and medium-sized businesses. Access to AI-powered data analytics can make a big difference for these companies, providing the same competitive insights typically available to larger companies with data science teams.
How LLMs transform data analysis into spreadsheets
LLMs transform data analysis within spreadsheets, bringing advanced data processing and accuracy improvements directly to familiar tools like Microsoft Excel and Google Sheets. Traditionally, spreadsheet users had to rely on complex formulas and nested functions for data processing, which could be challenging and error-prone, especially for non-technical users. LLMs allow users to easily enter commands in plain language, such as ‘Calculate the annual growth” or “Highlight sales discrepancies”, allowing the model to generate the right formulas or provide immediate insights. This natural language capability significantly reduces the time spent on analysis and improves accuracy. This is an advantage in rapidly evolving areas such as e-commerce and finance.
In addition to data processing, LLMs excel at automating essential data cleaning tasks that are crucial for accurate analysis. Users can instruct the model to perform tasks such as “normalize data to MM/DD/YYYY” or “fill in missing values with the median.” The model performs these processes in seconds, ensuring higher data quality and improving downstream analytics. Studies have shown that AI is powered data cleansing significantly improves the accuracy of data analysis, making these capabilities especially useful for users who need reliable insights without spending a lot of time on data preparation.
Another crucial advantage of LLMs is their ability to interpret data trends and generate natural language summaries. For example, a marketer might ask: “What are the most important sales trends of the past year?‘ and get a concise summary of critical insights without having to manually scroll through large data sets. This ease of trend analysis and summary generation has made it easier for non-technical users to understand and act on data insights. Surveys show that many users find LLMs improve their ability to interpret data for strategic planning, demonstrating a growing reliance on AI for informed decision-making.
LLMs also play a crucial role in democratizing data analytics by reducing the need for specialized technical skills. With LLM integrations, non-technical professionals from different departments can independently access advanced data insights. For example, a retail manager can analyze customer trends without having to rely on a data specialist. This accessibility allows organizations at every level to make data-driven decisions, fostering a culture of informed, flexible decision-making.
LLMs are now embedded directly into spreadsheet tools, with examples like Microsoft’s Copilot in Excel and Google’s Duet AI in Google Sheets. These integrations enable formula generation, data categorization, and visualizations using simple language prompts. For example, a financial analyst might type: ‘Show a trend line for quarterly revenue growth”, and the model will produce the visualization, streamlining a task that would otherwise be manual and time-consuming.
Challenges and limitations of LLMs in data analysis
While LLMs provide powerful data analytics capabilities, they come with significant challenges and limitations. These issues are especially relevant in sensitive or high-stakes environments, where accuracy and privacy are essential.
First, data privacy and security are critical. Because many LLMs are cloud-based, they pose potential risks for sensitive data exposure. Regulations such as GDPR And CCPA maintain strict data protection requirements, so companies using LLMs must ensure compliance by implementing robust security protocols. Solutions include using models that process data locally or improving data encryption and anonymization. These measures help limit the risk of data breaches or unauthorized access, which is critical when handling personal or proprietary information.
Another challenge is accuracy and reliability. Although LLMs are highly sophisticated, they are not immune to mistakes. They may misinterpret vague or complex clues, potentially leading to incorrect insights. This is especially problematic in areas such as finance or healthcare, where decisions based on faulty data can have significant consequences.
LLMs also struggle with noisy or contextless data sets, which affects the accuracy of the output. To address this, many organizations are integrating human oversight and AI verification checks to validate results so they meet reliability standards before being used in critical decisions.
Furthermore, technical limitations make integrating LLMs within existing systems, such as spreadsheets, challenging. Processing large data sets in real time or scaling LLM applications requires significant computing power. Additionally, because LLMs require regular updates to stay relevant, especially for domain-specific tasks, maintaining them can be resource-intensive. For many companies, it is an ongoing challenge to combine these technical requirements with the benefits of LLMs.
These limitations highlight the need for strategic planning, especially for organizations that want to effectively integrate LLMs while protecting data integrity and ensuring operational reliability.
Future trends and innovations
The future of LLMs in spreadsheet-based data analytics is promising, and some exciting developments are expected. A big trend is customization and personalization. Future LLMs are expected to learn from users’ past interactions and tailor their responses to specific preferences. This means users can get faster, more relevant insights without having to adjust settings every time.
Collaboration is another area where LLMs will improve. Soon, multiple users will be able to collaborate on the same spreadsheet and make real-time updates and decisions. This could transform spreadsheets into powerful collaboration tools that allow team members to instantly exchange ideas and see changes.
Furthermore, we may soon see the integration of multi-modal AI capabilities. This technology allows LLMs to work with text, numbers, images and structured data simultaneously. Imagine you’re analyzing a data set that combines sales figures and customer reviews in one spreadsheet. This would provide a more complete and holistic picture, making the analysis more comprehensive and insightful.
These developments will make LLMs even more useful, allowing users to make smarter, faster decisions and collaborate more effectively.
The bottom line
The rise of LLMs in spreadsheets is changing the way we interact with data. What once required complex formulas and specialized skills can now be handled by simply typing what we need in everyday language. This shift means that data analysis is no longer the preserve of technical experts. Now professionals from all backgrounds can benefit from powerful insights, make informed decisions and get the most out of their data.
Yet LLMs, like any innovation, bring both opportunities and challenges. Data privacy, model reliability, and technical requirements are fundamental considerations for companies using these tools. Companies should use LLMs carefully, ensuring they protect sensitive information and validate the insights AI generates.