Improvements in ‘reasoning’ AI models may slow down soon, analysis finds
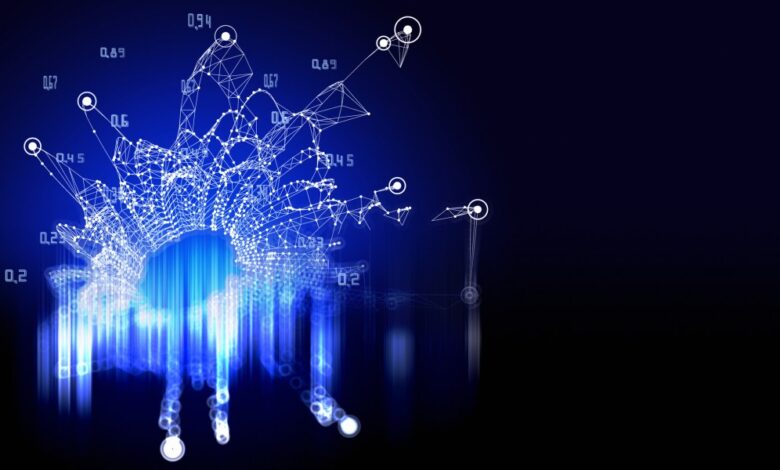
A analysis By Epoch AI, a non -profit AI Research Institute, suggests that the AI industry may not be able to win enormous performance outlet from the reasoning of AI models for much longer. As soon as the progress of reasoning models could slow down within a year, according to the findings of the report.
In recent months, reasoning models such as OpenAi’s O3 have led to substantial profit on AI benchmarks, in particular benchmarks that measure mathematics and programming skills. The models can apply more computing to problems, which can improve their performance, with the disadvantage that they last longer than conventional models to complete tasks.
Reasoning models are developed by first training a conventional model on a huge amount of data and then applying a technique called reinforcement learning, which effectively gives “feedback” the model about its solutions to difficult problems.
Until now, Frontier AI laboratories such as OpenAI have not applied an enormous amount of computing power to the learning stage of learning the reasoning model.
That changes. OpenAi has said that it has applied approximately 10x more computing to train O3 than its predecessor, O1 and Epoch speculates that the majority of this computer use was devoted to learning reinforcement. And OpenAi researcher Dan Roberts recently demonstrated that the company’s future plans are asking Give priority to learning reinforcement To use much more computing power, even more than for the initial model training.
But there is still an upper limit how much computing can be applied to learning reinforcement, per period.

Josh you, an analyst at Epoch and the author of the analysis, explains that performance profits of standard AI modelt training currently quadrate every year, while performance profits of learning reinforcement grow ten times every 3-5 months. The progress of the reasoning training will probably come together with the overall border by 2026, “he continues.
The analysis of Epoch makes a number of assumptions and is partly based on public comments from AI Company Executives. But it also claims that scaling of reasoning models can prove to be a challenge for reasons in addition to computer use, including high overhead costs for research.
“If there are a persistent overhead costs required for research, reasoning models cannot be as far as expected,” you write. “Fast calculation is potentially a very important ingredient in the progress of the reasoning model, so it is worth following this closely.”
Every indication that reasoning models can reach a sort of limit in the near future will probably be concerned about the AI industry, which has invested enormous resources that develop these types of models. Studies have already shown that reasoning models, which can be incredibly expensive to turn, have serious defects, such as a tendency to hallucinate more than certain conventional models.