How Does Claude Think? Anthropic’s Quest to Unlock AI’s Black Box
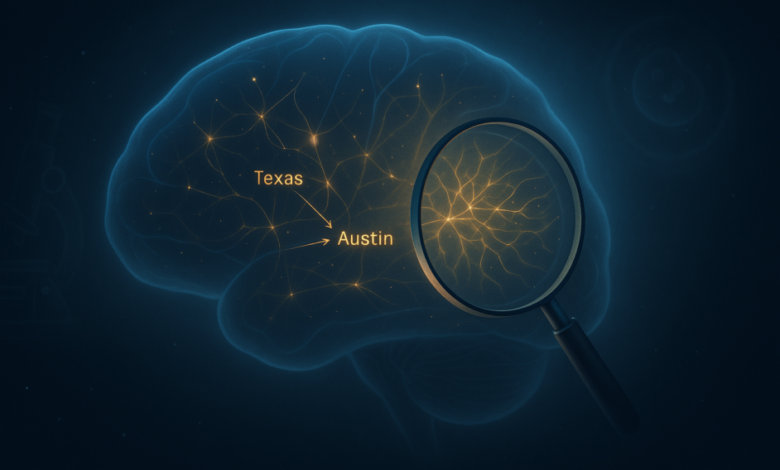
Large language models (LLMs) such as Claude have changed the way we use technology. They are electricity tools such as chatbots, help to write essays and even make poetry. But despite their great skills, these models are still a mystery in many ways. They often call them a “black box” because we can see what they say, but not how they find out. This lack of understanding causes problems, especially in important areas such as medicine or rights, where errors or hidden prejudices can really cause damage.
Insight into how LLMS works is essential for building trust. If we cannot explain why a model has given a certain answer, it is difficult to trust the results, especially in sensitive areas. Interpretability also helps to identify and resolve prejudices or errors, so that the models are safe and ethical. For example, if a model promotes certain geenspoints consistently, you can know why developers can help it to correct it. This need for clarity is what drives research to make these models more transparent.
Anthropic, the company behind Claude, has worked to open this black box. They have made exciting progress when finding out what LLMS thinks, and this article is investigating their breakthroughs by making Claude’s processes easier to understand.
Mapping Claude’s thoughts
In mid -2024, the Anthropic team made an exciting one breakthrough. They created a basic “map” of how Claude processes information. With the help of a technique called Dictionary Learning, they found millions of patterns in Claude’s “Brain” – the neural network. Each pattern or “function” connects to a specific idea. Some functions help, for example, claude cities, famous people or code errors. Others bind to more difficult subjects, such as gender proportion or confidentiality.
Researchers discovered that these ideas are not isolated within individual neurons. Instead, they are spread over many neurons of the Claude network, where every neuron contributes to different ideas. That overlap made anthropically difficult time to find out these ideas in the first place. But by seeing these recurring patterns, the researchers of Anthropic started decoding how Claude organizes his thoughts.
Reasoning Claude Tracing
Then Anthropic wanted to see how Claude uses those thoughts to make decisions. They recently built a tool called Attribution -graphicsIt works as a step -by -step manual for Claude’s thinking process. Every point in the graph is an idea that lights up in Claude’s Spirit, and show the arrows how one idea flows into the next. With this graph, researchers can keep track of how Claude turns a question in an answer.
Consider this example to better understand the functioning of attribution graphs: “What is the capital of the state with Dallas?” Claude must realize that Dallas is in Texas and reminds that the capital of Texas is Austin. The attribution graph showed this exact process – part of Claude marked “Texas”, which led to another part that “Austin” Koos. The team even tested it by adjusting the “Texas” section, and yes, it changed the answer. This shows that Claude not only gambles – it works because of the problem, and now we can see it happen.
Why this matters: an analogy of biological sciences
To see why this matters, it is useful to think about some important developments in biological sciences. Just as the invention of the microscope scientists enabled to discover cells – the hidden building blocks of life – with these interpretability tools, AI researchers can discover the building blocks of thinking in models. And just as mapping neural circuits in the brain or the sequencing of the genome freeing the way for breakthroughs in medicine, the inner operation of Claude could pave the way for more reliable and controllable machine intelligence. These interpretability tools can play a crucial role and help us peek into the thinking process of AI models.
The challenges
Even with all this progress we are still far from fully understanding of LLMS such as Claude. At the moment, attribution graphs can only explain one in four decisions by Claude. Although the map of his functions is impressive, it only covers part of what is going on in Claude’s Brain. With billions of parameters, Claude and other LLMs perform countless calculations for each task. Tracing everyone to see how an answer is, is like trying to follow any neuron fires in a human brain during a single thought.
There is also the challenge of ‘hallucination’. Sometimes AI models generate reactions that sound plausible, but actually incorrect – such as confidence in an incorrect fact. This happens because the models rely on patterns from their training data instead of a real understanding of the world. Understanding why they float in manufacture remains a difficult problem and emphasizes the gaps in our understanding of their inner operation.
Bias is another important obstacle. AI models learn from enormous data sets that have been scraped from the internet, which wear inherently wear human prejudices – stuffedotypes, prejudices and other social defects. If Claude picks up these prejudices from his training, this can reflect them in his answers. Unpacking where these prejudices originated and how they influence the reasoning of the model is a complex challenge that requires both technical solutions and careful consideration of data and ethics.
The Bottom Line
Anthropic’s work when making large language models (LLMs) such as Claude is more understandable in an important step forward in AI transparency. By revealing how Claude processes information and makes decisions, they forward to tackling important concerns about AI accounting obligation. This progress opens the door for a safe integration of LLMS in critical sectors such as health care and rights, where trust and ethics are vital.
As methods for improving interpretability develop, industries that have been careful with accepting AI can now reconsider. Transparent models such as Claude offer a clear path to the future of AI machines that not only replicate human intelligence, but also explain their reasoning.