How Does Synthetic Data Impact AI Hallucinations?
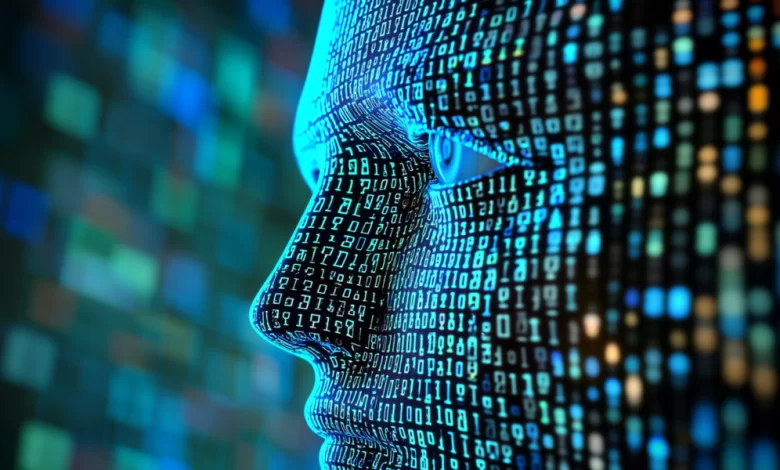
Although synthetic data is a powerful tool, it can only reduce artificial intelligence hallucinations under specific circumstances. In almost any other case it will strengthen them. Why is this? What does this phenomenon mean for those who have invested in it?
How do synthetic data differ from real data?
Synthetic data is information generated by AI. Instead of being collected from real-world events or observations, it is artificially produced. However, it looks like the original to produce an accurate, relevant output. That is the idea anyway.
To make an artificial data set, AI -Governmente train a generative algorithm on a true relational database. When asked, it produces a second set that closely reflects the first, but contains no real information. Although the general trends and mathematical properties remain intact, there is enough noise to mask the original relationships.
A dataset generated by AI goes beyond Deidentification and replaces the underlying logic of relationships between fields instead of simple fields to replace equivalent alternatives. Because it does not contain identifying details, companies can use it to bypass privacy and copyright regulations. What is even more important, they can freely share or distribute it without fear of an infringement.
However, fake information is used more often for supplementation. Companies can use it to enrich or expand sample sizes that are too small, making them large enough to effectively train AI systems.
Minimizes synthetic data AI -Hallucinations?
Sometimes algorithms refer to non -existent events or make logical impossible suggestions. These hallucinations are often absurd, misleading or incorrect. For example, a large language model can write a how-to-article about the interior of Leeuwen or become a doctor at the age of 6. However, they are not that extreme that recognizing them can make a challenge.
If composed correctly, artificial data can reduce these incidents. A relevant, authentic training database is the basis for each model, so it makes sense that the more details someone has, the more accurate the output of their model will be. An additional dataset makes scalability possible, even for niche applications with limited public information.
Debiasing is another way in which a synthetic database can minimize ai -halucinations. According to the MIT Sloan School of Management Can help tackle Bias Because it is not limited to the original sample size. Professionals can use realistic details to enter the gaps in which selected subpopulations are under or over -represented.
How artificial data make hallucinations worse
Since intelligent algorithms Cannot reason or contextualize informationThey are susceptible to hallucinations. Generative models – in particular starter large language models – are particularly vulnerable. In some respects, artificial facts worsen the problem.
Bias -reinforcement
Just like people, AI can learn and reproduce prejudices. If an artificial database overperes some groups, while others under-representative-which are carefully easy to accidentally do the decision-making logic skewed racks, which adversely affects the outcutness.
A similar problem can arise when companies use fake data to eliminate prejudices from practice because it may no longer reflect the reality. For example since More than 99% of breast cancer Prevention in women, the use of additional information to balance the display, can diagnoses skewers.
Intersectional hallucinations
Intersectionality is a sociological framework that describes how demography such as age, gender, race, profession and classes intersect. It analyzes how the overlapping social identities of groups result in unique combinations of discrimination and privileges.
When a generative model is asked to produce artificial details based on what it has trained, it can generate combinations that did not exist in the original or are logically impossible.
Ericka Johnson, a professor of gender and society at Linköping University, collaborated with a Machine Learning scientist to demonstrate this phenomenon. They used a generative opponent network To make synthetic versions from United States Census figures from 1990.
Immediately they saw a striking problem. The artificial version had categories entitled “Wife and Single” and “Never Married Husbers”, who were both intersectional hallucinations.
Without the correct curation, the replica database will always over -representatives in dates sets in data sets, while under -represented – or even exclusion – under -represented groups. Edge cases and from bijters can be completely ignored in favor of dominant trends.
Model
An exaggerated dependence on artificial patterns and trends leads to the collapse of the model-from which the performance of an algorithm drastically deteriorates as it becomes less adaptable to observations and events in practice.
This phenomenon is particularly clear in generative AI of the next generation. Repeated use of an artificial version to train them results in a self -examination. One study showed that their Quality and recovery Gradually without sufficient recent, actual figures in every generation.
Overfect
Overfitting is an exceedance of training data. The algorithm initially performs well, but will hallucinate when they present new data points. Synthetic information can aggravate this problem if it does not accurately reflect reality.
The implications of continuous synthetic data use
The market for synthetic data is booming. Companies in this niche industry about $ 328 million picked up In 2022, an increase of $ 53 million in 2020 – an increase of 518% in just 18 months. It is worth noting that this is exclusively publicly known financing, which means that the actual figure can be even higher. It is safe to say that companies are invested incredibly in this solution.
If companies continue to use an artificial database without the right curation and debasing, the performance of their model will gradually decrease, which means that their AI investments are sent. The results can be more serious depending on the application. In health care, for example, an increase in hallucinations can lead to incorrect diagnoses or incorrect treatment plans, which leads to poorer patient results.
The solution does not include returning to real data
AI systems require millions, if not billions, images, text and videos for training, a large part of public websites are scraped and compiled in massive, open data sets. Unfortunately, algorithms use this information faster than people can generate it. What happens when they learn everything?
Managers are concerned about touching the data wall – the point at which all public information is exhausted on the internet. It can approach faster than they think.
Although both the amount of flat text on the average common crawl -webpage and the number of internet users grow by 2% to 4% Every year, algorithms no longer have high -quality data. Only 10% to 40% can be used for training without jeopardizing performance. If the trends continue, the government information generated by people could be able to be on by 2026.
In all likelihood, the AI sector can hit the data wall earlier. The generative AI tree of recent years has increased tensions about information possession and copyright infringement. More website owners use Robots Exclusion Protocol-a standard that uses a robots.txt file to block webcrawlers or make it clear that their site is prohibited.
A study from 2024 published by a research group led by MIT revealed that the data set of the colossal Cleaned Common Crawl (C4)-a large-scale webcrawlcorpus restrictions are rising in the elevator. Over 28% of the most active, critical sources In C4 were completely limited. Moreover, 45% of the C4 is now prohibited by the service conditions.
If companies respect these restrictions, the freshness, relevance and accuracy of public facts will decrease from practice, forcing them to rely on artificial databases. They may not have much choice if the courts rule that an alternative is a copyright infringement.
The future of synthetic data and AI -Hallucinations
As copyright laws modernize and more website owners hide their content for webcrawlers, the generation of artificial data set becomes increasingly popular. Organizations must prepare for the threat of hallucinations.